Hybrid Dictionary Learning and Matching for Video-based Face Verification
2019 IEEE 10th International Conference on Biometrics Theory, Applications and Systems (BTAS)(2019)
摘要
We propose a hybrid dictionary learning and matching approach using deep features for unconstrained videobased face verification. Popular off-the-shelf image-based deep neural networks often fail to effectively exploit multiple frames for video-based verification. Unlike recurrent neural network-based approaches which require an external large-scale annotated data for training, the proposed unsupervised approach can effectively model both structural and temporal information of face features in target videos using structural and dynamical dictionaries, respectively. We propose an iterative optimization procedure to learn the dynamical dictionaries from videos. Using the learned dictionaries, we model video-to-video similarity as subspace-to-subspace similarity which is not only more robust but also utilizes the information in multiple frames better than the widely used reconstruction error-based measures, where the subspaces are spanned by the learned dictionaries. Experiments on challenging video-based face verification datasets, including Multiple Biometric Grand Challenge (MBGC), Face and Ocular Challenge Series (FOCS) and IARPA JANUS Benchmark A (IJB-A) datasets, demonstrate that the proposed method can effectively learn robust and discriminative representations for videos and improve the face verification performance.
更多查看译文
关键词
learned dictionaries,model video-to-video similarity,subspace-to-subspace similarity,widely used reconstruction error-based measures,challenging video-based face verification datasets,face verification performance,hybrid dictionary learning,matching approach,deep features,unconstrained videobased face verification,popular off-the-shelf image-based deep neural networks,video-based verification,recurrent neural network-based approaches,large-scale annotated data,unsupervised approach,structural information,temporal information,face features,target videos,dynamical dictionaries
AI 理解论文
溯源树
样例
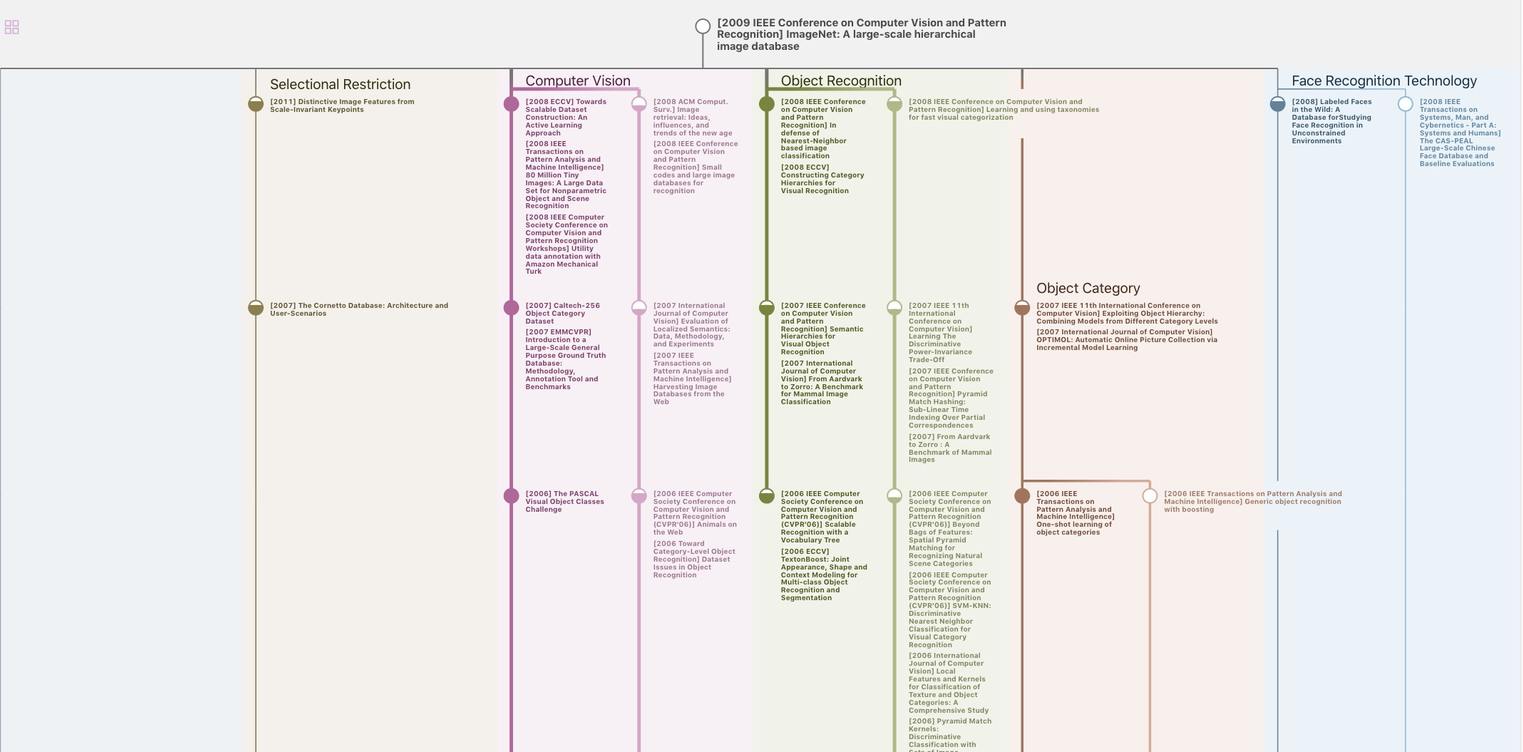
生成溯源树,研究论文发展脉络
Chat Paper
正在生成论文摘要