Forecasting Office Indoor Co2 Concentration Using Machine Learning With A One-Year Dataset
BUILDING AND ENVIRONMENT(2021)
摘要
Modern buildings are expected to fulfill energy efficiency regulations while providing a healthy and comfortable living environment for their occupants. Demand-controlled heating, ventilation, and air conditioning can improve energy efficiency and well-being, especially if changes in indoor environmental factors can be forecast reliably enough. As a first step, this study afforded a baseline for CO2 forecasting evaluation by providing for the public use a comprehensive dataset covering a full year. Secondly, we studied the applicability of four machine learning methods, Ridge regression, Decision Tree, Random Forest, and Multilayer Perceptron, for modeling the future concentration of CO2 in indoors. We evaluated their prediction accuracy within different forecasting and history window time frames and the impact of multiple sensor modalities. All models performed better than the baseline method of predicting the last observed value, and the Decision Tree was found to be almost as accurate as the computationally heavier Random Forest model. When the future forecasting window was longer than a minute, the optimal sensor modalities included occupant activity data from passive infrared sensors in addition to CO2 concentration. Our findings suggest that machine learning can be applied in multimodal time-series data to find a simple, accurate, and resource-efficient forecasting model for proactive control of indoor environments.
更多查看译文
关键词
Carbon dioxide, Modeling, Indoor air quality, Environmental sensor data, Energy efficiency, Well-being
AI 理解论文
溯源树
样例
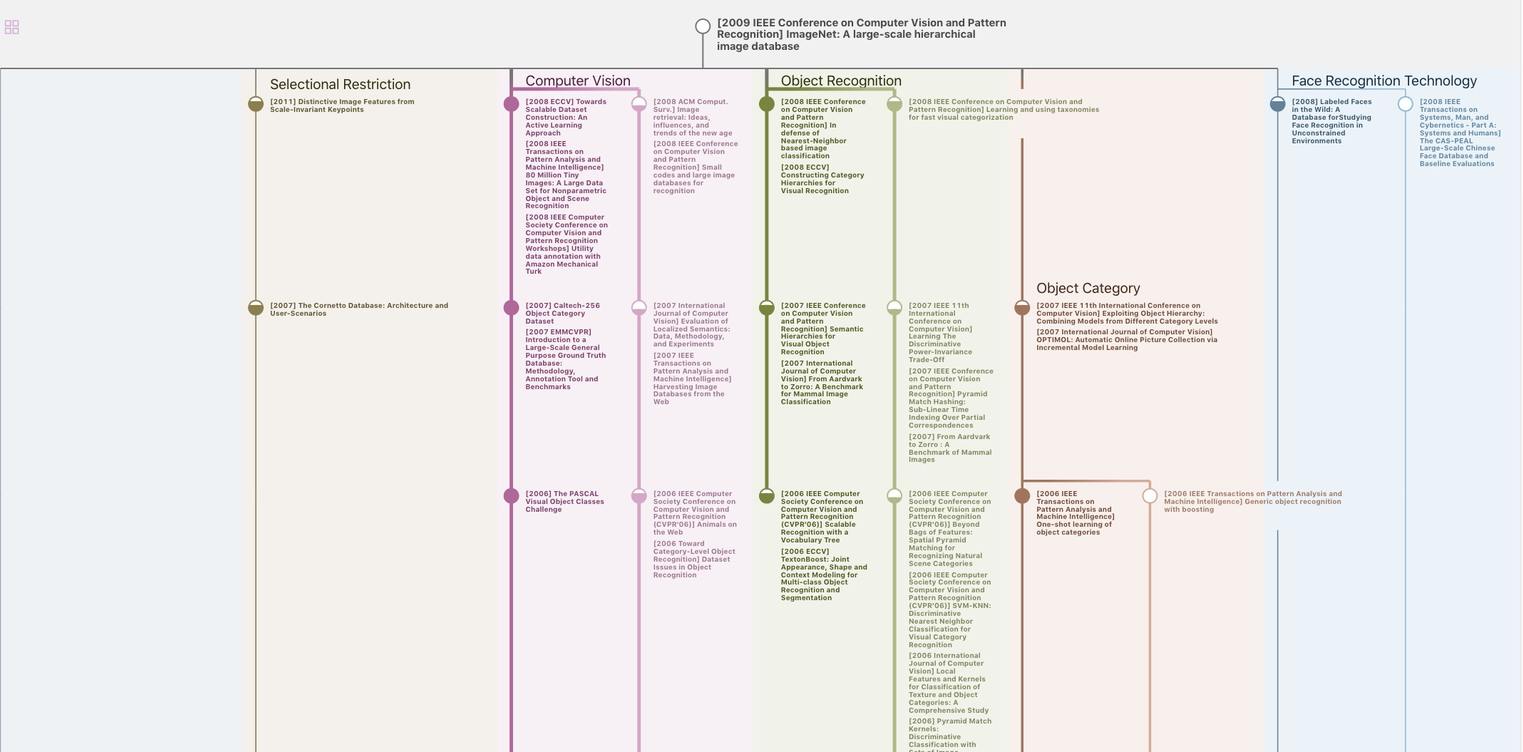
生成溯源树,研究论文发展脉络
Chat Paper
正在生成论文摘要