LOS/NLOS Identification for Indoor UWB Positioning Based on Morlet Wavelet Transform and Convolutional Neural Networks
IEEE Communications Letters(2021)
摘要
In indoor ultra-wideband (UWB) positioning systems, positioning accuracy can be improved by determining the conditions of line-of-sight (LOS) and non-line-of-sight (NLOS) propagation and taking appropriate measures. The existing methods, such as support vector machine (SVM), decision tree (DT), k-Nearest Neighbor (KNN), identify LOS/NLOS mainly using time-domain characteristics. However, using only time-domain characteristics cannot achieve satisfactory performance. In this letter, we propose a LOS/NLOS identification method based on Morlet wave transform and convolutional neural networks (MWT-CNN). MWT-CNN is capable of identifying LOS/NLOS in the time-frequency domain. Our simulations are based on the 802.15.4a UWB model and an open-source dataset. The simulation results show that MWT-CNN achieves an accuracy of 100% in the office scenario, 99.89% in the industrial scenario, 96.10% in the residential scenario, and 98.84% in a real experimental scenario. Further simulation results show that MWT-CNN is more suitable to be deployed in static scenarios.
更多查看译文
关键词
UWB,LOS/NLOS identification,wavelet transform,convolutional neural networks
AI 理解论文
溯源树
样例
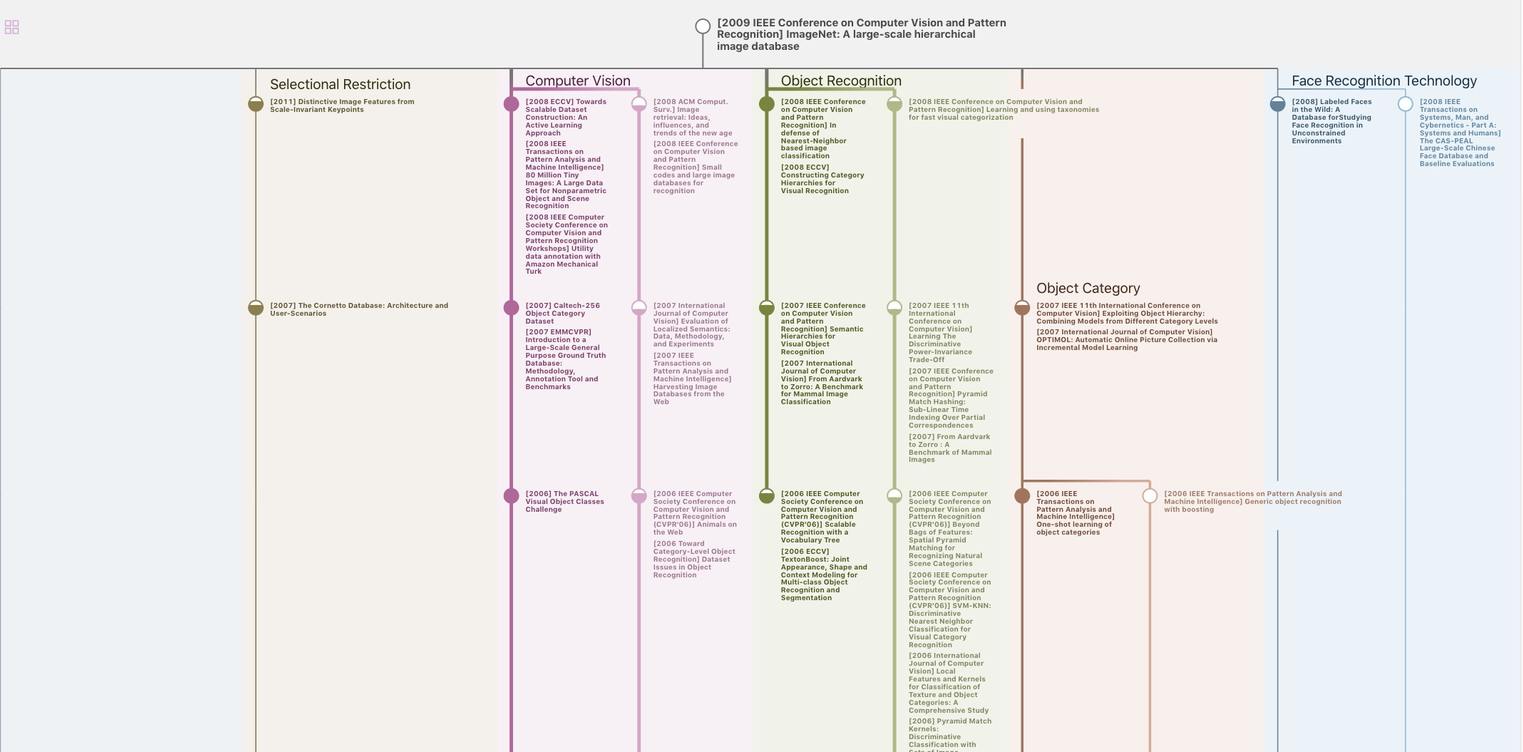
生成溯源树,研究论文发展脉络
Chat Paper
正在生成论文摘要