Deep Learning Based Approach Implemented to Image Super-Resolution
Journal of Advances in Information Technology(2020)
摘要
The aim of this research is about application of deep learning approach to the inverse problem, which is one of the most popular issues that has been concerned for many years about, the image Super-Resolution (SR). From then on, many fields of machine learning and deep learning have gained a lot of momentum in solving such imaging problems. In this article, we review the deep-learning techniques for solving the image super-resolution especially about the Generative Adversarial Network (GAN) technique and discuss other ways to use the GAN for an efficient solution on the task. More specifically, we review about the Enhanced Super-Resolution Generative Adversarial Network (ESRGAN) and Residual in Residual Dense Network (RRDN) that are introduced by 'idealo' team and evaluate their results for image SR, they had generated precise results that gained the high rank on the leader board of state-of-the-art techniques with many other datasets like Set5, Set14 or DIV2K, etc. To be more specific, we will also review the Single-Image Super-Resolution using Generative Adversarial Network (SRGAN) and the Enhanced Super-Resolution Generative Adversarial Networks (ESRGAN), two famous state-of-the-art techniques, by re-train the proposed model with different parameter and comparing with their result. So that can be helping us understand the working of announced model and the different when we choose others parameter compared to theirs.
更多查看译文
关键词
image super-resolution, deep learning, inverse problems, Residual in Residual Dense Network (RRDN), Generative Adversarial Network (GAN), Enhanced Super-Resolution Generative Adversarial Networks (ESRGAN)
AI 理解论文
溯源树
样例
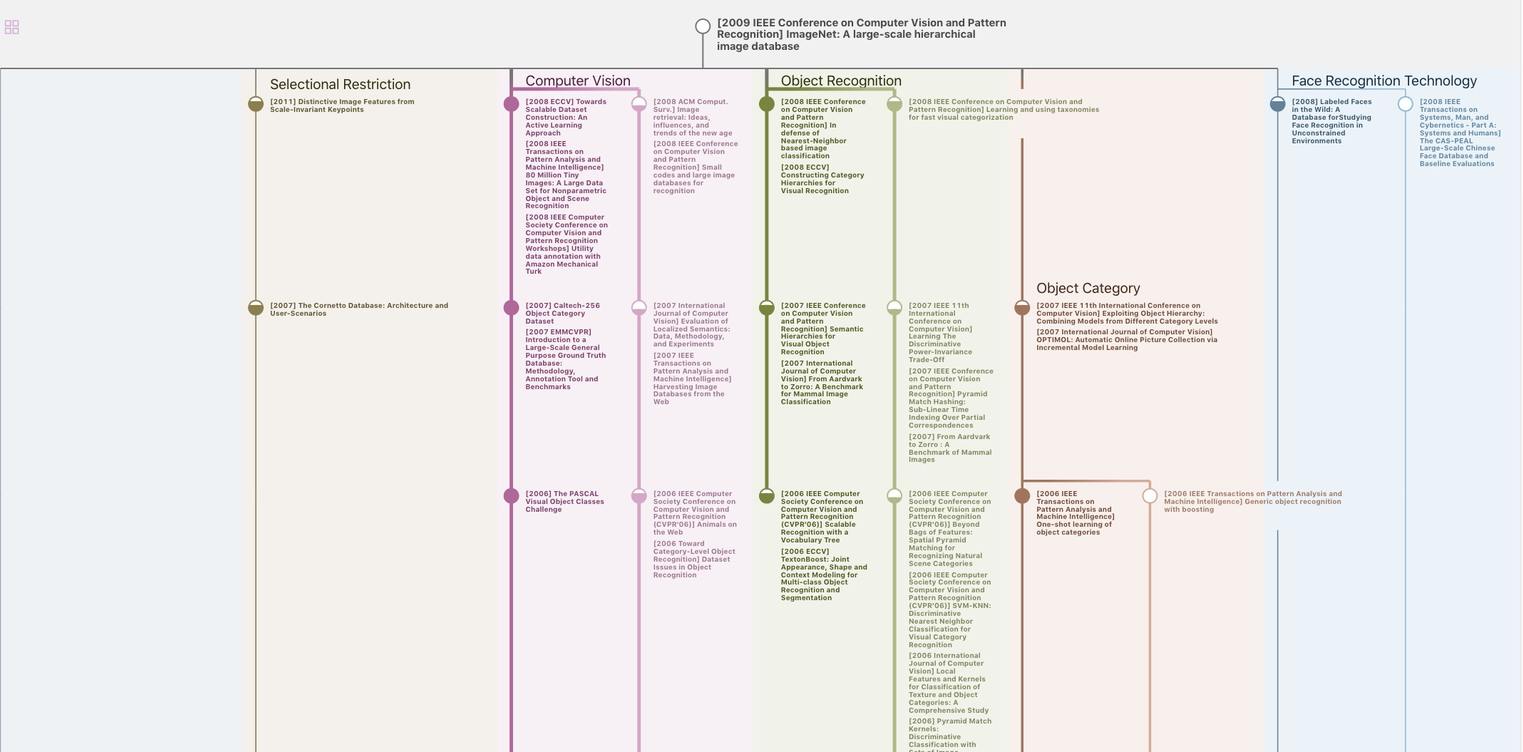
生成溯源树,研究论文发展脉络
Chat Paper
正在生成论文摘要