Parametric Shape Estimation of Human Body Under Wide Clothing
IEEE TRANSACTIONS ON MULTIMEDIA(2021)
摘要
The shape of the human body plays an important role in many applications, such as those involving personal healthcare and virtual clothing try-ons. However, accurate body shape measurements typically require the user to be wearing a minimal amount of clothing, which is not practical in many situations. To resolve this issue using deep learning techniques, we need a paired dataset of ground-truth naked human body shapes and their corresponding color images with clothes. As it is practically impossible to collect enough of this kind of data from real-world environments to train a deep neural network, in this paper, we present the Synthetic dataset of Human Avatars under wiDE gaRment (SHADER). The SHADER dataset consists of 300,000 paired ground-truth naked and dressed images of 1,500 synthetic humans with different body shapes, poses, garments, skin tones, and backgrounds. To take full advantage of SHADER, we propose a novel silhouette confidence measure and show that our silhouette confidence prediction network can help improve the performance of state-of-the-art shape estimation networks for human bodies under clothing. The experimental results demonstrate the effectiveness of the proposed approach. The code and dataset are available at https://github.com/YCL92/SHADER.
更多查看译文
关键词
Shape, Clothing, Three-dimensional displays, Two dimensional displays, Biological system modeling, Pose estimation, Silhouette confidence, convolutional neural network, human shape estimation, synthetic dataset
AI 理解论文
溯源树
样例
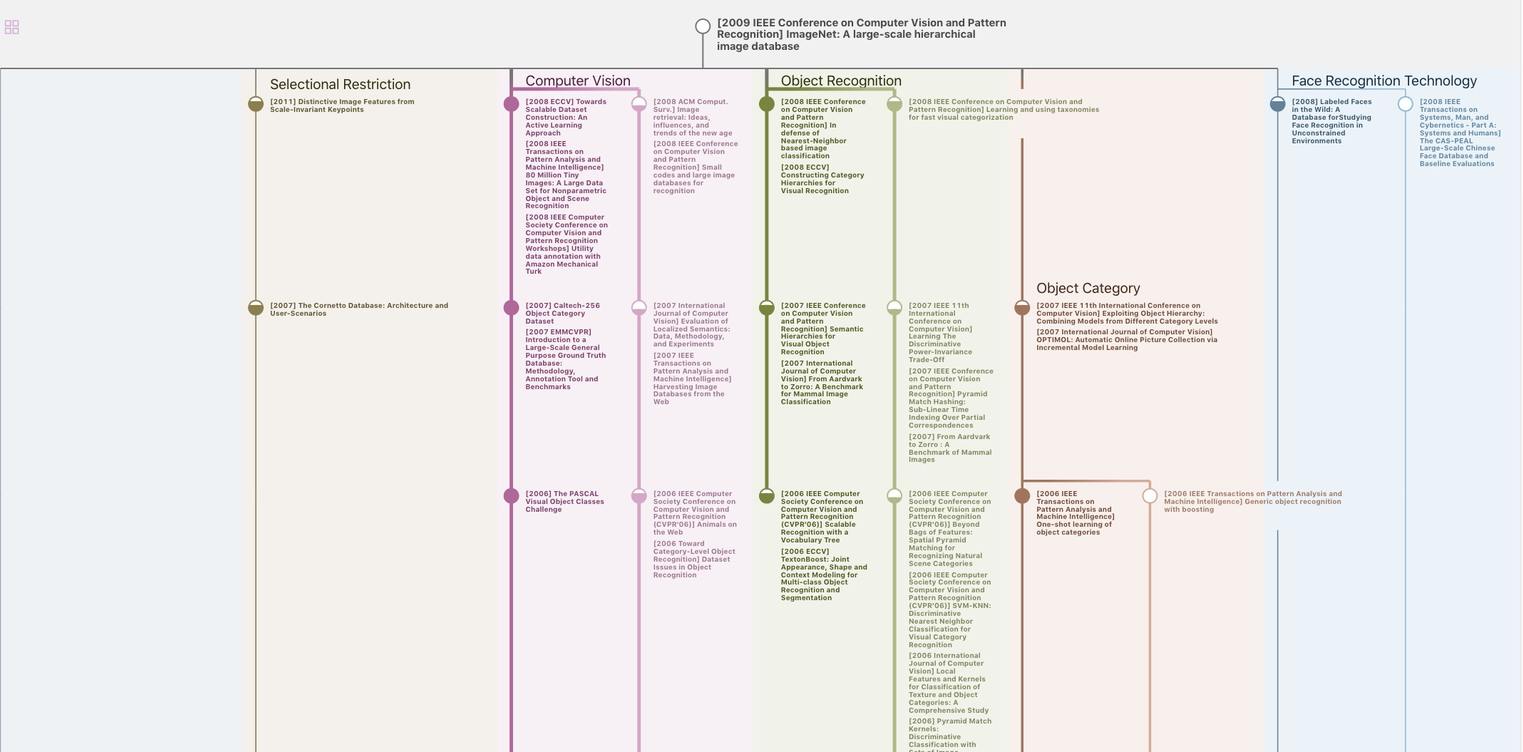
生成溯源树,研究论文发展脉络
Chat Paper
正在生成论文摘要