An Efficient And Effective Model Based On Mean Positive Examples For Social Image Annotation
IEEE ACCESS(2020)
摘要
Nowadays, with the rapid growth of imaging and social network, huge volumes of image data are produced and shared on social media. Social image annotation has been an important and challenging task in the fields of computer vision and machine learning, which can facilitate large-scale image retrieval, indexing, and management. The four most challenges of social image annotation are semantic gap, tag refinement, label-imbalance, and annotation efficiency. To address these issues, we propose an efficient and effective annotation method based on the Mean of Positive Examples (MPE) corresponding to each label. First, we refine user-provided noisy tags by our proposed local smoothing process, and consider the refined tags as key features in contrast to the previous methods that consider them as side information, which significantly improves annotation performance. Second, we propose a weighted trans-media similarity measure method that fuses all modality information in identifying proper neighbors, which promotes the semantic level and eases image annotation. Third, our MPE model gives equal importance to all labels, thus, improving the annotation performance of infrequent labels without sacrificing that of frequent labels. Fourth, our MPE model can dramatically decrease space-time overheads, since the time cost of annotating an image is unaffected by the size of the training image dataset, but relying on the size of label vocabulary. Therefore, our proposed method can be applied to real-world large-scale online social image repositories. Extensive experiments on both benchmark datasets demonstrate the effectiveness and efficiency of our MPE model.
更多查看译文
关键词
Image annotation, Semantics, Annotations, Visualization, Training, Task analysis, Social networking (online), Automatic image annotation, social image, tag refinement, semantic gap
AI 理解论文
溯源树
样例
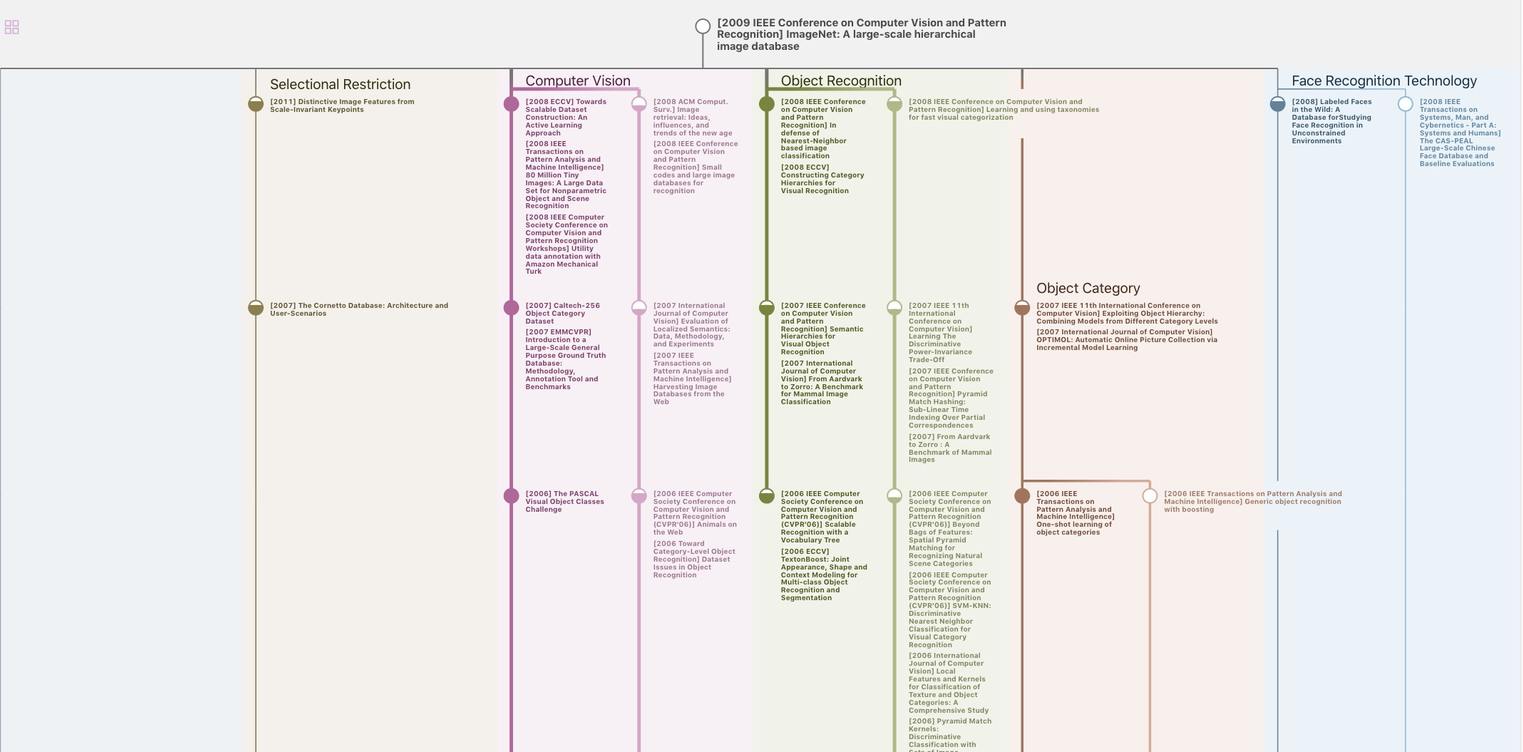
生成溯源树,研究论文发展脉络
Chat Paper
正在生成论文摘要