Accurate detection and 3D localization of humans using a novel YOLO-based RGB-D fusion approach and synthetic training data.
ICRA(2020)
摘要
While 2D object detection has made significant progress, robustly localizing objects in 3D space under presence of occlusion is still an unresolved issue. Our focus in this work is on real-time detection of human 3D centroids in RGB-D data. We propose an image-based detection approach which extends the YOLO v3 architecture with a 3D centroid loss and mid-level feature fusion to exploit complementary information from both modalities. We employ a transfer learning scheme which can benefit from existing large-scale 2D object detection datasets, while at the same time learning end-to-end 3D localization from our highly randomized, diverse synthetic RGB-D dataset with precise 3D groundtruth. We further propose a geometrically more accurate depth-aware crop augmentation for training on RGB-D data, which helps to improve 3D localization accuracy. In experiments on our challenging intralogistics dataset, we achieve state-of-the-art performance even when learning 3D localization just from synthetic data.
更多查看译文
关键词
synthetic training data,real-time detection,human 3D centroids,RGB-D data,image-based detection approach,YOLO v3 architecture,3D centroid loss,mid-level feature fusion,transfer learning scheme,large-scale 2D object detection datasets,end-to-end 3D localization,precise 3D groundtruth,3D localization accuracy,learning 3D localization,YOLO-based RGB-D fusion approach,depth-aware crop augmentation,intralogistics dataset
AI 理解论文
溯源树
样例
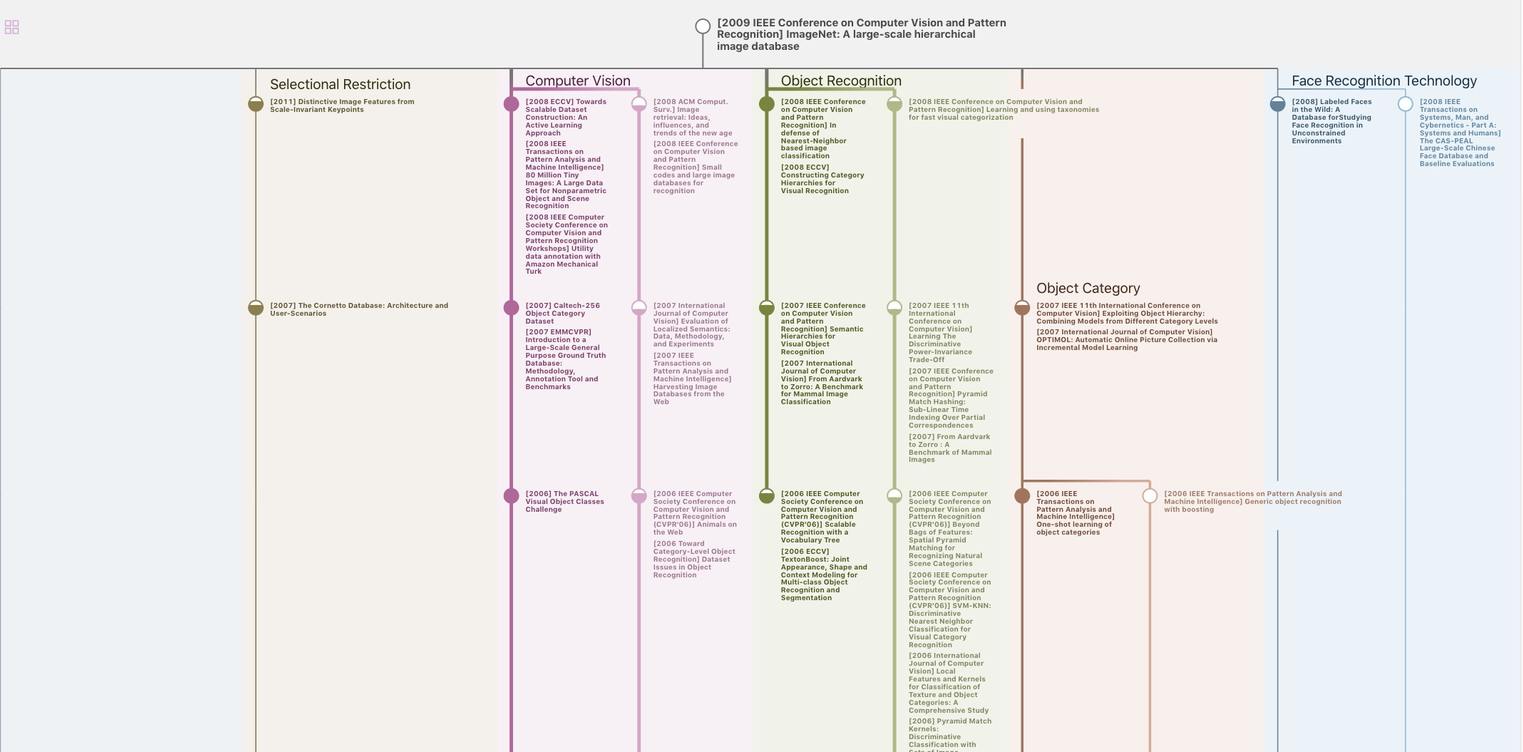
生成溯源树,研究论文发展脉络
Chat Paper
正在生成论文摘要