Dcgan for the Synthesis of Multivariate Multifractal Textures: How do We Know it Works?
2020 IEEE 30th International Workshop on Machine Learning for Signal Processing (MLSP)(2020)
摘要
Deep Learning is nowadays widely used for several tasks in image processing. Notably, it has been massively used for image synthesis, mostly however with strong geometrical contents. Focused on a race for better performance via more complex architectures, research on Deep Learning, however, left behind the critical issue of assessing quantitatively and in a reproducible manner the quality of the synthesized images, notably for the case of pure textures. The present work aims to study the ability of Deep Convolutional Generative Adversarial Networks to synthesize multivariate textures characterized by rich multiscale multivariate statistics (multifractals). The focus is thus on quantifying the quality of the synthesized textures, on assessing the reproducibility of the learning procedure and on studying the impact of loss functions and of training dataset sizes, rather than on proposing yet another architecture.
更多查看译文
关键词
Generative Adversarial Network,Multivariate Texture Synthesis,Quality Assessment,Multifractals
AI 理解论文
溯源树
样例
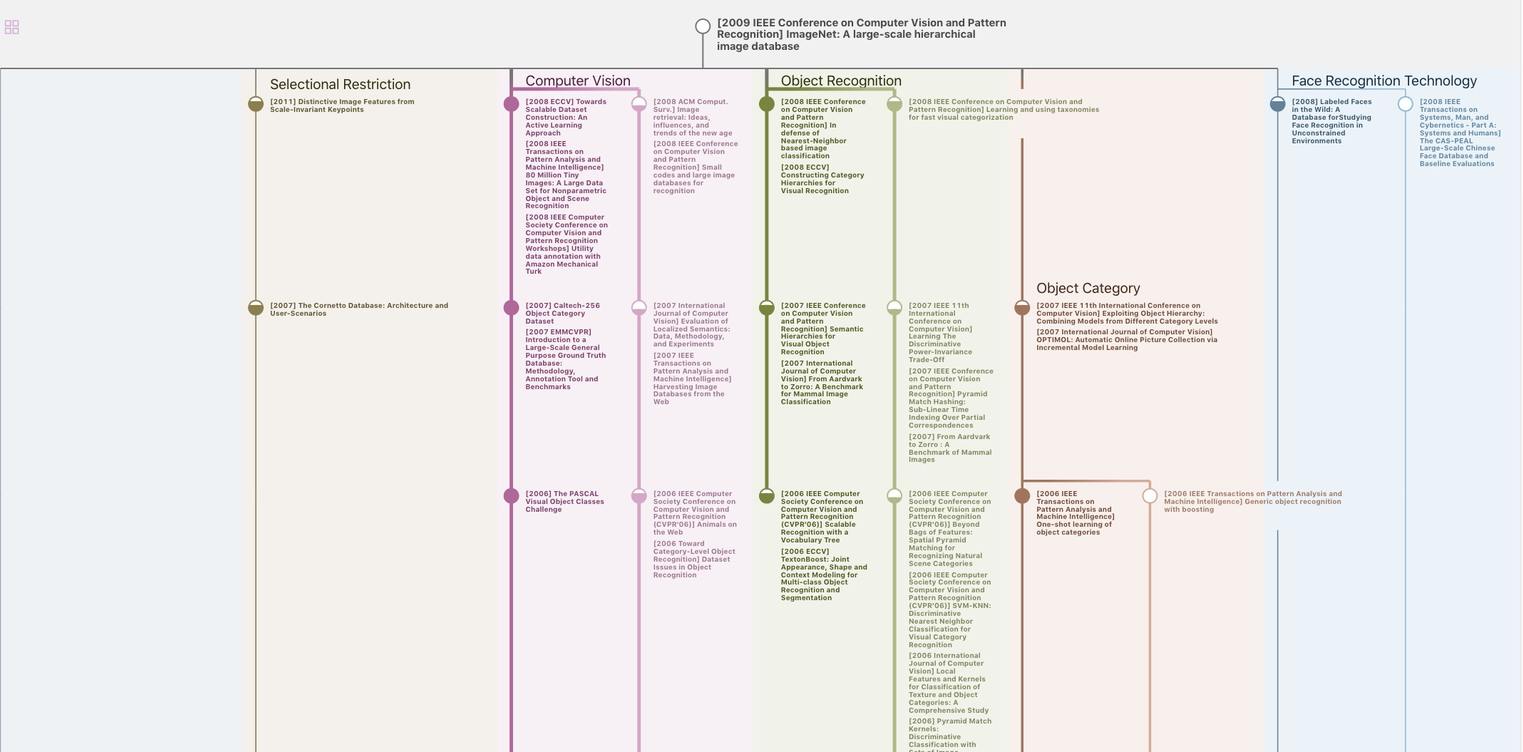
生成溯源树,研究论文发展脉络
Chat Paper
正在生成论文摘要