Improved State Stability Of Hfo2 Ferroelectric Tunnel Junction By Template-Induced Crystallization And Remote Scavenging For Efficient In-Memory Reinforcement Learning
2020 IEEE SYMPOSIUM ON VLSI TECHNOLOGY(2020)
摘要
We investigated the effects of read current instabilities originated from depolarization field and charge trapping in HfO2 ferroelectric tunnel junctions (FTJs) on the performance of in-memory reinforcement learning. We utilized, for the first time, remote scavenging to control interfacial layer thickness, combined with template-induced crystallization to stabilize the ferroelectric phase. These are found to improve both short-term and long-term stability of memory state. Pole-cart simulation results reveal that these improvements significantly contribute to the efficiency and stability of reinforcement learning with the FTJ cross-point array.
更多查看译文
关键词
FTJ cross-point array,Polecart simulation,long-term memory stability,short-term memory stability,interfacial layer thickness,HfO2 ferroelectric tunnel junction,ferroelectric phase stabilization,charge trapping,depolarization field,read current instabilities,in-memory reinforcement learning,remote scavenging,template-induced crystallization,improved state stability,HfO2
AI 理解论文
溯源树
样例
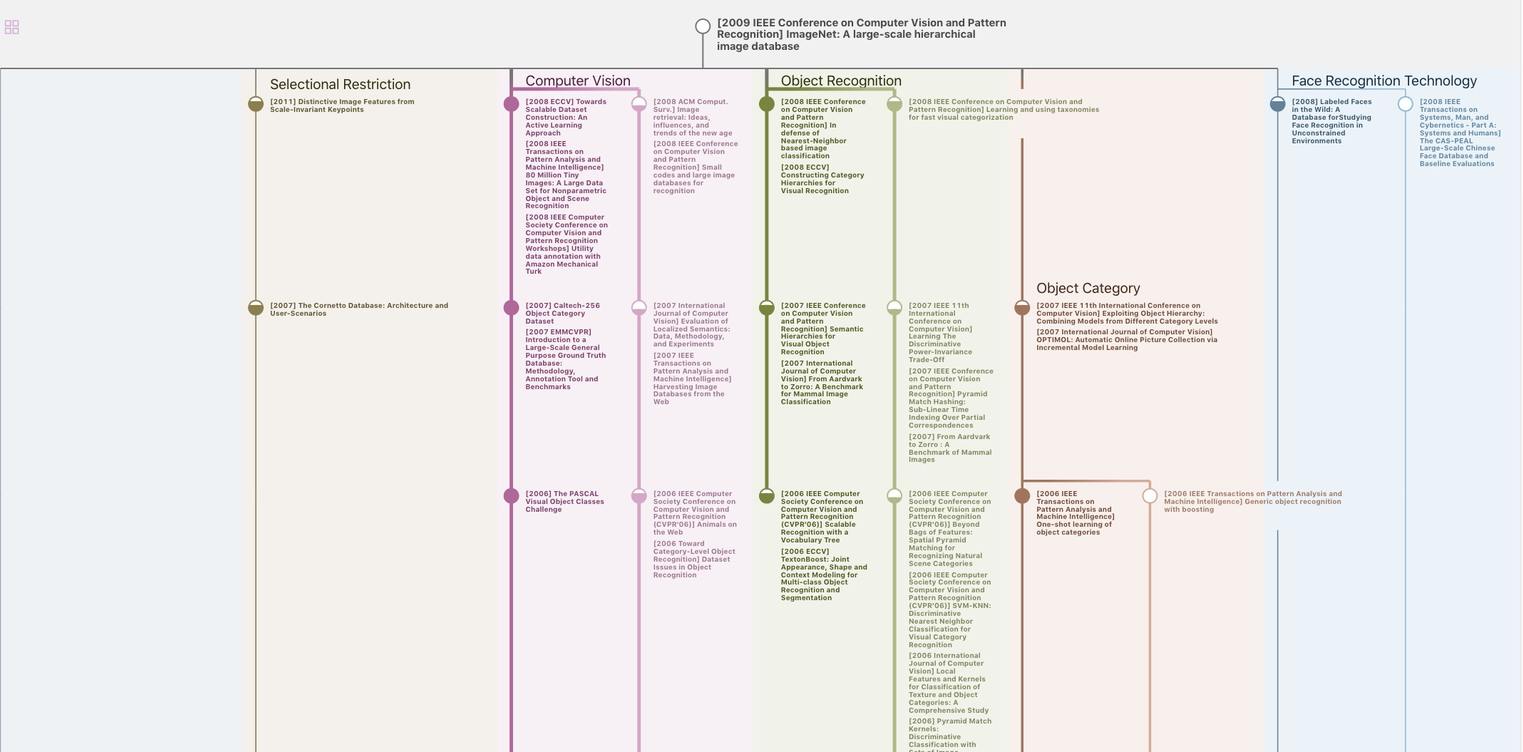
生成溯源树,研究论文发展脉络
Chat Paper
正在生成论文摘要