Efficient Privacy-Preserving Approaches for Trajectory Datasets.
DASC/PiCom/CBDCom/CyberSciTech(2020)
摘要
The use of credit cards or RFID cards for transaction payment has become ubiquitous in recent years. This transactional data is stored in the form of a trajectory, including a sequence of locations at which the customer used the credit card. Such datasets are sensitive since they store customer's locations and purchasing patterns. Releasing this type of dataset without proper anonymization may breach individual's privacy. Moreover, an adversary can attack the dataset with partial trajectory knowledge to reveal sensitive information about a person. We study trajectory data anonymization techniques for this problem, where a data publisher can construct a safe dataset with minimum information loss. A global suppression algorithm was proposed in [1] for trajectory data anonymization. The algorithm is computationally expensive for large trajectory datasets. We propose a tree-based data structure that significantly reduces the computational cost of the global suppression algorithm. In an experiment with a real-world dataset, our data structure performs global suppression in 50% less time than the stateof-the-art algorithm.
更多查看译文
关键词
anonymizaton, trajectory, generalization, suppression, projection, tree
AI 理解论文
溯源树
样例
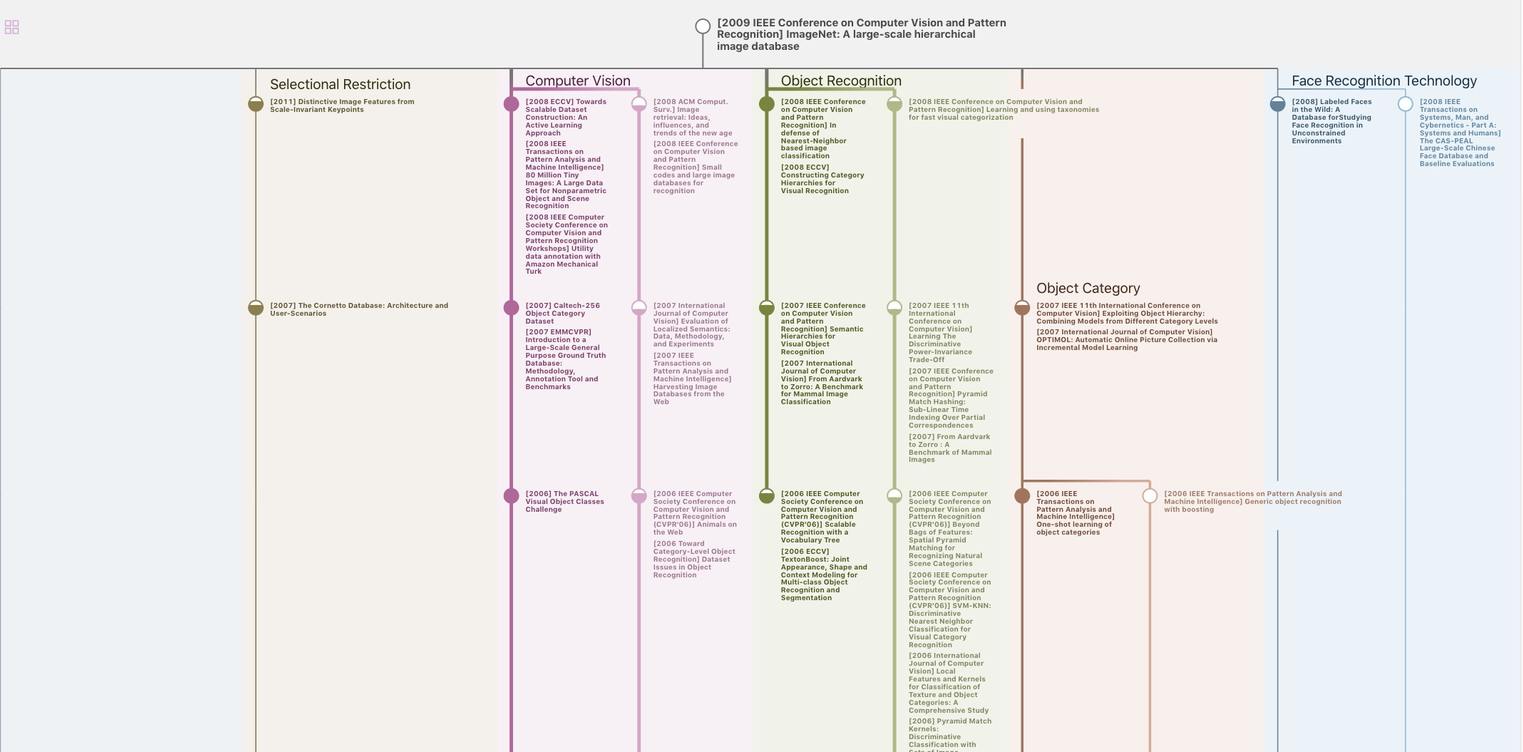
生成溯源树,研究论文发展脉络
Chat Paper
正在生成论文摘要