Real-time video dehazing via incremental transmission learning and spatial-temporally coherent regularization.
Neurocomputing(2021)
摘要
Video dehazing is of crucial importance to the outdoor surveillance, and its main challenges mainly come from the spatial-temporal coherence and computational efficiency. This paper presents an efficient real-time video dehazing approach via incremental transmission learning and spatial-temporally coherent regularization, while explicitly suppressing the possible visual artifacts. First, we propose to initialize the transmission map frame-by-frame by a boundary constrained open dark channel model. Then, supposing a scene point yields highly correlated transmission values between adjacent frames, we impose a temporally coherent term to maintain the temporal consistency of consecutive transmission values, and simultaneously derive an incremental transmission adjusting term to adapt the abrupt scene depth changes between the adjacent frames. Accordingly, the highly correlated and spatial-temporally coherent transmission maps can be optimized for each video frame dehazing, whereby the flickering artifacts can be well reduced. Finally, we group each haze/haze-free images in pair and further utilize the guided joint bilateral filter to suppress the visual artifacts that possibly amplified during the dehazing process. The experimental results show that the proposed video dehazing approach is able to well preserve the spatial-temporal coherence, runs sufficiently fast, and also performs favorably compared to the state-of-the-art methods.
更多查看译文
关键词
Video dehazing,Incremental transmission learning,Coherent regularization,Boundary constrained dark channel model
AI 理解论文
溯源树
样例
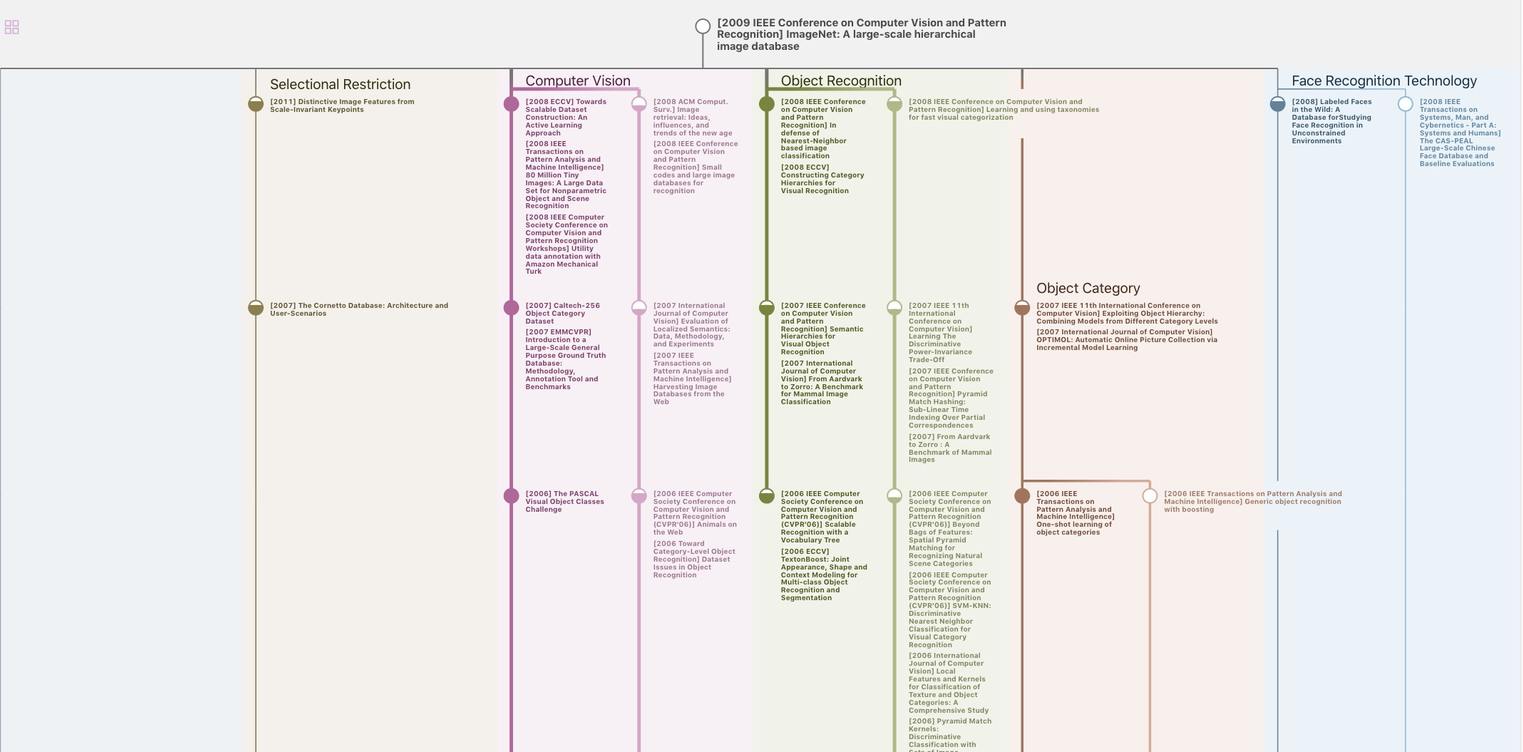
生成溯源树,研究论文发展脉络
Chat Paper
正在生成论文摘要