Improving Speech Recognition of Compound-Rich Languages.
INTERSPEECH(2020)
摘要
Traditional hybrid speech recognition systems use a fixed vocabulary for recognition, which is a challenge for agglutinative and compounding languages due to the presence of large number of rare words. This causes high out-of-vocabulary rate and leads to poor probability estimates for rare words. It is also important to keep the vocabulary size in check for a low-latency WFST-based speech recognition system. Previous works have addressed this problem by utilizing subword units in the language model training and merging them back to reconstruct words in the post-processing step. In this paper, we extend such open vocabulary approaches by focusing on compounding aspect. We present a data-driven unsupervised method to identify compound words in the vocabulary and learn rules to segment them. We show that compound modeling can achieve 3% to 8% relative reduction in word error rate and up to 9% reduction in the vocabulary size compared to word-based models. We also show the importance of consistency between the lexicon employed during decoding and acoustic model training for subword-based systems.
更多查看译文
关键词
Speech Recognition, Subword Modeling, Compounding, German
AI 理解论文
溯源树
样例
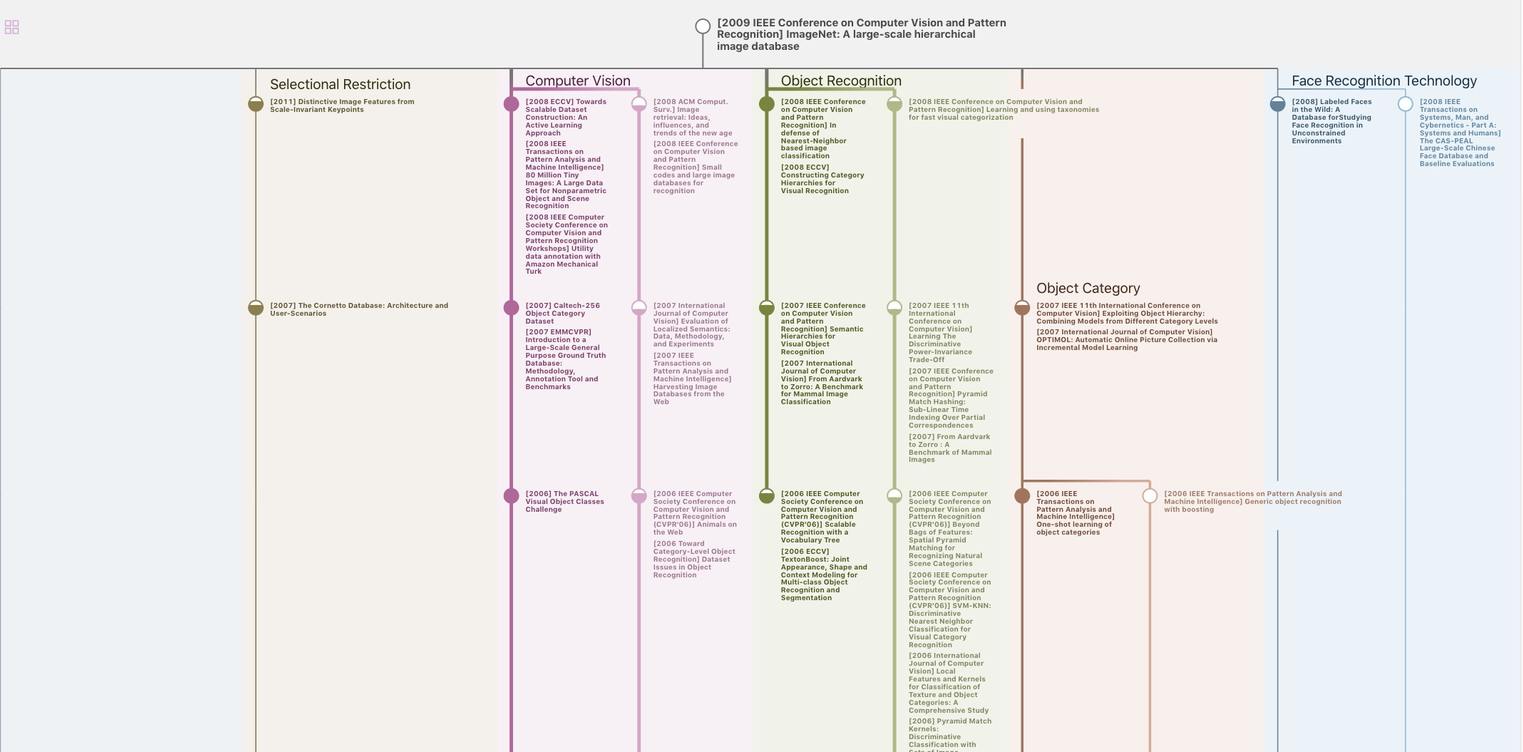
生成溯源树,研究论文发展脉络
Chat Paper
正在生成论文摘要