Towards Noise Resilient SLAM
2020 IEEE International Conference on Robotics and Automation (ICRA)(2020)
摘要
Sparse-indirect SLAM systems have been dominantly popular due to their computational efficiency and photometric invariance properties. Depth sensors are critical to SLAM frameworks for providing scale information to the 3D world, yet known to be plagued by a wide variety of noise sources, possessing lateral and axial components. In this work, we demonstrate the detrimental impact of these depth noise components on the performance of the state-of-the-art sparse-indirect SLAM system (ORB-SLAM2). We propose (i) Map-Point Consensus based Outlier Rejection (MC-OR) to counter lateral noise, and (ii) Adaptive Virtual Camera (AVC) to combat axial noise accurately. MC-OR utilizes consensus information between multiple sightings of the same landmark to disambiguate noisy depth and filter it out before pose optimization. In AVC, we introduce an error vector as an accurate representation of the axial depth error. We additionally propose an adaptive algorithm to find the virtual camera location for projecting the error used in the objective function of the pose optimization. Our techniques work equally well for stereo image pairs and RGB-D input directly used by sparse-indirect SLAM systems. Our methods were tested on the TUM (RGB-D) and EuRoC (stereo) datasets and we show that they outperform existing state-of-the-art ORB-SLAM2 by 2-3x, especially in sequences critically affected by depth noise.
更多查看译文
关键词
RGB-D input,TUM datasets,EuRoC datasets,stereo image pairs,adaptive algorithm,error vector,outlier rejection,computational efficiency,map-point consensus,adaptive virtual camera,noise resilient SLAM,ORB-SLAM2,sparse-indirect SLAM systems,virtual camera location,axial depth error,pose optimization,consensus information,axial noise,lateral noise,depth noise components,axial components,lateral components,noise sources,scale information,SLAM frameworks,depth sensors,photometric invariance properties
AI 理解论文
溯源树
样例
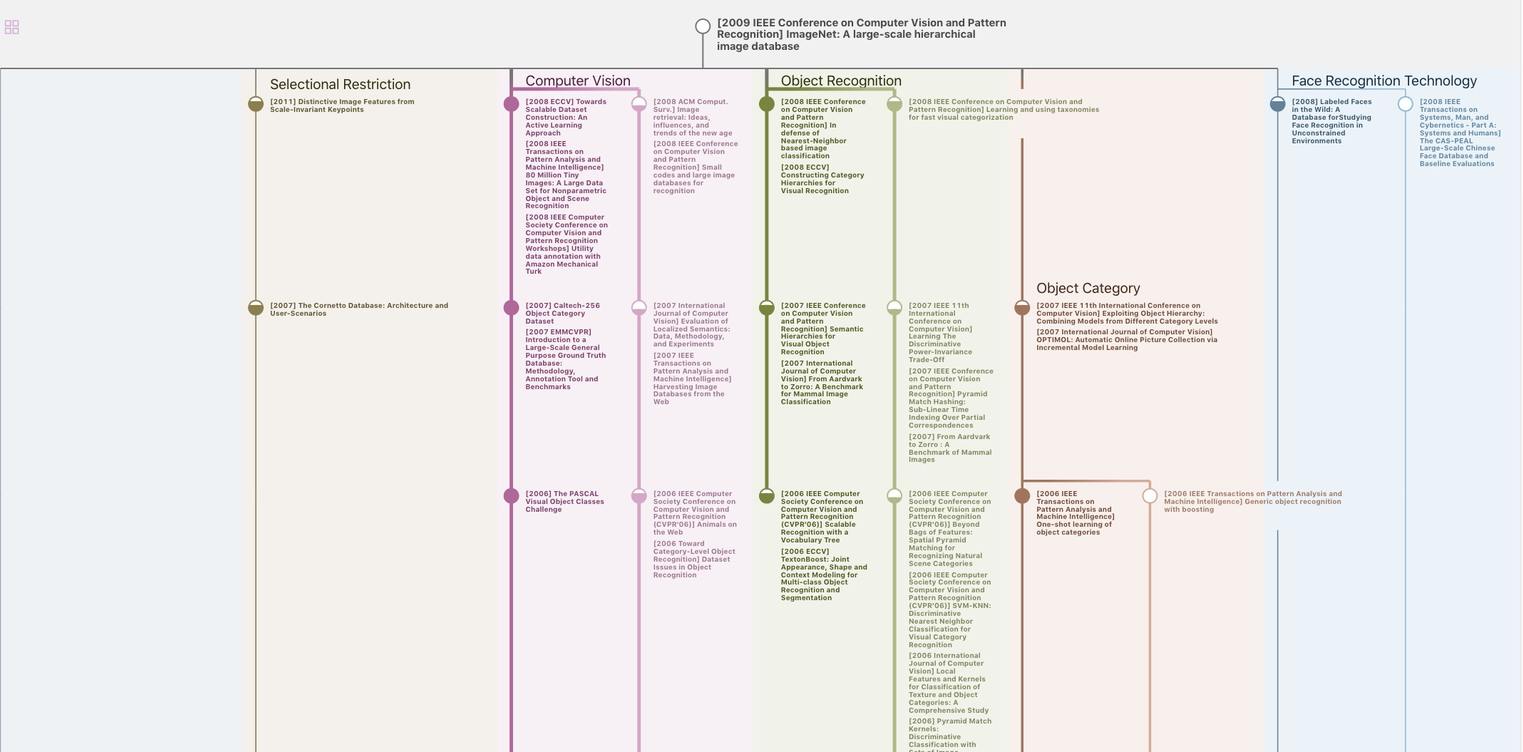
生成溯源树,研究论文发展脉络
Chat Paper
正在生成论文摘要