Adaptive rolling window selection for minimum variance portfolio estimation based on reinforcement learning.
MIPRO(2020)
摘要
When allocating wealth to a set of financial assets, portfolio optimization techniques are used to select optimal portfolio allocations for given investment goals. Among benchmark portfolios commonly used in modern portfolio theory, the global minimum variance portfolio is becoming increasingly popular with investors due to its relatively good performance which stems from both the low-volatility anomaly and the avoidance of the estimation of first moments i.e. mean returns. However, estimates of minimum variance portfolio weights significantly depend on the size of the rolling window used for estimation, especially considering the non-stationarity of the underlying market dynamics. In this paper, we use a model-free policy-based reinforcement learning framework in order to directly and adaptively determine the optimal size of the rolling window. Training is done on a subset of trading stocks from the NYSE. The resulting agent achieves superior performance when compared against multiple benchmarks, including those with fixed rolling window sizes.
更多查看译文
关键词
reinforcement learning, portfolio optimization, covariance estimation
AI 理解论文
溯源树
样例
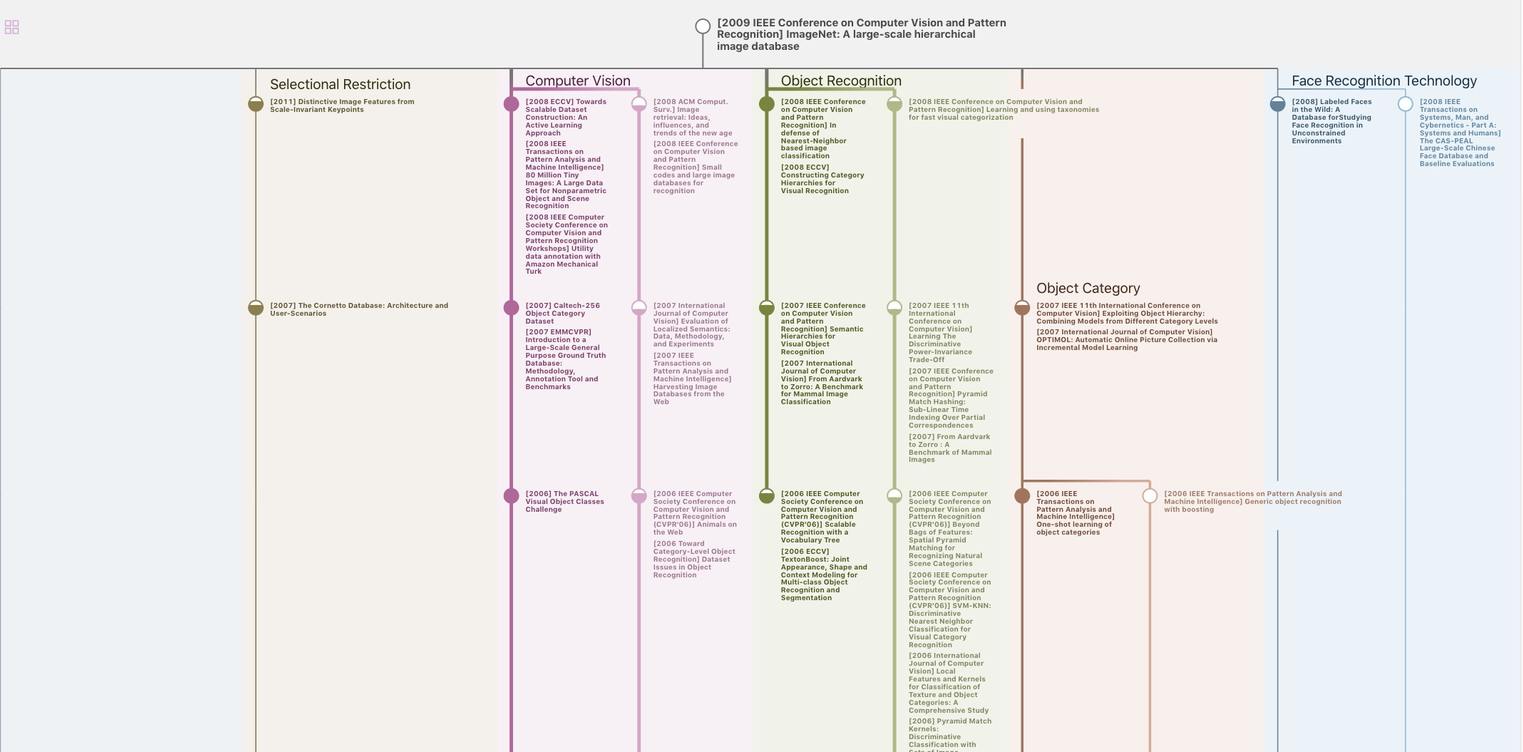
生成溯源树,研究论文发展脉络
Chat Paper
正在生成论文摘要