The Deep 3d Convolutional Multi-Branching Spatial-Temporal-Based Unit Predicting Citywide Traffic Flow
APPLIED SCIENCES-BASEL(2020)
摘要
Recently, for public safety and traffic management, traffic flow prediction is a crucial task. The citywide traffic flow problem is still a big challenge in big cities because of many complex factors. However, to handle some complex factors, e.g., spatial-temporal and some external factors in the intelligent traffic flow forecasting problem, spatial-temporal data for urban applications (i.e., travel time estimation, trajectory planning, taxi demand, traffic congestion, and the regional rainfall) is inherently stochastic and unpredictable. In this paper, we proposed a deep learning-based novel model called "multi-branching spatial-temporal attention-based long-short term memory residual unit (MBSTALRU)" for the citywide traffic flow from lower-level layers to high-level layers, simultaneously. In our work, initially, we have modeled the traffic flow with spatial correlations multiple 3D volume layers and propose the novel multi-branching scheme to control the spatial-temporal features. Our approach is useful for exploring temporal dependencies through the 3D convolutional neural network (CNN) multiple branches, which aim to merge the spatial-temporal characteristics of historical data with three-time intervals, namely closeness, daily, and weekly, and we have embedded features by attention-based long-short term memory (LSTM). Then, we capture the correlation between traffic inflow and outflow with residual layers units. In the end, we merge the external factors dynamically to predict citywide traffic flow simultaneously. The simulation results have been performed on two real-world datasets, BJTaxi and NYCBike, which show better performance and effectiveness of the proposed method than previous state-of-the-art models.
更多查看译文
关键词
citywide traffic flow, multi-branching spatial-temporal, attention-based LSTM
AI 理解论文
溯源树
样例
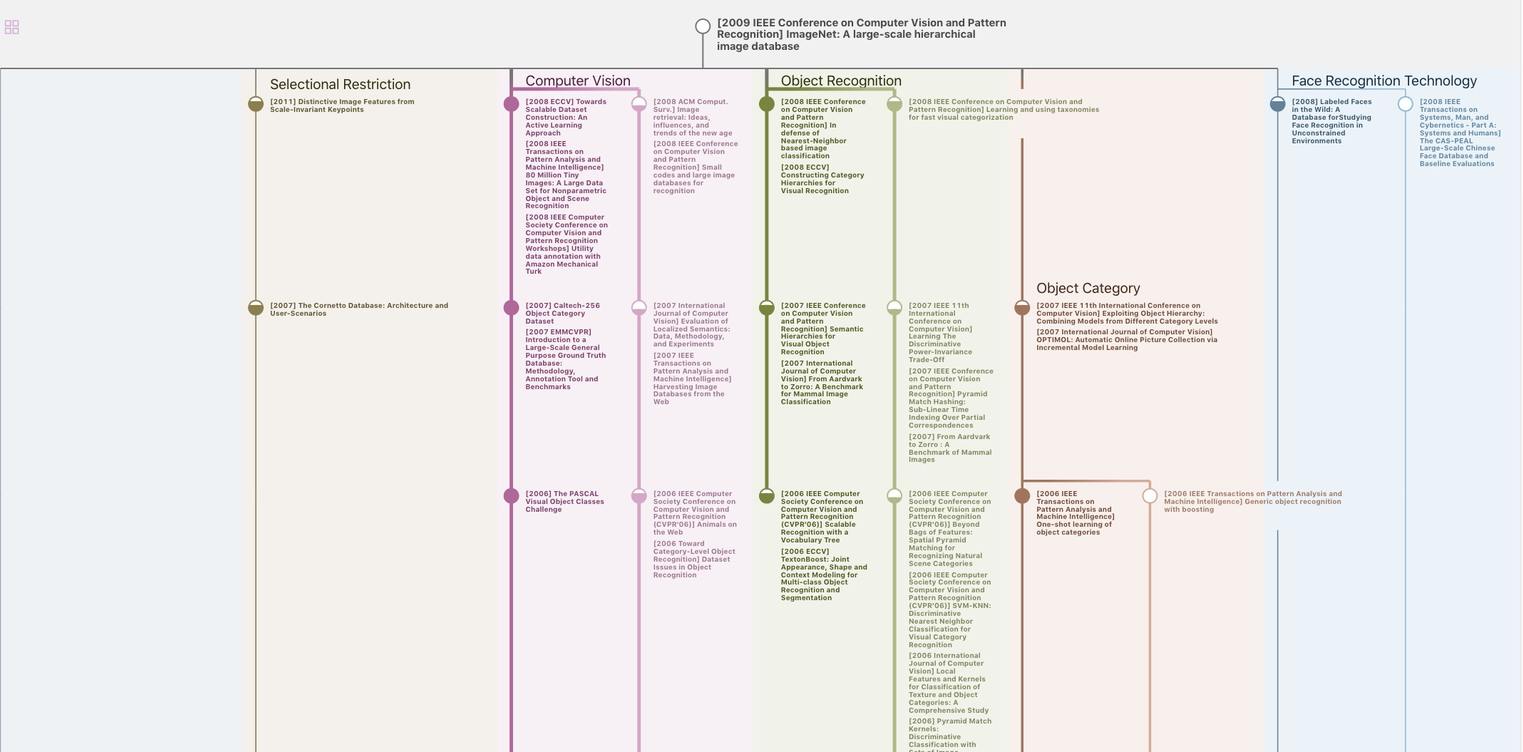
生成溯源树,研究论文发展脉络
Chat Paper
正在生成论文摘要