Area- And Energy-Efficient Stdp Learning Algorithm For Spiking Neural Network Soc
IEEE ACCESS(2020)
摘要
Recently, spiking neural networks have gained attention owing to their energy efficiency. All-to-all spike-time dependent plasticity is a popular learning algorithm for spiking neural networks because it is suitable for nondifferentiable spike event-based learning and requires fewer computations than back-propagation-based algorithms. However, the hardware implementation of all-to-all spike-time dependent plasticity is limited by the large storage area required for spike history and large energy consumption caused by frequent memory access. We propose a time-step scaled spike-time dependent plasticity to reduce the storage area required for spike history by reducing the area of the spike-time dependent plasticity learning circuit by 60% and a post-neuron spike-referred spike-time dependent plasticity to reduce the energy consumption by 99.1% by efficiently accessing the memory while learning. The accuracy of Modified National Institute of Standards and Technology image classification degraded by less than 2% when both time-step scaled spike-time dependent plasticity and post-neuron spike-referred spike-time dependent plasticity were applied. Thus, the proposed hardware-friendly spike-time dependent plasticity algorithms make all-to-all spike-time dependent plasticity implementable in more compact areas while reducing energy consumption and experiencing insignificant accuracy degradation.
更多查看译文
关键词
Neurons, History, Synapses, Artificial neural networks, Firing, Hardware, Biological neural networks, Spike-time dependent plasticity (STDP), time-step scaled STDP (TS-STDP), post-neuron spike-referred STDP (PR-STDP), spiking neural network (SNN)
AI 理解论文
溯源树
样例
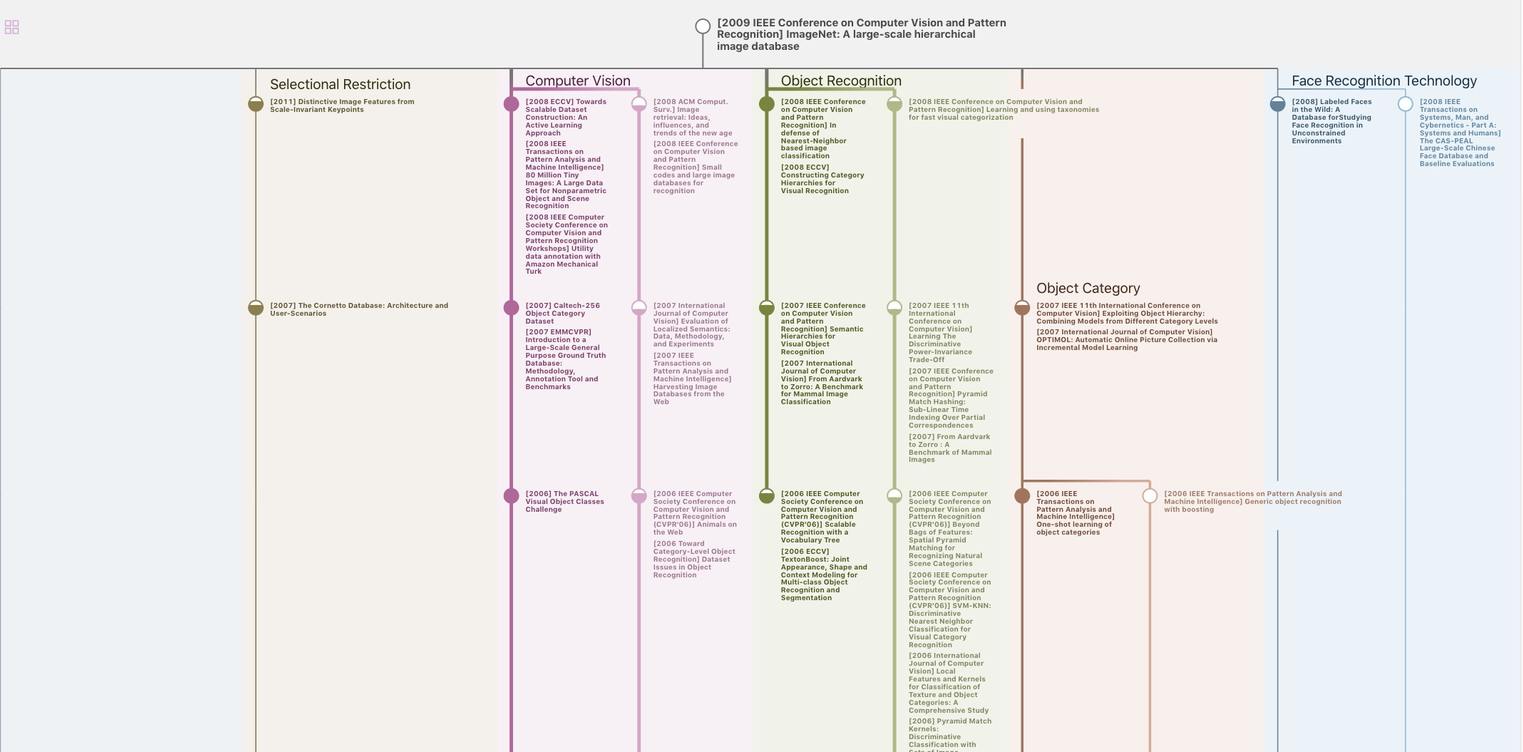
生成溯源树,研究论文发展脉络
Chat Paper
正在生成论文摘要