Improved Configuration Of Transmission Variables For Lorawan In High-Noise Channels
2020 28TH IRANIAN CONFERENCE ON ELECTRICAL ENGINEERING (ICEE)(2020)
摘要
The long-range wide-area network (LoRaWAN) as a promising communication technology for the Internet of Things (IoT) has attracted a lot of attention from both industry and academia. The LoRaWAN covers thousands of connected things over a wide network, with low energy consumption. However, increasing the number of things in the LoRaWAN networks affects the reliability, scalability, and energy consumption of the network which results in decreasing the packet delivery ratio. In LoRaWAN networks, the adaptive data rate (ADR) method is an approach for adjusting the transmission variables of a thing aiming at improving the communication quality between the thing and the gateway. This paper studies the main ADR method and the other proposed algorithms for the LoRa networks involving a high number of things considering variable frequency channel conditions. Our studies illustrate that by employing the ADR algorithm when the noise of the channel is high and the number of end devices is numerous, the packet delivery ratio is reduced while consumed energy by each node is significantly increased. In this paper, to improve the performance of the main ADR algorithm, we propose a new method to adjust the transmission variables consisting of the spread factor and transmission power with respect to the high-noisy channels for the LoRa networks. Our proposed algorithm determines the transmission variables in such a way that the packet delivery ratio is increased while energy consumption is reduced. The simulation results illustrate that the proposed algorithm improves the packet delivery ratio and reduced energy consumption more than 4 times compared to the main ADR algorithm considering the sub-urban area with high-noise channels.
更多查看译文
关键词
IoT, adaptive data rate, configuration management, packet delivery ratio
AI 理解论文
溯源树
样例
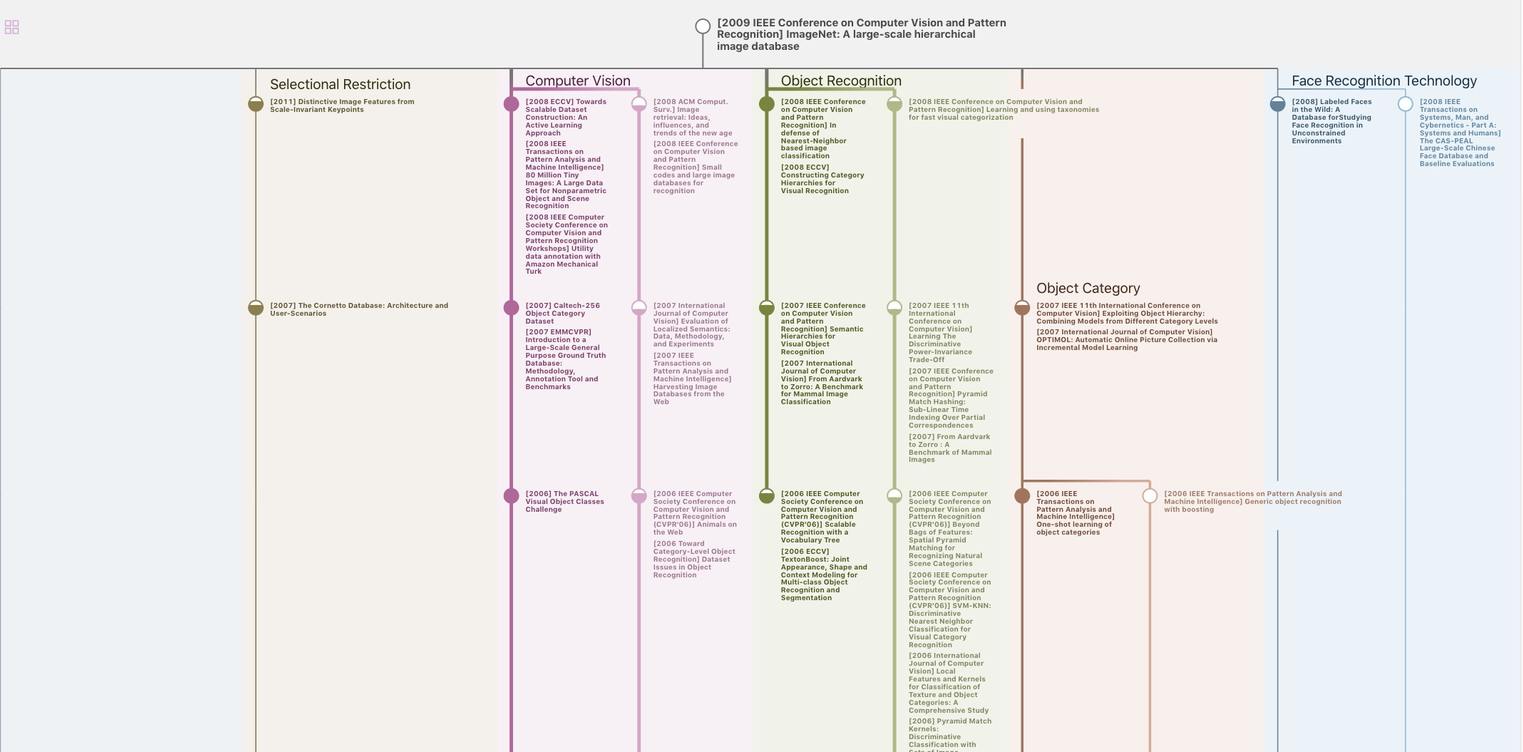
生成溯源树,研究论文发展脉络
Chat Paper
正在生成论文摘要