Anti-Aliasing Regularization in Stacking Layers.
INTERSPEECH(2020)
摘要
Shift-invariance is a desirable property of many machine learning models. It means that delaying the input of a model in time should only result in delaying its prediction in time. A model that is shift-invariant, also eliminates undesirable side effects like frequency aliasing. When building sequence models, not only should the shift-invariance property be preserved when sampling input features, it must also be respected inside the model itself. Here, we study the impact of the commonly used stacking layer in LSTM-based ASR models and show that aliasing is likely to occur. Experimentally, by adding merely 7 parameters to an existing speech recognition model that has 120 million parameters, we are able to reduce the impact of aliasing. This acts as a regularizer that discards frequencies the model shouldn't be relying on for predictions. Our results show that under conditions unseen at training, we are able to reduce the relative word error rate by up to 5%.
更多查看译文
关键词
aliasing, sampling theorem, stacking layers, regularization, speech recognition
AI 理解论文
溯源树
样例
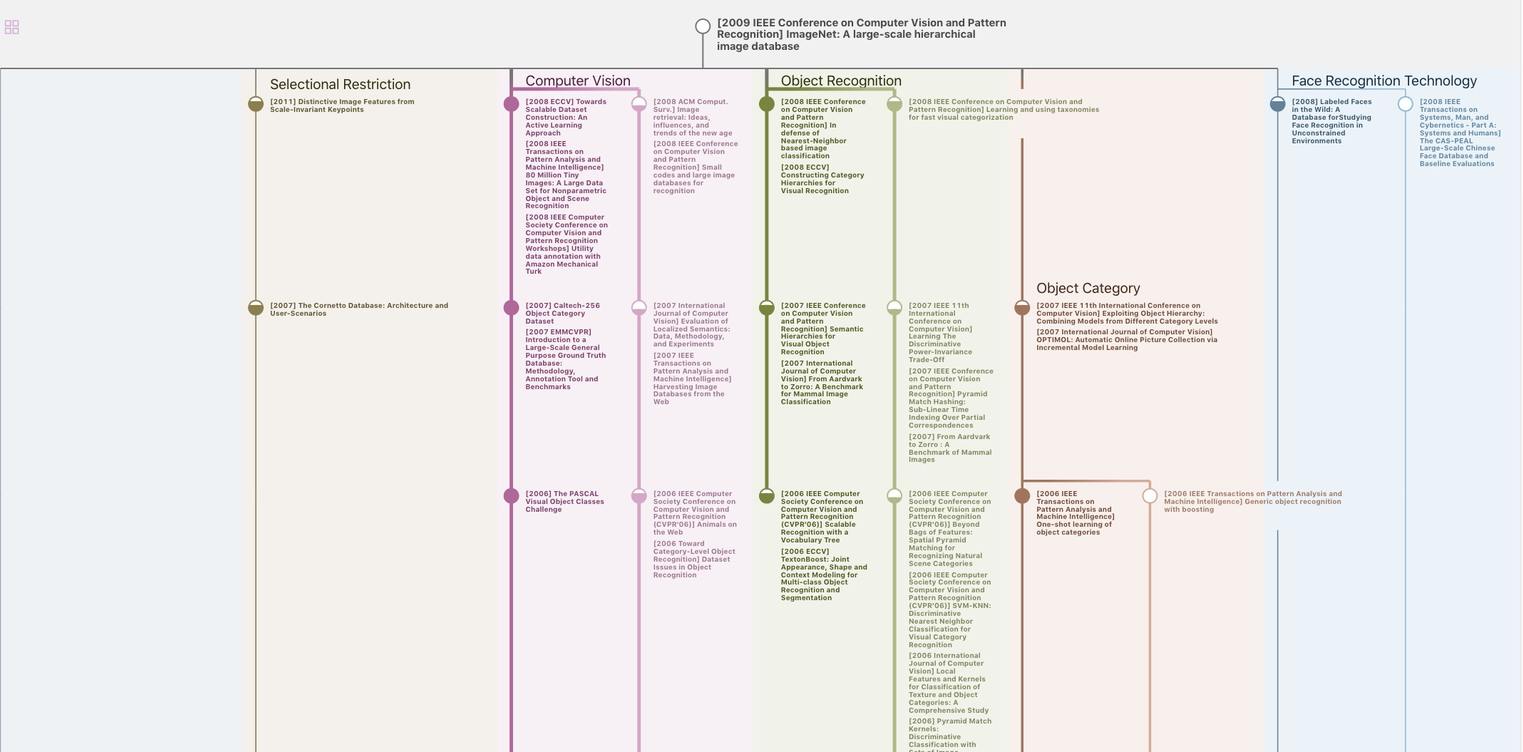
生成溯源树,研究论文发展脉络
Chat Paper
正在生成论文摘要