Altering Gaussian Process To Student-T Process For Maximum Distribution Construction
INTERNATIONAL JOURNAL OF SYSTEMS SCIENCE(2021)
摘要
Gaussian process (GP) regression is widely used to find the extreme of a black-box function by iteratively approximating an objective function when new evaluation obtained. Such evaluation is usually made by optimising a given acquisition function. However, for non-parametric Bayesian optimisation, the extreme of the objective function is not a deterministic value, but a random variant with distribution. We call such distribution the maximum distribution which is generally non-analytical. To construct such maximum distribution, traditional GP regression method by optimising an acquisition function is computational cost as the GP model has a cubic computation of training data. Moreover, the introduction of acquisition function brings extra hyperparameters which made the optimisation more complicated. Recently, inspired by the idea of Sequential Monte Carlo method and its application in Bayesian optimisation, a Monte Carlo alike method is proposed to approximate the maximum distribution with weighted samples. Alternative to the method on GP model, we construct the maximum distribution within the framework of Student-t process (TP) which considers more uncertainties from the training data. Toy examples and real data experiment show the TP-based Monte Carlo maximum distribution has a competitive performance to the GP-based method.
更多查看译文
关键词
Gaussian process regression, Student-t process regression, Maximum distribution, Sequential Monte Carlo, Bayesian optimisation
AI 理解论文
溯源树
样例
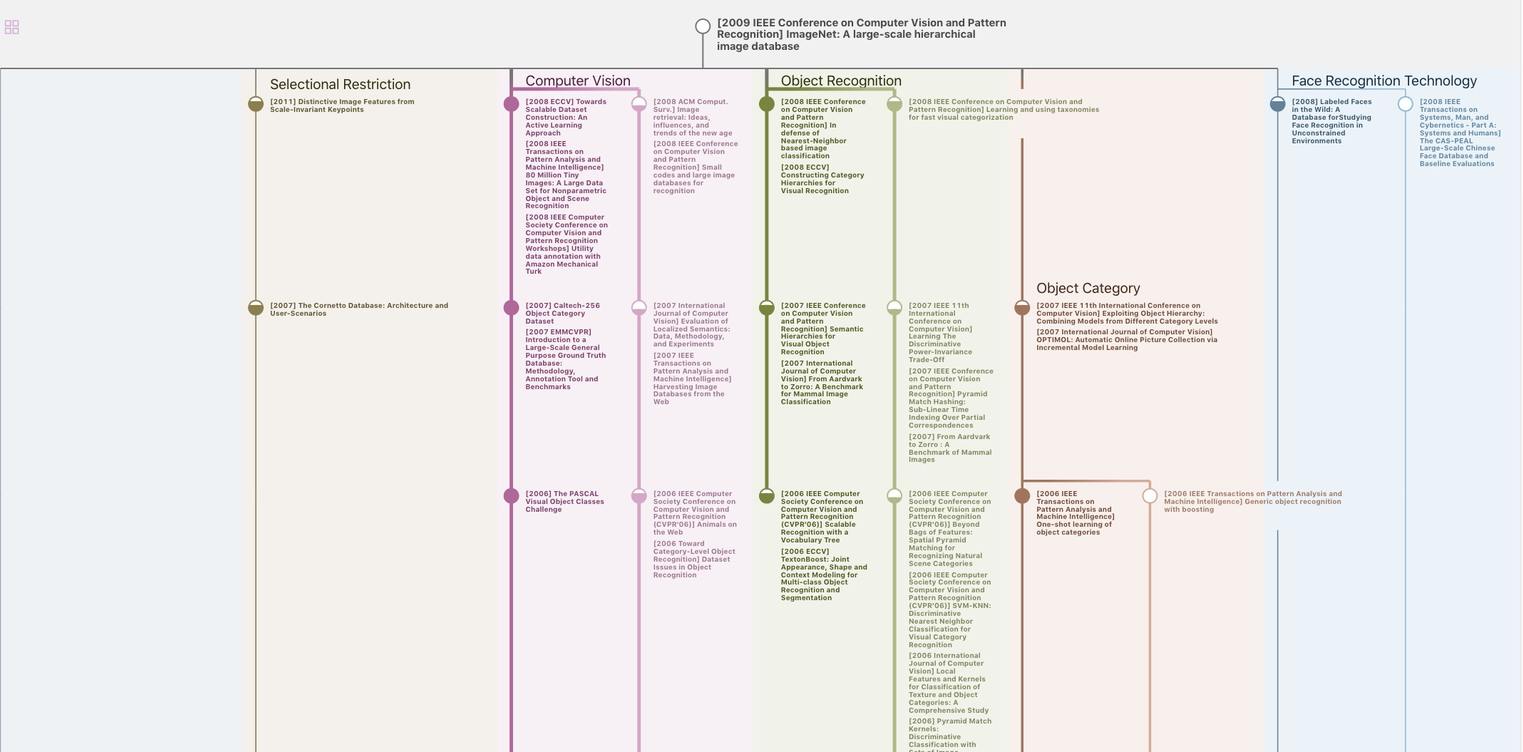
生成溯源树,研究论文发展脉络
Chat Paper
正在生成论文摘要