Equity in Learning Problems - An OWA Approach.
SUM(2020)
摘要
It is well-known in computational social choice that the weighted average does not guarantee any equity or fairness in the share of goods. In a supervised learning problem, this translates into the fact that the empirical risk will lead to models that are good in average, but may have terrible performances for under-represented populations. Such a behaviour is quite damaging in some problems, such as the ones involving imbalanced data sets, in the inputs or the outputs (default prediction, ethical issues,. . .). On the other hand, the OWA operator is known in computational social choice to be able to correct this unfairness. This paper proposes a means to transpose this feature to the supervised learning setting.
更多查看译文
关键词
learning problems,equity
AI 理解论文
溯源树
样例
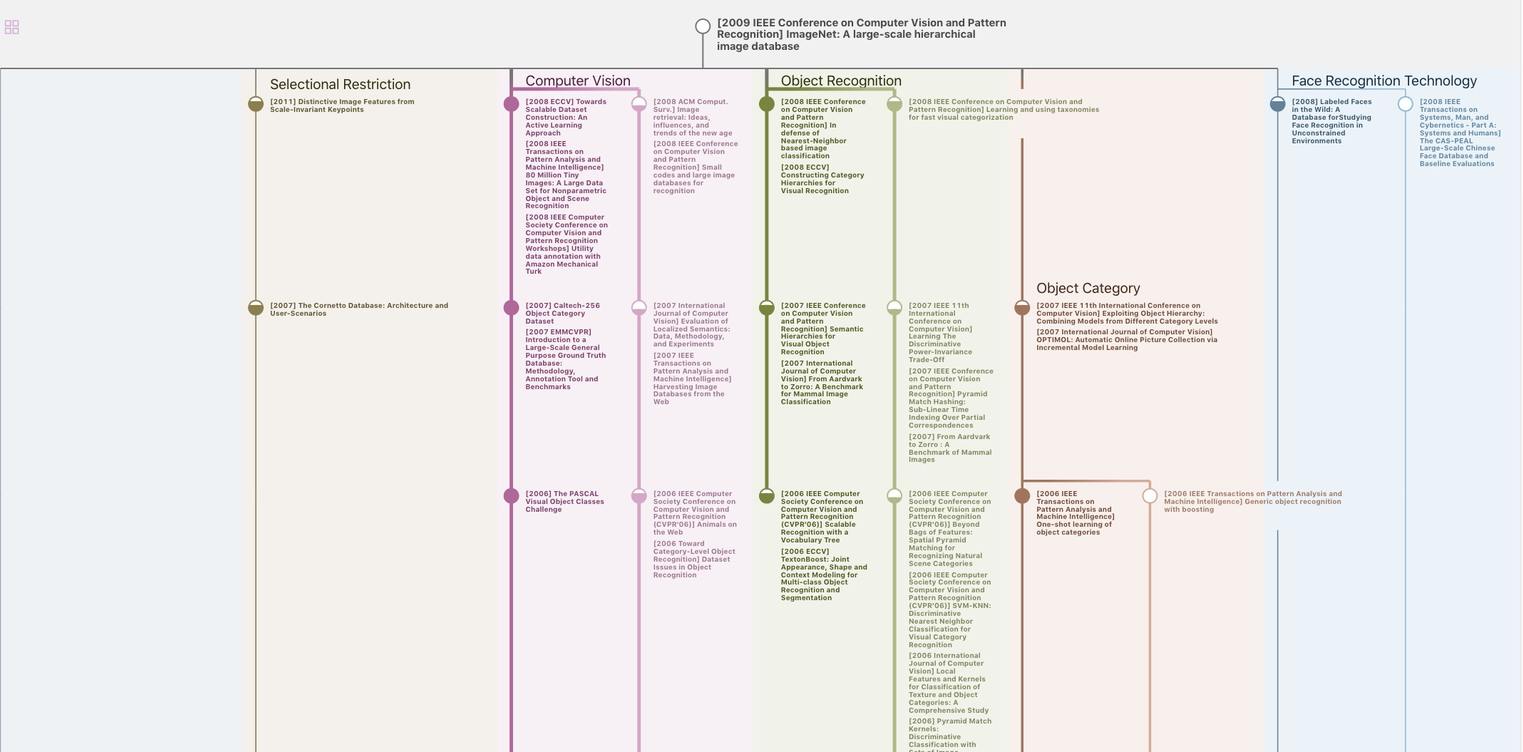
生成溯源树,研究论文发展脉络
Chat Paper
正在生成论文摘要