DR-KFS: A Differentiable Visual Similarity Metric for 3D Shape Reconstruction
European Conference on Computer Vision(2020)
摘要
We introduce a differential visual similarity metric to train deep neural networks for 3D reconstruction, aimed at improving reconstruction quality. The metric compares two 3D shapes by measuring distances between multi-view images differentiably rendered from the shapes. Importantly, the image-space distance is also differentiable and measures visual similarity, rather than pixel-wise distortion. Specifically, the similarity is defined by mean-squared errors over HardNet features computed from probabilistic keypoint maps of the compared images. Our differential visual shape similarity metric can be easily plugged into various 3D reconstruction networks, replacing their distortion-based losses, such as Chamfer or Earth Mover distances, so as to optimize the network weights to produce reconstructions with better structural fidelity and visual quality. We demonstrate this both objectively, using well-known shape metrics for retrieval and classification tasks that are independent from our new metric, and subjectively through a perceptual study.
更多查看译文
关键词
differentiable visual similarity metric,3d shape
AI 理解论文
溯源树
样例
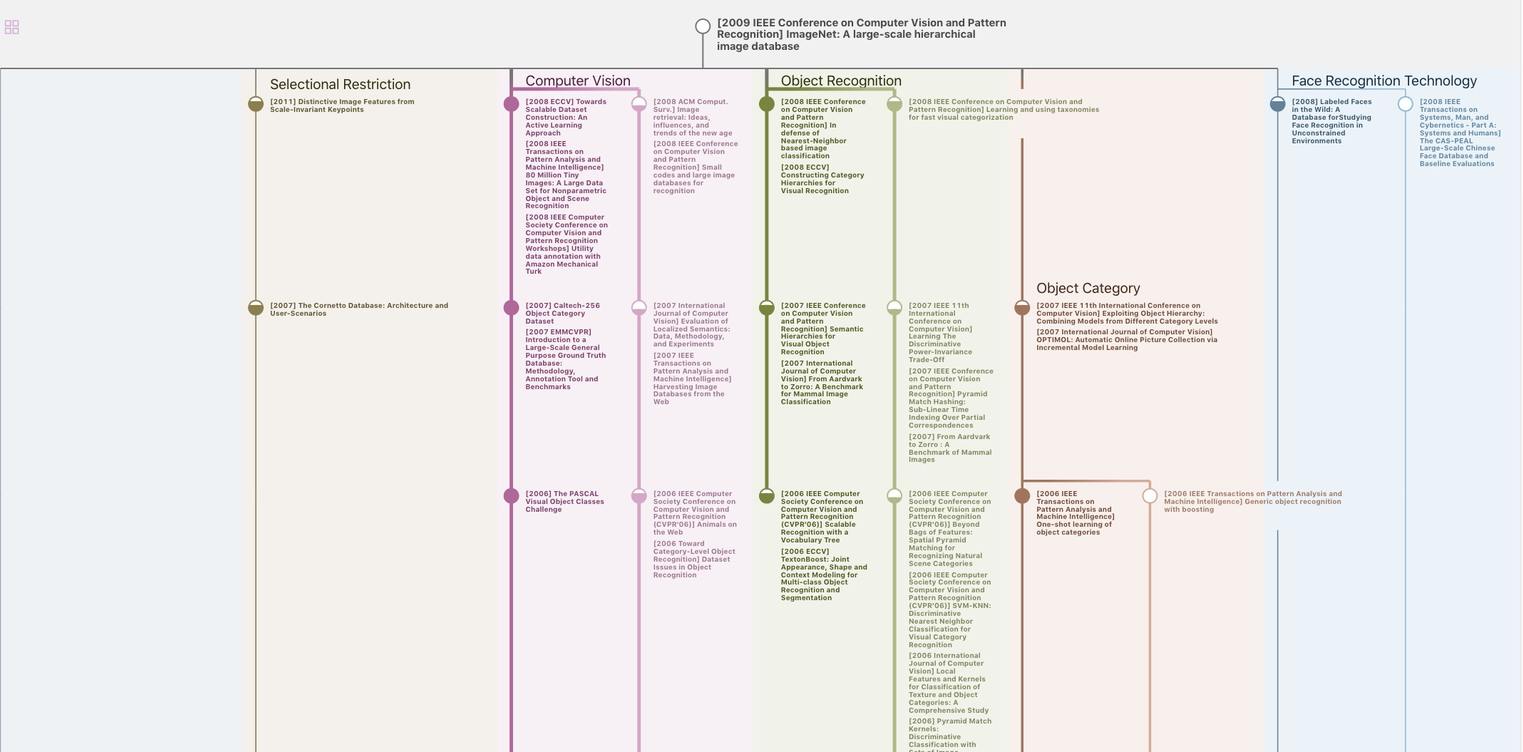
生成溯源树,研究论文发展脉络
Chat Paper
正在生成论文摘要