Learning to Walk : Bio-Mimetic Hexapod Locomotion via Reinforcement-Based Spiking Central Pattern Generation
IEEE Journal on Emerging and Selected Topics in Circuits and Systems(2020)
摘要
Online learning for the legged robot locomotion under performance and energy constraints remains to be a challenge. Methods such as stochastic gradient, deep reinforcement learning (RL) have been explored for bipeds, quadrupeds and hexapods. These techniques are computationally intensive and thus difficult to implement on edge computing platforms. These methods are also inefficient in energy consumption and throughput because of their reliance on complex sensors and pre-processing of data. On the other hand, neuromorphic computing paradigms, such as spiking neural networks (SNN), become increasingly favorable in low power computing on edge intelligence. SNN has exhibited the capability of performing reinforcement learning mechanisms with biomimetic spike time-dependent plasticity (STDP) of synapses. However, training a legged robot to walk in the synchronized gait patterns generated by a central pattern generator (CPG) in an SNN framework has not yet been explored. Such a method can combine the efficiency of SNNs with the synchronized locomotion of CPG based systems - providing breakthrough performance improvement of end-to-end learning in mobile robotics. In this paper, we propose a reinforcement based stochastic learning technique for training a spiking CPG for a hexapod robot which learns to walk using bio-inspired tripod gait without prior knowledge. The whole system is implemented on a lightweight raspberry pi platform with integrated sensors. Our method opens new opportunities for online learning with limited edge computing resources.
更多查看译文
关键词
Central pattern generator,spiking neural networks,spike time-dependent plasticity,stochastic reinforcement-based STDP,robotic locomotion
AI 理解论文
溯源树
样例
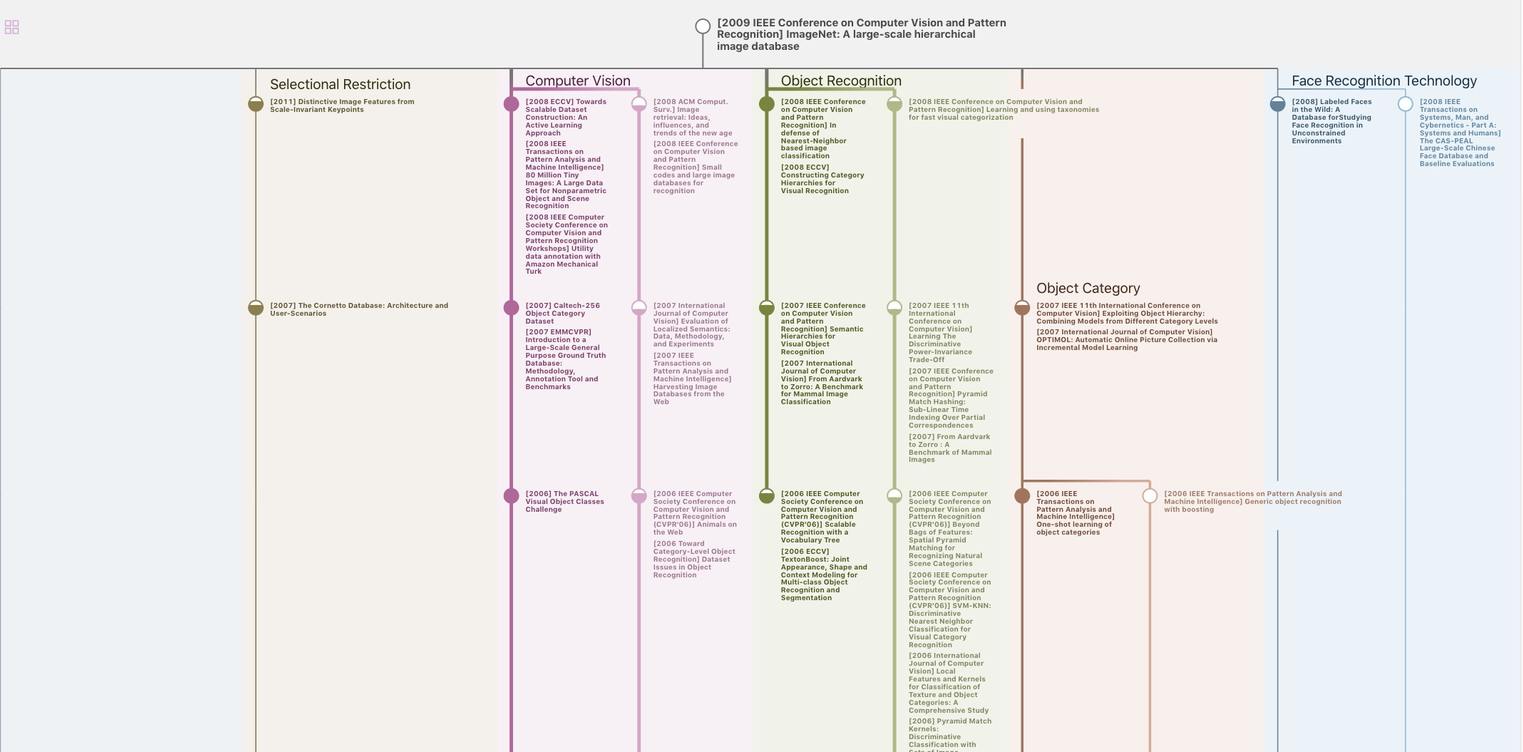
生成溯源树,研究论文发展脉络
Chat Paper
正在生成论文摘要