An Accurate And Real-Time Surface Defects Detection Method For Sawn Lumber
IEEE TRANSACTIONS ON INSTRUMENTATION AND MEASUREMENT(2021)
摘要
Although one-stage detectors, for example, YOLOv3, SSD, and RefineDet, based on the convolutional neural networks (CNNs) have been widely used in object detection, their localization accuracy of the defects is still poor and the problem of repeated detection still occurs. This article proposes a surface defects detection method based on improved YOLOv3 for sawn lumbers. First, a Gaussian YOLOv3 is used to estimate the coordinates and the localization uncertainty of the prediction box. Then, the complete intersection over union (CIoU) loss function is adopted to replace the intersection over union (IoU) loss for non-maximum suppression (NMS), because it considers the overlap area, the center point, and the aspect ratio, and thus can reduce the repeated detection. Finally, this article establishes two data sets, that is, the rubber lumber data set containing four kinds of defects and the pine lumber data set containing two kinds of defects. In total, there are 16 633 images and 6705 annotated images in these two data sets. The effectiveness of the proposed method has been validated by experiments.
更多查看译文
关键词
Complete intersection over union (CIoU) loss, Gaussian function, improved Yolov3, sawn lumber data sets, surface defects detection
AI 理解论文
溯源树
样例
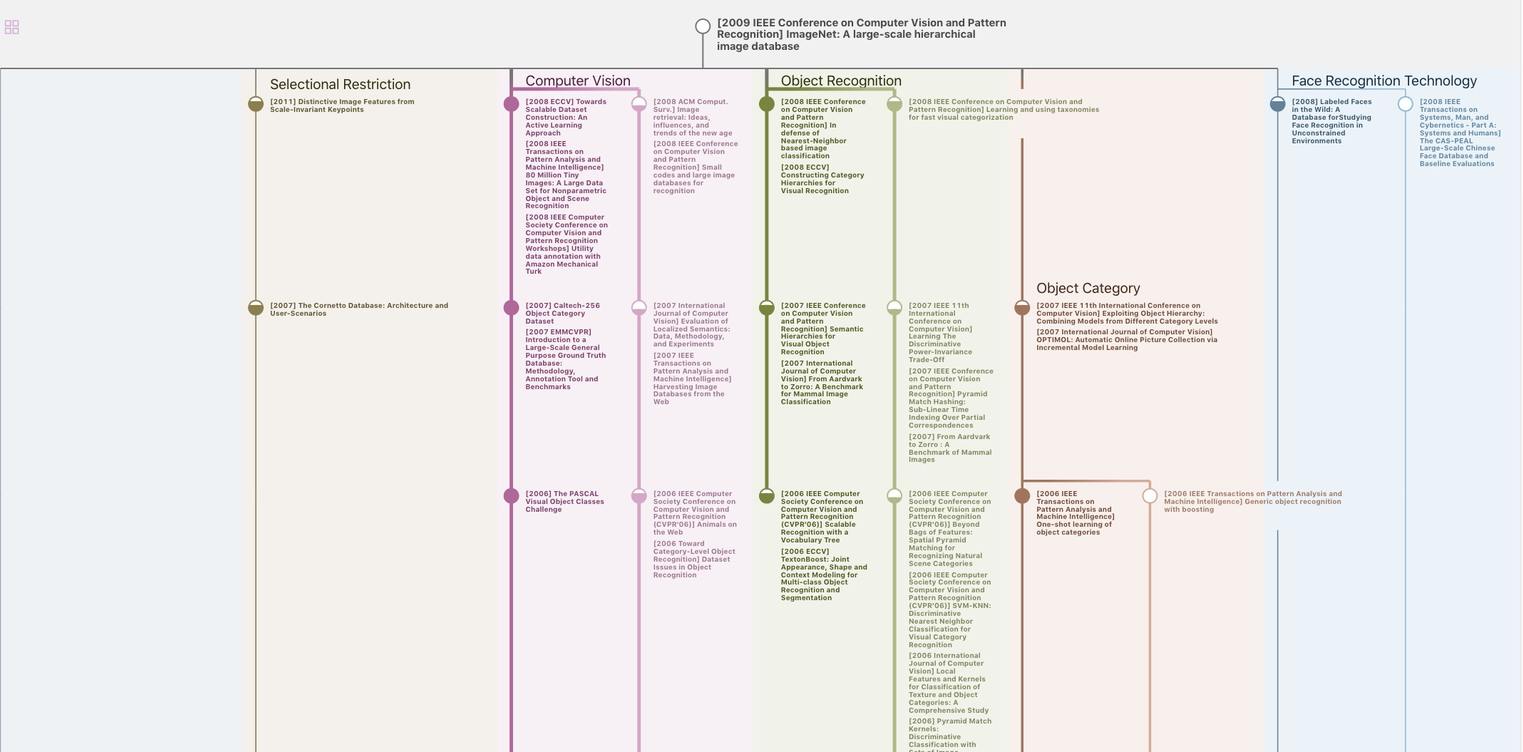
生成溯源树,研究论文发展脉络
Chat Paper
正在生成论文摘要