Statistical Learning For Accurate And Interpretable Battery Lifetime Prediction
JOURNAL OF THE ELECTROCHEMICAL SOCIETY(2021)
摘要
Data-driven methods for battery lifetime prediction are attracting increasing attention for applications in which the degradation mechanisms are poorly understood and suitable training sets are available. However, while advanced machine learning and deep learning methods promise high performance with minimal data preprocessing, simpler linear models with engineered features often achieve comparable performance, especially for small training sets, while also providing physical and statistical interpretability. In this work, we use a previously published dataset to develop simple, accurate, and interpretable data-driven models for battery lifetime prediction. We first present the "capacity matrix" concept as a compact representation of battery electrochemical cycling data, along with a series of feature representations. We then create a number of univariate and multivariate models, many of which achieve comparable performance to the highest-performing models previously published for this dataset; thus, our work can serve as a comprehensive benchmarking study for this dataset. These models also provide insights into the degradation of these cells. Our approaches can be used both to quickly train models for a new battery cycling dataset and to benchmark the performance of more advanced machine learning methods.
更多查看译文
关键词
Batteries-Li-ion, Battery lifetime prediction, Cycle life prediction, Statistical learning, Machine learning
AI 理解论文
溯源树
样例
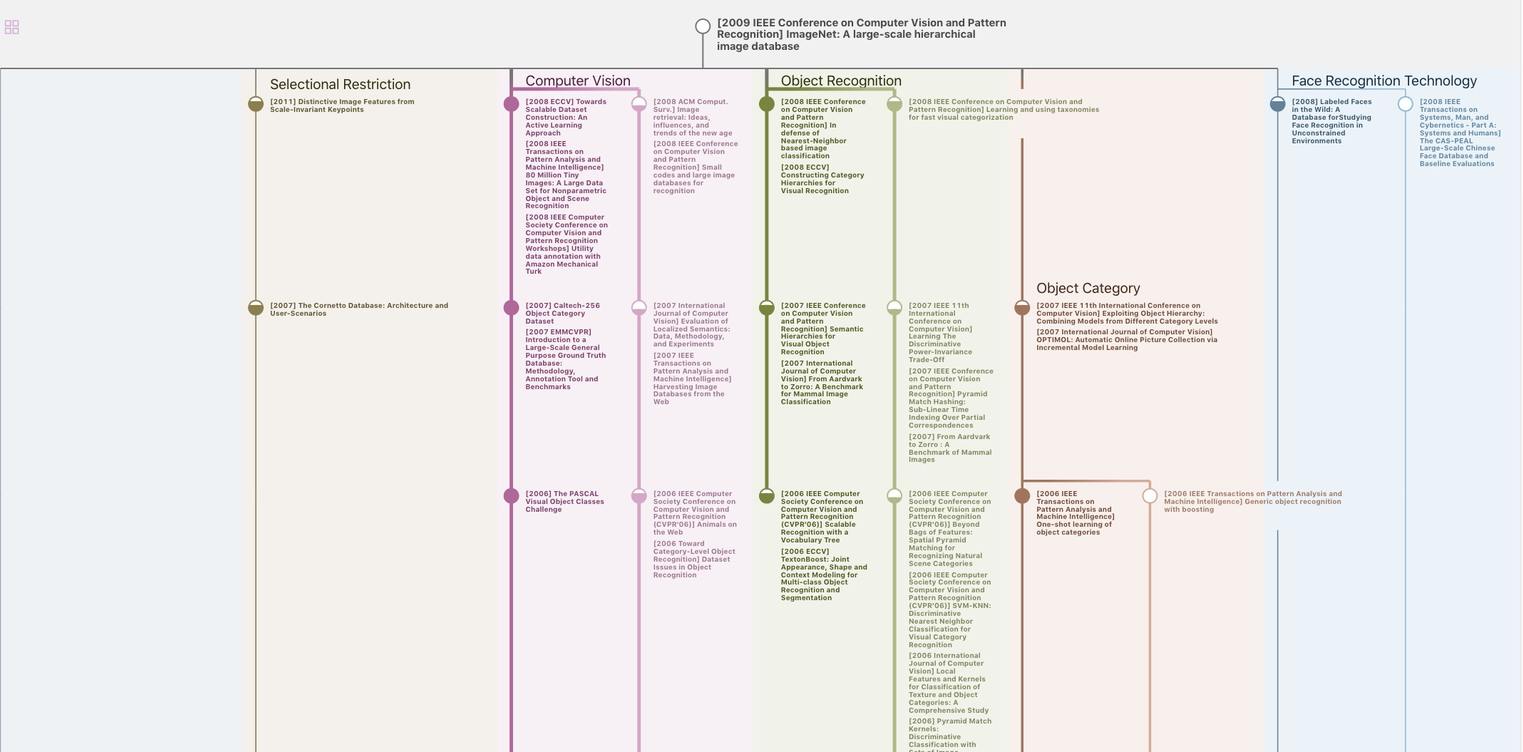
生成溯源树,研究论文发展脉络
Chat Paper
正在生成论文摘要