A Comparative Study Of Transfer Learning Approaches For Video Anomaly Detection
INTERNATIONAL JOURNAL OF PATTERN RECOGNITION AND ARTIFICIAL INTELLIGENCE(2021)
摘要
Recent research has shown that features obtained from pretrained Convolutional Neural Network (CNN) models can be promptly applied to a variety of problems they were not originally designed to solve. This concept, often referred to as Transfer Learning (TL), is a common practice when labeled data is limited. In some fields, such as video anomaly detection, TL is still an underexplored subject in the sense that it is not clear whether the architecture of the pretrained CNN model impacts on the video anomaly detection performance. In order to clarify this issue, we perform an extensive benchmark using 12 different pretrained CNN models on ImageNet as feature extractors and apply the features obtained to seven video anomaly detection benchmark datasets. This work presents some interesting findings about video anomaly detection using TL. The highlights of our findings were revealed by our experiments, which have shown that a simple classification process using One-Class Support Vector Machines yields similar results to state-of-the-art models. Moreover, a statistical analysis suggests that architectural differences are negligible when choosing a pretrained model for video anomaly detection, since all models presented similar performance. At last, we present an in-depth visual analysis of the Avenue dataset, and reveal several aspects that may be limiting the performance of state-of-the-art video anomaly detection methods.
更多查看译文
关键词
Transfer learning, anomaly detection, dataset analysis, deep learning
AI 理解论文
溯源树
样例
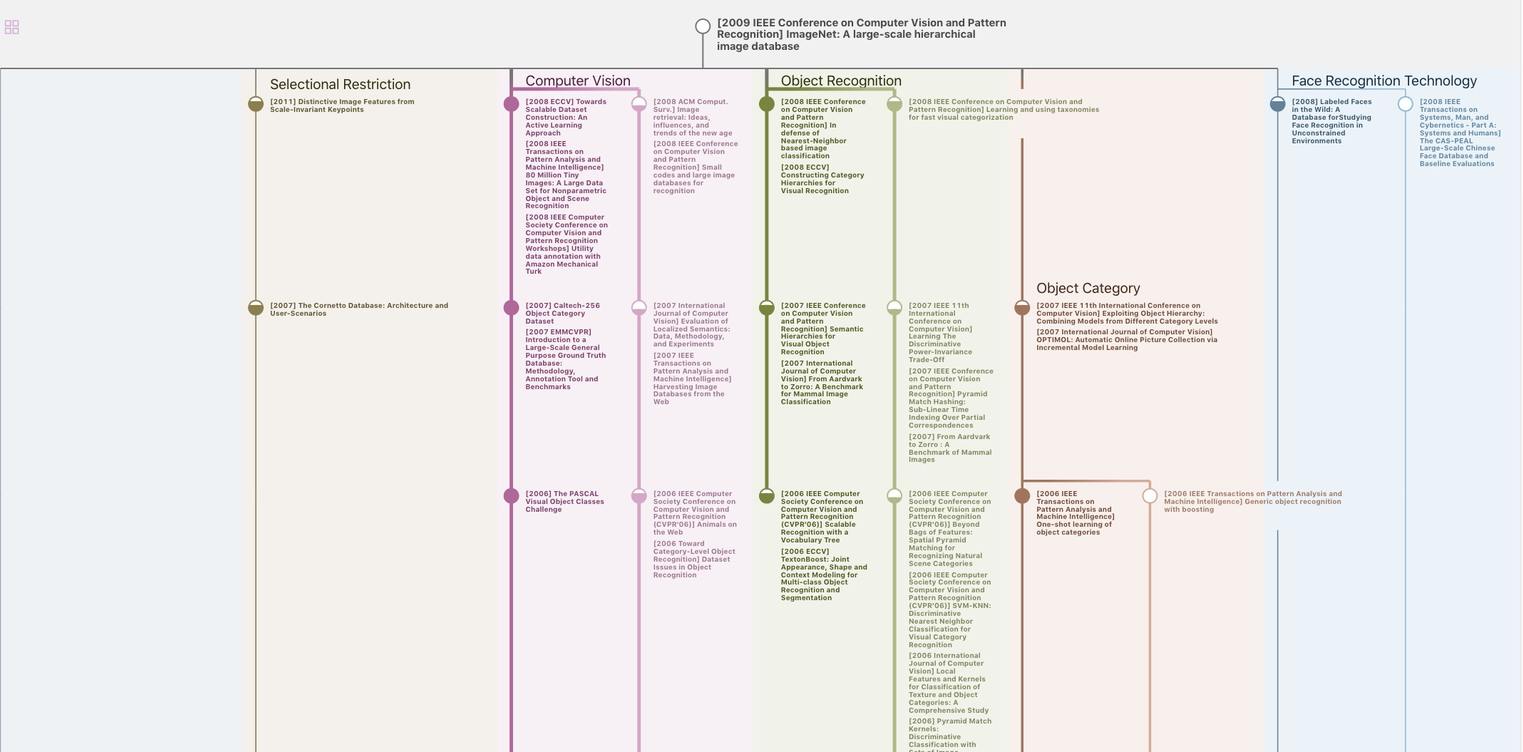
生成溯源树,研究论文发展脉络
Chat Paper
正在生成论文摘要