A cross-disciplinary comparison of multimodal data fusion approaches and applications: Accelerating learning through trans-disciplinary information sharing
Expert Systems with Applications(2021)
摘要
Multimodal data fusion (MMDF) is the process of combining disparate data streams (of different dimensionality, resolution, type, etc.) to generate information in a form that is more understandable or usable. Despite the explosion of data availability in recent decades, as yet there is no well-developed theoretical basis for multimodal data fusion, i.e., no way to determine a priori which approach is best suited to combine an arbitrary set of available data to achieve a stated goal for a given application. This has resulted in exploration of a wide variety of approaches across numerous domains but as yet very little integration of conclusions at a meta (cross-disciplinary) level. In response, this manuscript poses the following questions: (1) How convergent (or divergent) are approaches within single disciplines? (2) How similar are the challenges posed across different disciplines, i.e., might there be opportunity for successes in MMDF achieved in one field to inform progress in other areas as well? and (3) Where are the outstanding gaps in MMDF research, and what does this imply as targets for high impact research in the coming years? To begin to answer these questions, an apples-to-apples comparison of the literature of nine stakeholder-centric engineering domains (civil engineering, transportation, energy, environmental engineering, food engineering, critical care (healthcare), neuroscience, manufacturing/automation, and robotics) was created by quantifying the numbers and dimensionalities of modalities and sensors in each published project and classifying the algorithms used and purposes for which they are used. Within disciplines, it is shown there is often a tendency for use of similar methodologies, both in choice of level of fusion and data algorithm class. Yet this analysis also reveals that many problem types (defined by data dimensionality, modality number and type, and fusion purpose) are shared across different domains and are approached differently in those domains, e.g., transportation problems have similar characteristics to critical care, food science, robotics, and civil engineering. Of the disciplines studied, most (>75%) share problem characteristics with 3–5 others; to support leveraging these resources, lookup tables indexed by data dimensions, number of modalities, etc. are provided as a starting point for cross-disciplinary MMDF literature searches for new applications. Critical gaps identified are (1) a drop off of the number of published studies with increasing number of distinct modalities and (2) a dearth of publications tackling challenges with high dimensionality inputs, especially time-series 2D and 3D data. These gaps may point to topics where algorithm development will be fruitful to enable future solutions as video and other high-dimensionality sensors decrease in price. Finally, the lack of a shared vocabulary across disciplines makes analyses like the one conducted here challenging, as does the often implicit incorporation of expert knowledge into design; therefore progress toward a better leveraging of the current state of knowledge and toward a theoretical MMDF framework depends critically on improved cross-disciplinary communication and coordination on this topic.
更多查看译文
关键词
Multimodal data fusion,Machine learning,Complex systems,Big data,Trans-disciplinary
AI 理解论文
溯源树
样例
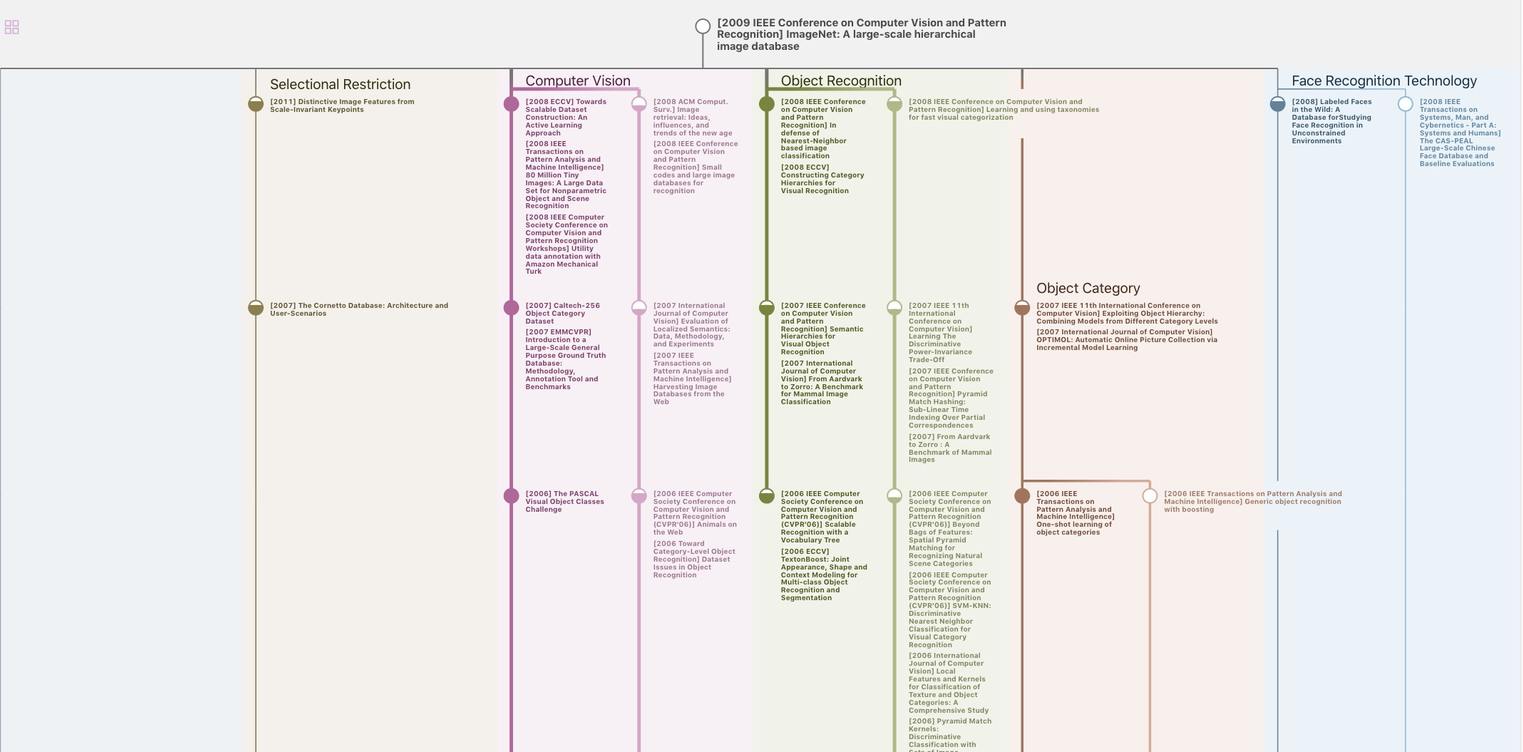
生成溯源树,研究论文发展脉络
Chat Paper
正在生成论文摘要