Adaptive Weighted Maximum Margin Criterion
2020 IEEE 3rd International Conference on Information Systems and Computer Aided Education (ICISCAE)(2020)
摘要
Feature extraction is an image processing method widely used in machine learning, playing a significant role in both recognition and classification tasks. In contrast with unsupervised feature learning methods, supervised feature learning methods supported by labels achieve more valuable discriminative information. However, this inevitably draws attention to the small sample size (SSS) problem, which leads to singular matrices and impacts on the accuracy of results. In this paper, we propose a novel method based on Maximum Margin Criterion (MMC) with adaptive weighting mechanism to preserve features of good discriminant performance while reducing feature dimensionality. There are two major novelties of the proposed method: (1) the MMC model is utilized to resolve the irreversibility of matrices by maximizing the difference of the between-class and within-class scatter matrix when the feature dimension outnumbers the number of samples; (2) the adaptive weighting mechanism which can automatically find the optimal weight is designed as the balancer to adjust the within-class scatter and the between-class scatter. We validate our new approach on three popular image datasets. The experimental results indicate that the proposed method shows superiority in the following classification tasks, which performs favorably against classical methods in accuracy and calculation cost.
更多查看译文
关键词
Adaptive weighting,SSS,MMC,Supervised learning,Feature extraction
AI 理解论文
溯源树
样例
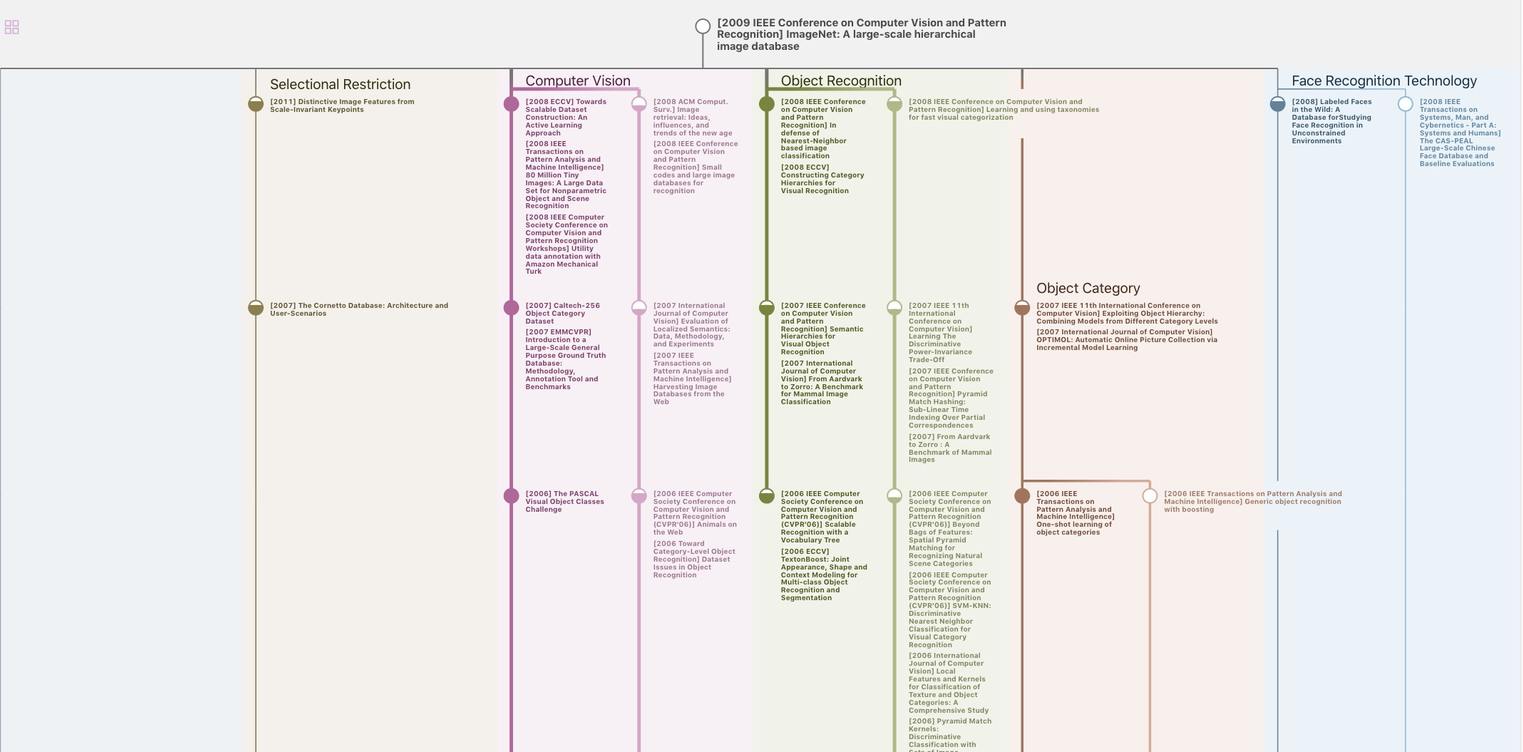
生成溯源树,研究论文发展脉络
Chat Paper
正在生成论文摘要