Benchmarking reinforcement learning algorithms for demand response applications
2020 IEEE PES Innovative Smart Grid Technologies Europe (ISGT-Europe)(2020)
摘要
Through many recent successes in simulation and real-world projects, reinforcement learning (RL) has emerged as a promising approach for demand response applications especially in the residential setting. Reinforcement learning is a self-learning and self-adaptive technique that can be used to control flexibility providing devices by relying mainly on historical and/or real-time data rather than on system models. This paper presents a benchmark of five RL algorithms - fitted Q-iteration, policy iteration with Q-functions, double Q-learning, REINFORCE and actor-critic - and compares these with a model-based optimal, rule-based and naive control. We consider a task of controlling the operation of a heat pump (HP) for space heating in a building with a photovoltaic (PV) installation. The HP is controlled with goal of maximizing PV self-consumption and consequently, minimizing electricity cost. To evaluate the performance of these algorithms, three main indicators are considered: PV self-consumption, electricity cost and computation time. Based on simulation results in which the same number of training samples are considered, fitted Q-iteration outperforms the other RL algorithms, the naive and rule-based controls in terms of PV self-consumption and net electricity cost. However, a 7.6% decrease in PV self-consumption and 77% increase in net electricity cost is observed compared to the optimal control.
更多查看译文
关键词
benchmarking,control,demand response,reinforcement learning
AI 理解论文
溯源树
样例
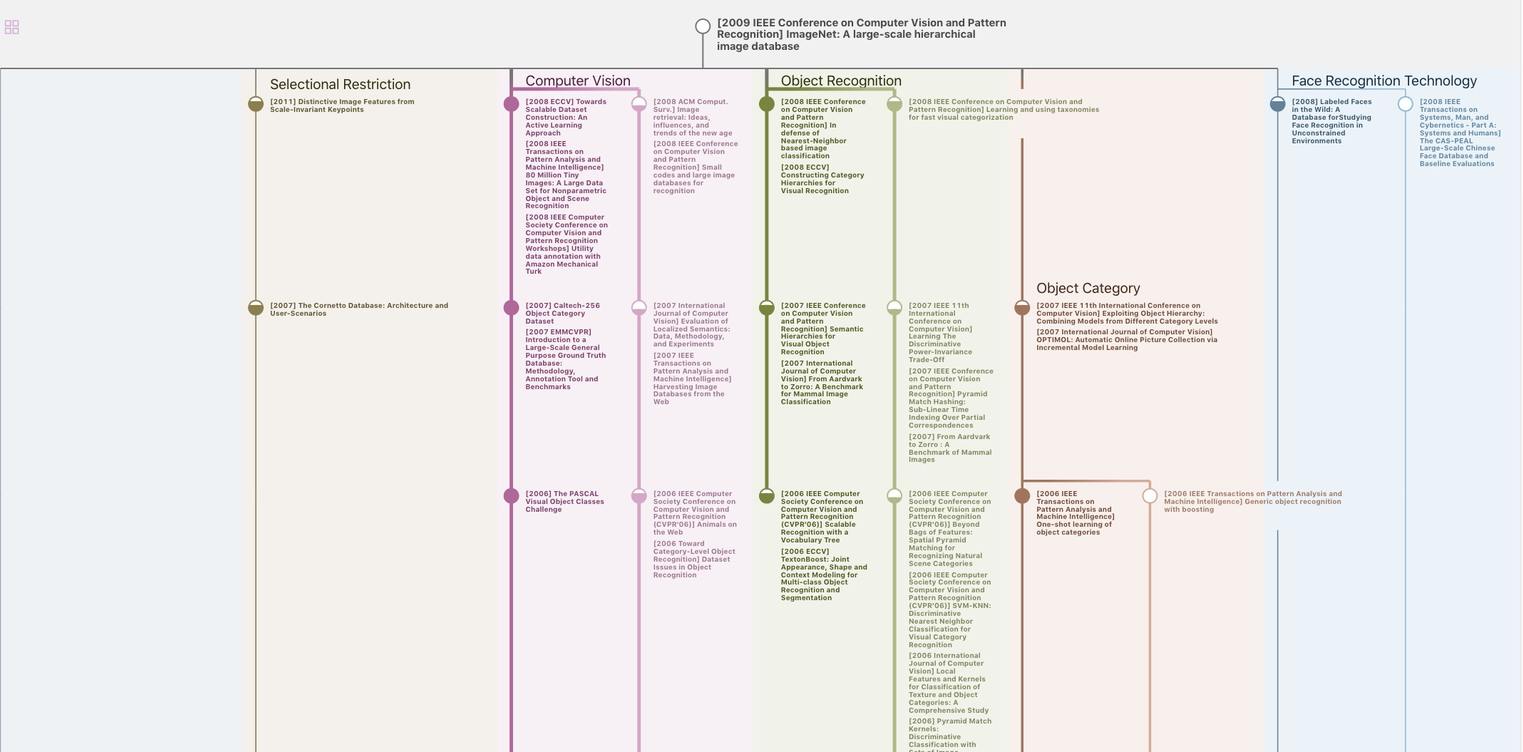
生成溯源树,研究论文发展脉络
Chat Paper
正在生成论文摘要