A Pipelined Approach to Deal with Image Distortion in Computer Vision.
BRACIS(2020)
摘要
Image classification is a well-established problem in computer vision. Most state-of-the-art models rely on Convolutional Neural Networks to achieve near-human performance in that task. However, CNNs have shown to be susceptible to image manipulation, which undermines the trustability of perception systems. This property is critical, especially in unmanned systems, autonomous vehicles, and scenarios where light cannot be controlled. We investigate the robustness of several Deep-Learning based image recognition models and how the accuracy is affected by several distinct image distortions. The distortions include ill-exposure, low-range image sensors, and common noise types. Furthermore, we also propose and evaluate an image pipeline designed to minimize image distortion before the image classification is performed. Results show that most CNN models are marginally affected by mild miss-exposure and Shot noise. On the one hand, the proposed pipeline can provide significant gain on miss-exposed images. On the other hand, harsh miss-exposure, signal-dependent noise, and impulse noise, incur in a high impact on all evaluated models.
更多查看译文
关键词
image distortion,computer vision,pipelined approach
AI 理解论文
溯源树
样例
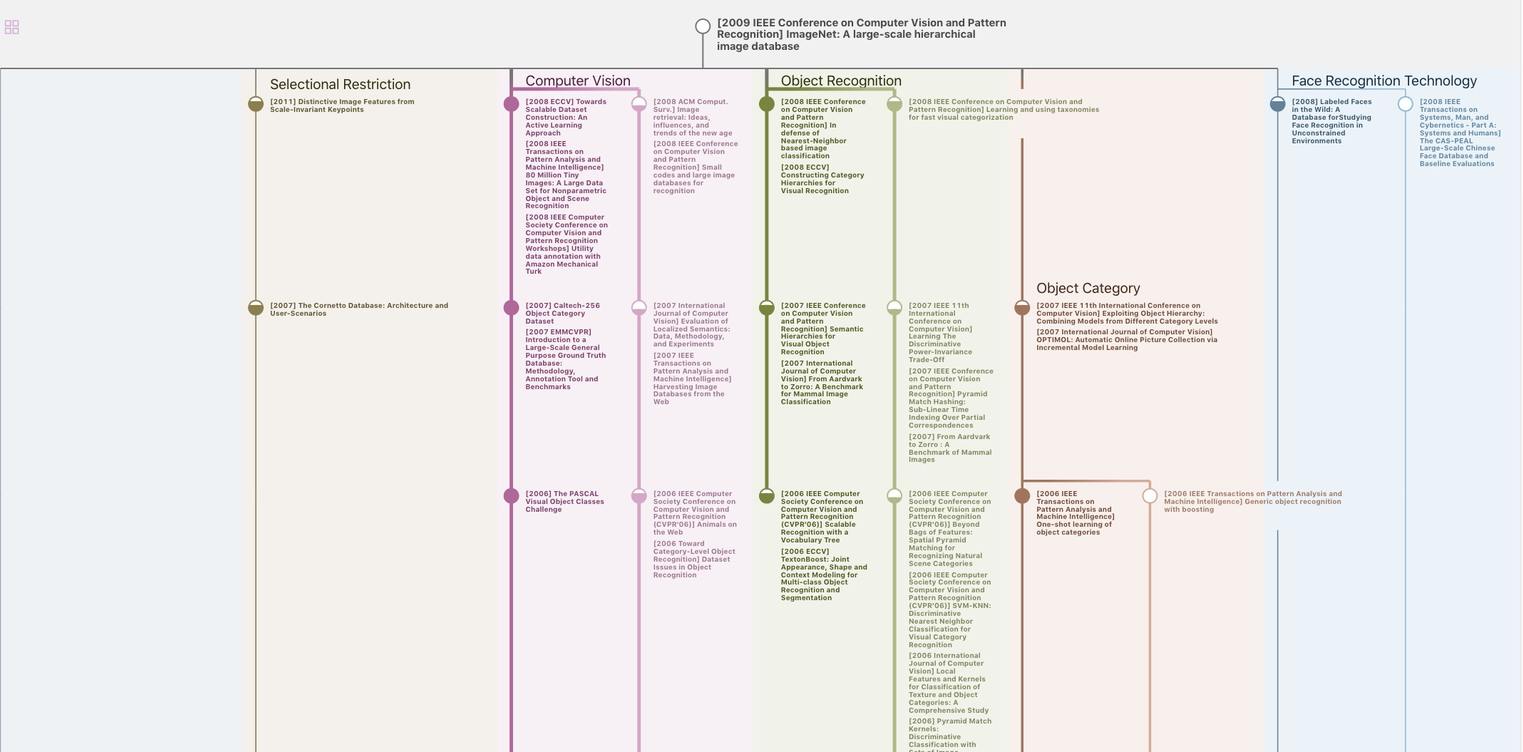
生成溯源树,研究论文发展脉络
Chat Paper
正在生成论文摘要