Accurately Quantifying a Billion Instances per Second
2020 IEEE 7th International Conference on Data Science and Advanced Analytics (DSAA)(2020)
摘要
Quantification is a thriving research area that develops methods to estimate the class prior probabilities in an unlabelled set of observations. Quantification and classification share several similarities. For instance, the most straightforward quantification method, Classify & Count (CC), directly counts the output of a classifier. However, CC has a systematic bias that makes it increasingly misestimate the counts as the class distribution drifts away from a distribution it perfectly quantifies. This issue has motivated the development of more reliable quantification methods. Such newer methods can consistently outperform CC at the cost of a significant increase in processing requirements. Yet, for a large number of applications, quantification speed is an additional criterion that must be considered. Frequently, quantification methods need to deal with large amounts of data or fast-paced streams, as it is the case of news feeding, tweets and sensor data. In this paper, we propose Sample Mean Matching (SMM), a highly efficient algorithm able to quantify billions of data instances per second. We compare SMM to a set of 14 established and state-of-the-art quantifiers in an empirical analysis comprising 25 benchmark and real-world datasets. We show that SMM is competitive with state-of-the-art methods with no statistical difference in counting accuracy, and it is orders of magnitude faster than the vast majority of the algorithms.
更多查看译文
关键词
Machine Learning,Quantification,Mixture Methods
AI 理解论文
溯源树
样例
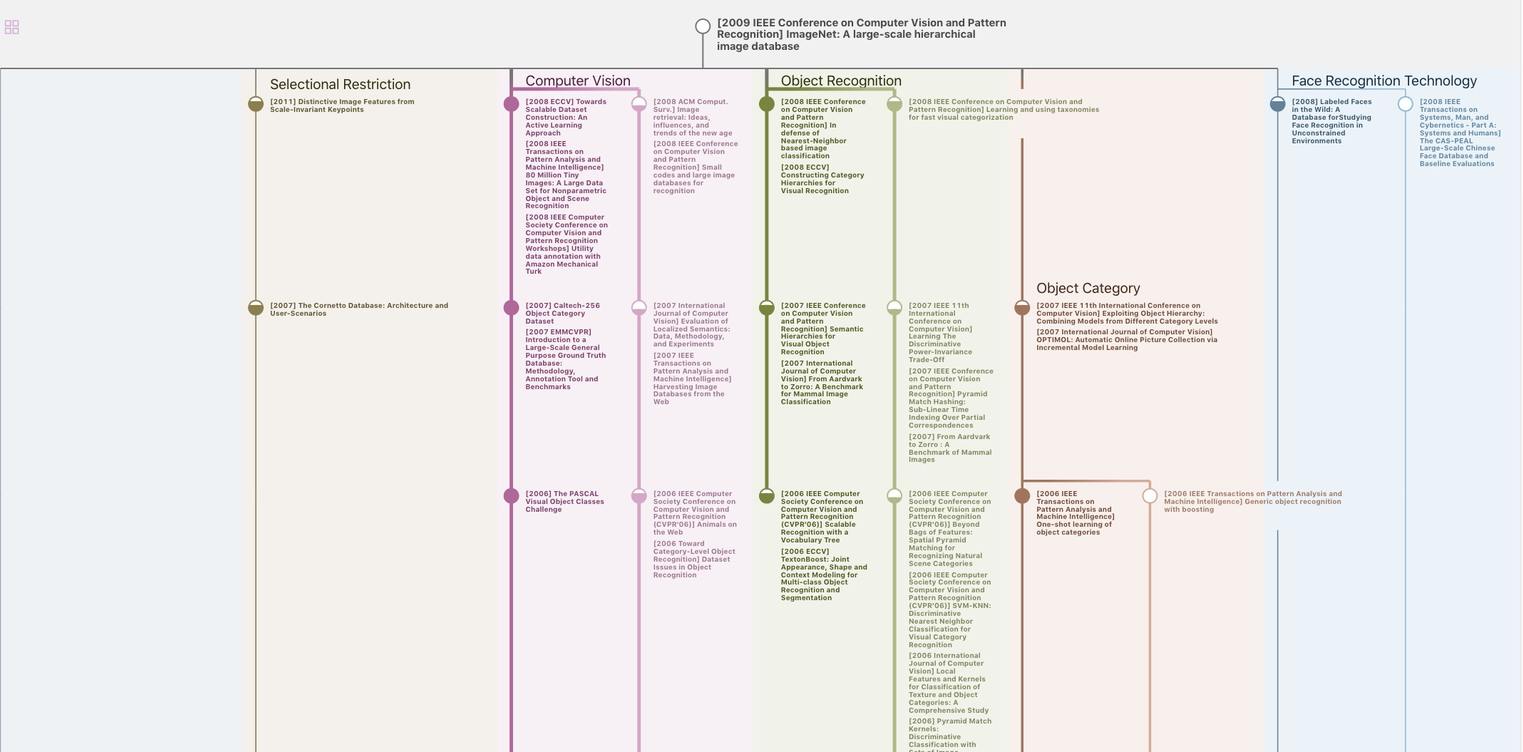
生成溯源树,研究论文发展脉络
Chat Paper
正在生成论文摘要