Compact Mixed-Signal Convolutional Neural Network Using a Single Modular Neuron
IEEE Transactions on Circuits and Systems I: Regular Papers(2020)
摘要
This paper demonstrates a compact mixed-signal (MS) convolutional neural network (CNN) design procedure by proposing a MS modular neuron unit that alleviates analog circuit related design issues such as noise. Through the first step of the proposed procedure, we design a CNN in software with a minimized number of channels for each layer while satisfying the network performance to the target, which creates low representational and computational cost. Then, the network is reconstructed and retrained with a single modular neuron that is recursively utilized for the entire network for the maximum hardware efficiency with a fixed number of parameters that consider signal attenuation. For the last step of the proposed procedure, the parameters of the networks are quantized to an implementable level of MS neurons. We designed the networks for MNIST and Cifar-10 and achieved compact CNNs with a single MS neuron with 97% accuracy for MNIST and 85% accuracy for Cifar-10 whose representational cost and computational cost are reduced to least two times smaller than prior works. The estimated energy per classification of the hardware network for Cifar-10 with a single MS neuron, designed with optimum noise and matching requirements, is 0.5μ J, which is five times smaller than its digital counterpart.
更多查看译文
关键词
Deep neural network,convolutional neural network,mixed-signal neuron,modular neuron,compact neural network,representational cost,computational cost,network retraining
AI 理解论文
溯源树
样例
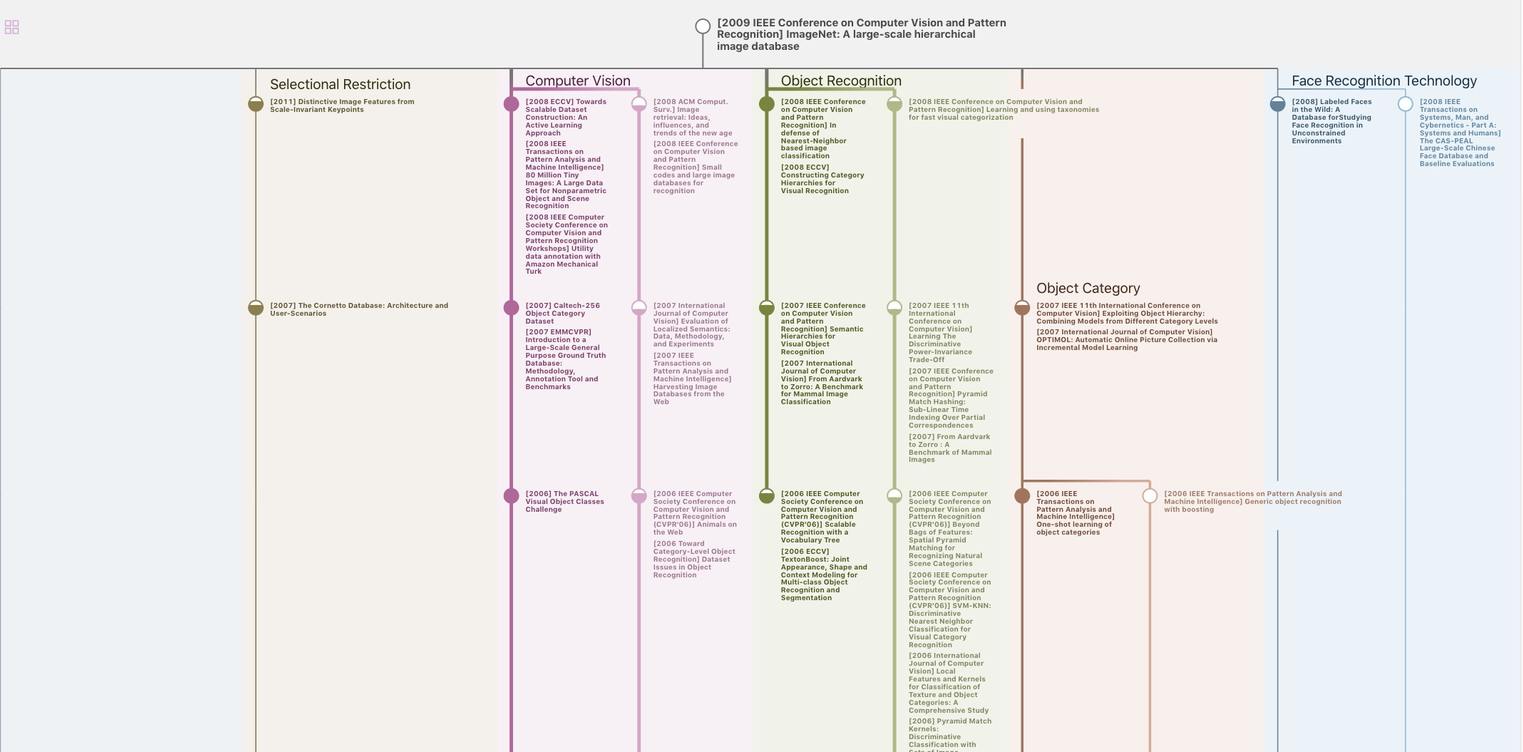
生成溯源树,研究论文发展脉络
Chat Paper
正在生成论文摘要