MaskAAE - Latent space optimization for Adversarial Auto-Encoders.
UAI(2020)
摘要
The field of neural generative models is dominated by the highly successful Generative Adversarial Networks (GANs) despite their challenges, such as training instability and mode collapse. Auto-Encoders (AE) with regularized latent space provide an alternative framework for generative models, albeit their performance levels have not reached that of GANs. In this work, we hypothesise that the dimensionality of the AE model's latent space has a critical effect on the quality of generated data. Under the assumption that nature generates data by sampling from a "true" generative latent space followed by a deterministic function, we show that the optimal performance is obtained when the dimensionality of the latent space of the AE-model matches with that of the "true" generative latent space. Further, we propose an algorithm called the Mask Adversarial Auto-Encoder (MaskAAE), in which the dimensionality of the latent space of an adversarial auto encoder is brought closer to that of the "true" generative latent space, via a procedure to mask the spurious latent dimensions. We demonstrate through experiments on synthetic and several real-world datasets that the proposed formulation yields betterment in the generation quality.
更多查看译文
AI 理解论文
溯源树
样例
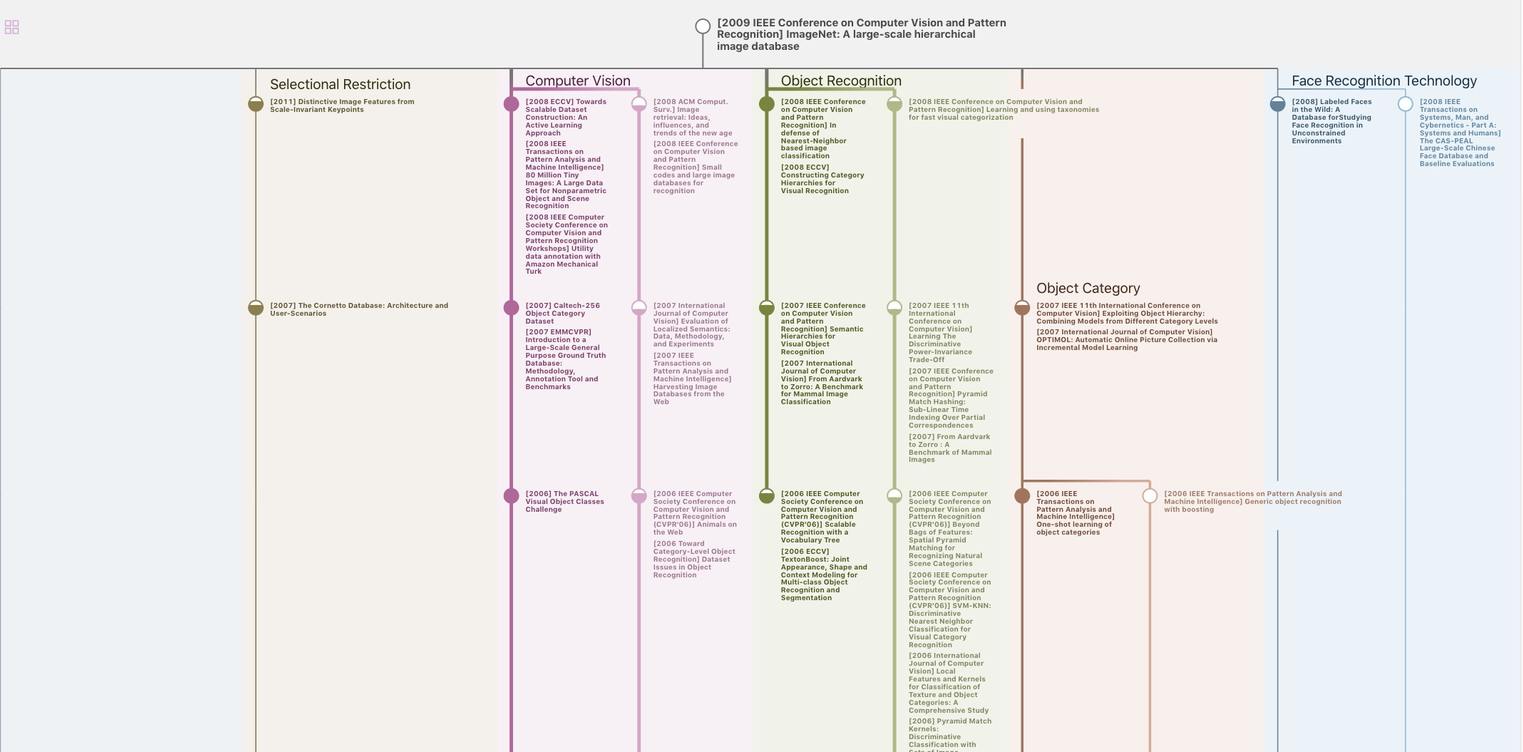
生成溯源树,研究论文发展脉络
Chat Paper
正在生成论文摘要