Regional Attention Network (RAN) for Head Pose and Fine-Grained Gesture Recognition
IEEE Transactions on Affective Computing(2023)
摘要
Affect is often expressed via non-verbal body language such as actions/gestures, which are vital indicators for human behaviors. Recent studies on recognition of fine-grained actions/gestures in monocular images have mainly focused on modeling spatial configuration of body parts representing body pose, human-objects interactions and variations in local appearance. The results show that this is a brittle approach since it relies on accurate body parts/objects detection. In this work, we argue that there exist local discriminative semantic regions, whose “informativeness” can be evaluated by the attention mechanism for inferring fine-grained gestures/actions. To this end, we propose a novel end-to-end
regional attention network (RAN)
, which is a fully convolutional neural network (CNN) to combine multiple contextual regions through attention mechanism, focusing on parts of the images that are most relevant to a given task. Our regions consist of one or more consecutive cells and are adapted from the strategies used in computing HOG (Histogram of Oriented Gradient) descriptor. The model is extensively evaluated on ten datasets belonging to 3 different scenarios: 1) head pose recognition, 2) drivers state recognition, and 3) human action and facial expression recognition. The proposed approach outperforms the state-of-the-art by a considerable margin in different metrics.
更多查看译文
关键词
Gesture recognition,head pose recognition,facial expressions recognition,attention mechanism,convolutional neural network,fine-grained gesture recognition,computer vision,human-object interaction,regional attention network
AI 理解论文
溯源树
样例
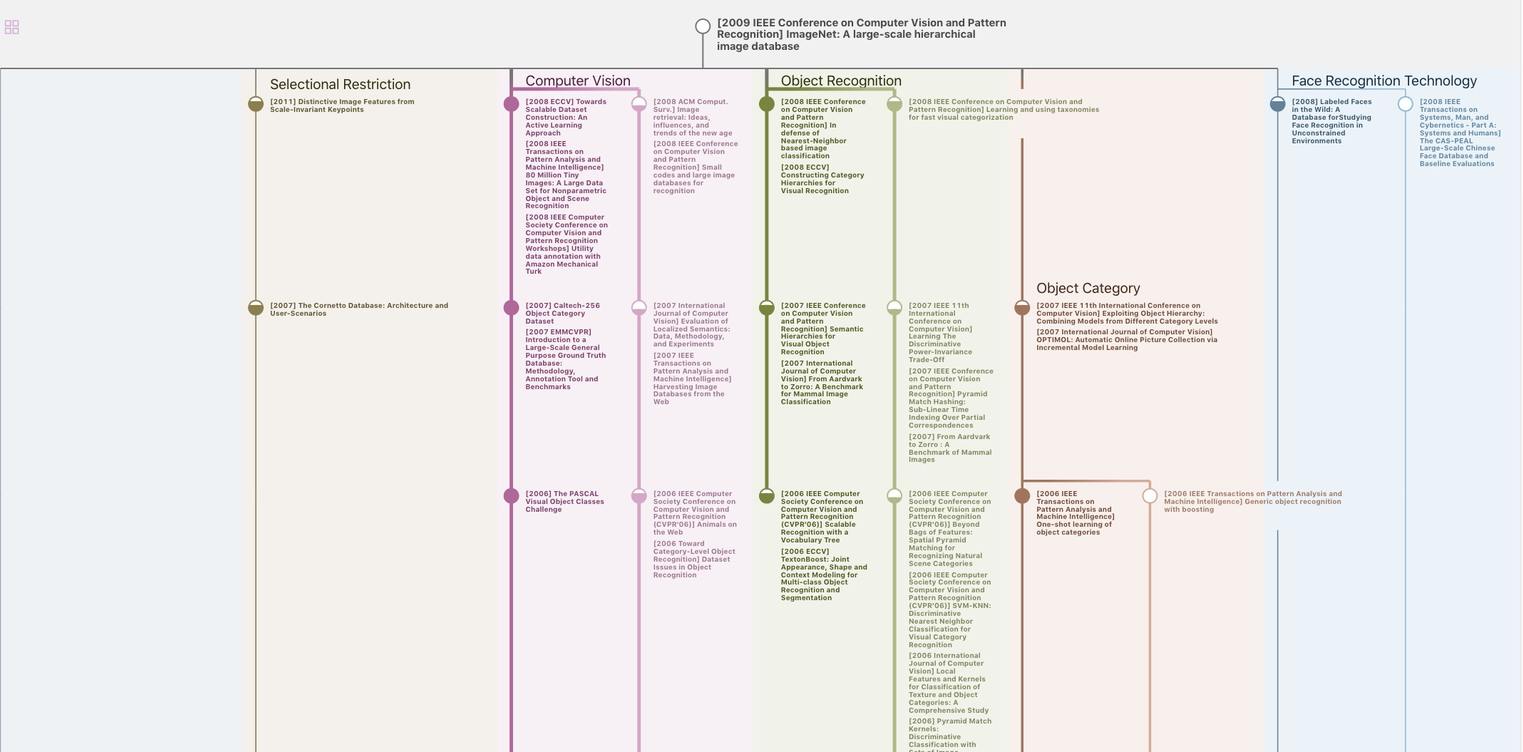
生成溯源树,研究论文发展脉络
Chat Paper
正在生成论文摘要