Improved learning of -parities.
THEORETICAL COMPUTER SCIENCE(2020)
摘要
We consider the problem of learning k-parities in the online mistake-bound model: given a hidden vector x is an element of {0, 1}(n) where the hamming weight of x is k and a sequence of "questions" a(1), a(2), . . . is an element of {0, 1}(n), where the algorithm must reply to each question with < a(i), x > (mod 2), what is the best trade-off between the number of mistakes made by the algorithm and its time complexity? We improve the previous best result of Buhrman et al. [3] by an exp(k) factor in the time complexity. Next, we consider the problem of learning k-parities in the PAC model in the presence of random classification noise of rate eta is an element of (0, 1/2). Here, we observe that even in the presence of classification noise of non-trivial rate, it is possible to learn k-parities in time better than ((n)(k/2)), whereas the current best algorithm for learning noisy k-parities, due to Grigorescu et al. [9], inherently requires time ((n)(k/2)) even when the noise rate is polynomially small. (C) 2020 Elsevier B.V. All rights reserved.
更多查看译文
关键词
Learning sparse parities,Learning sparse parities with noise,Mistake bound model,PAC model,Learning k parities
AI 理解论文
溯源树
样例
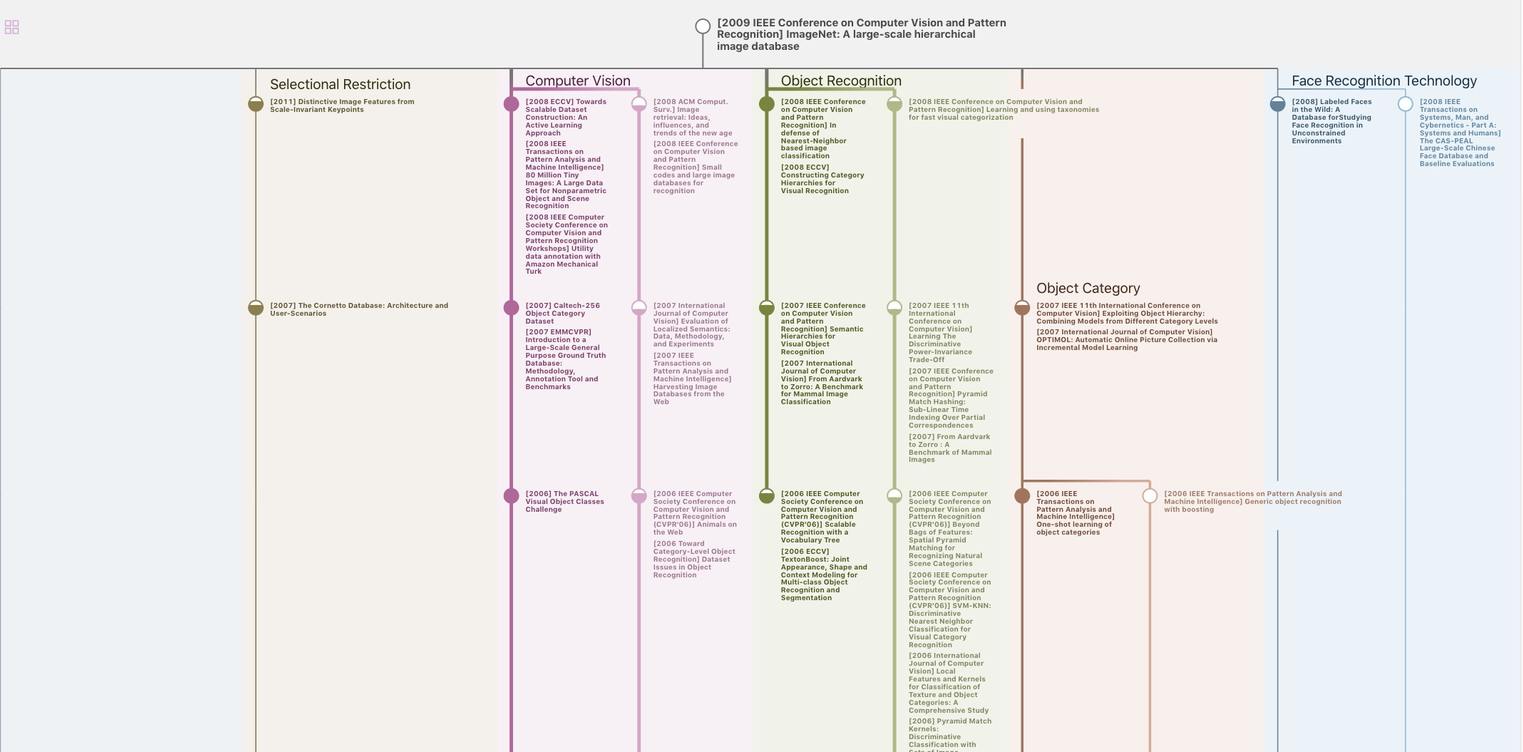
生成溯源树,研究论文发展脉络
Chat Paper
正在生成论文摘要