Hierarchical Grammar-Guided Genetic Programming Techniques for Scheduling in Heterogeneous Networks.
CEC(2020)
摘要
Grammar-Guided Genetic Programming is already outperforming humans at creating efficient transmission schedulers for large heterogeneous communications networks. We have previously proposed a multi-level grammar approach which achieved significantly better results than the canonical Grammar-Guided Genetic Programming approach. Initially, a restricted ‘small’ grammar is utilised in order to discover suitable structures. A full grammar is then adopted after this initial phase. Hence, evolution can focus on maximising performance, by fine-tuning the well-structured models. In this work, we propose to use a hierarchical approach by employing multiple small grammars instead of a unique small grammar at the lower level, in conjunction with the full grammar at the upper level. To use multiple small grammars while maintaining the same computational budget, we have to use either (i) reduce the number of generations, or (ii) reduce the size of the population for the evolution with each of the small grammars. In this work, we confirm that the hierarchical grammar approach using the division of number of generations strategy achieves significantly better results than the multi-level approach, but requires defining an ideal number of small grammars to achieve the best performance. We also show that the hierarchical grammar approach using the division of population size strategy achieves significantly better results than the multi-level approach. However the division of population size strategy is less sensitive to the number of small grammars.
更多查看译文
关键词
Genetic Programming, Telecommunications, Hierarchical Grammar-Guided Genetic Programming, Heterogeneous Network
AI 理解论文
溯源树
样例
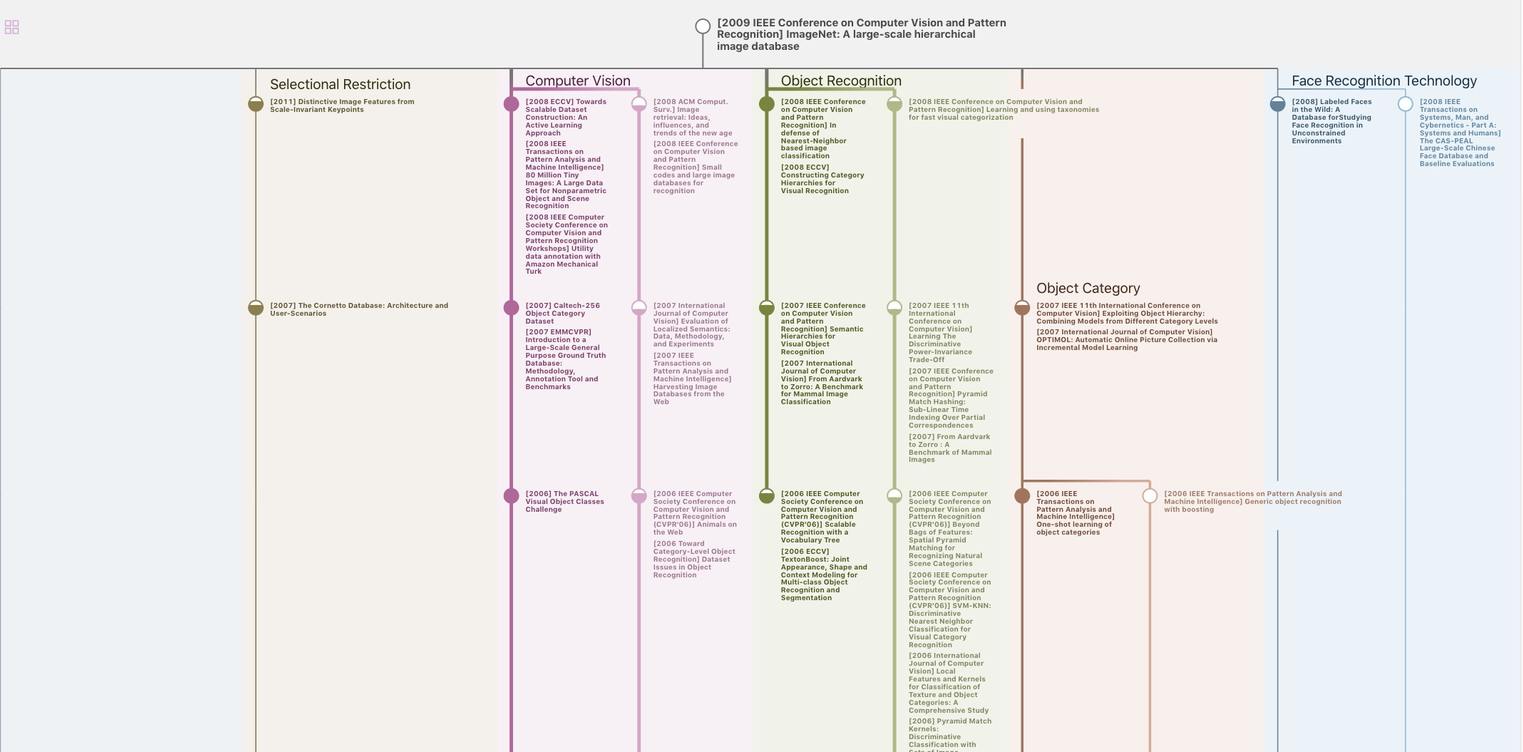
生成溯源树,研究论文发展脉络
Chat Paper
正在生成论文摘要