Learning Domain-Invariant And Discriminative Features For Homogeneous Unsupervised Domain Adaptationinspec Keywordsother Keywordskey Words
Chinese Journal of Electronics(2020)
摘要
A classifier trained on the label-rich source dataset tends to perform poorly on the unlabeled target dataset because of the distribution discrepancy across different datasets. Unsupervised domain adaptation aims to transfer knowledge from the labeled source dataset to the unlabeled target dataset to solve this problem. Most of the existing unsupervised domain adaptation methods only concentrate on learning domain-invariant features across different domains, but they neglect the discriminability of the learned features to satisfy the cluster assumption. In this paper, we propose Semantic pairwise centroid alignment (SPCA), which is a point-wise method to learn both domain-invariant and discriminative features for homogeneous unsupervised domain adaptation. SPCA utilizes a novel semantic centroid loss to reduce the intraclass distance in feature space by using source data and target High-confidence centroid points (HCCPs). Then a classifier trained on source features is expected to generalize well on target features. Extensive experiments on visual recognition tasks verify the effectiveness of the proposed SPCA and also demonstrate that both domaininvariant and discriminative features learned by SPCA can significantly boost the performance of homogeneous unsupervised domain adaptation.
更多查看译文
关键词
image classification, unsupervised learning, visual recognition, HCCP, high-confidence centroid points, point-wise method, semantic pairwise centroid alignment, homogeneous unsupervised domain adaptation methods, domaininvariant features, SPCA, learning domain-invariant features, unlabeled target dataset, label-rich source dataset, Transfer learning, Domain adaptation, Semantic alignment, Feature extraction
AI 理解论文
溯源树
样例
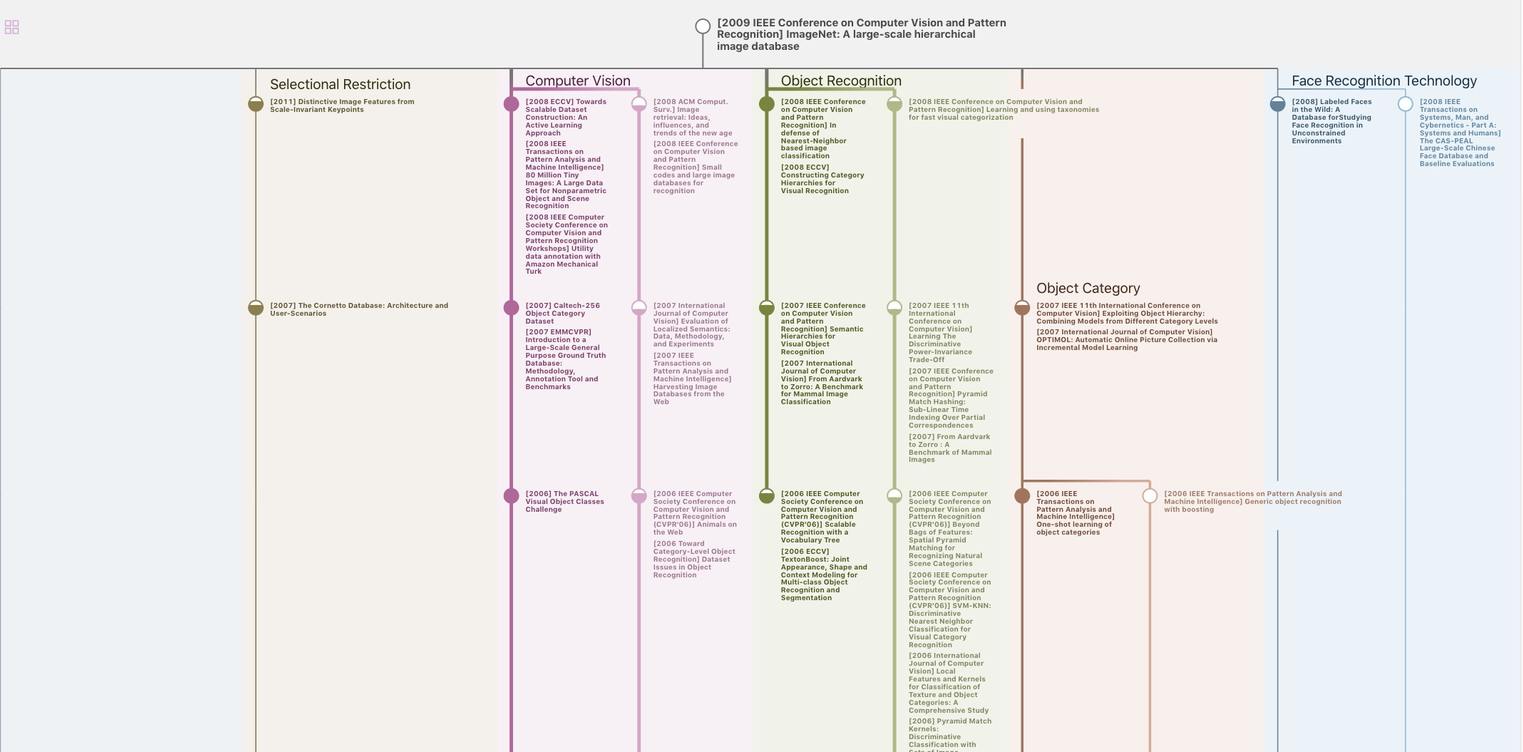
生成溯源树,研究论文发展脉络
Chat Paper
正在生成论文摘要