Rank Detection Thresholds For Hankel Or Toeplitz Data Matrices
28TH EUROPEAN SIGNAL PROCESSING CONFERENCE (EUSIPCO 2020)(2021)
摘要
In Principal Component Analysis (PCA), the dimension of the signal subspace is detected by counting the number of eigenvalues of a covariance matrix that are above a threshold. Random matrix theory provides accurate estimates for this threshold if the underlying data matrix has independent identically distributed columns. However, in time series analysis, the underlying data matrix has a Hankel or Toeplitz structure, and the columns are not independent. Using an empirical approach, we observe that the largest eigenvalue is fitted well by a Generalized Extreme Value (GEV) distribution, and we obtain accurate estimates for the thresholds to be used in a sequential rank detection test. In contrast to AIC or MDL, this provides a parameter that controls the probability of false alarm. Also a lower bound is presented for the rank detection rate of threshold-based detection for rank-1 problems.
更多查看译文
关键词
PCA, structuredWishart matrix, rank detection, Generalized Extreme Value
AI 理解论文
溯源树
样例
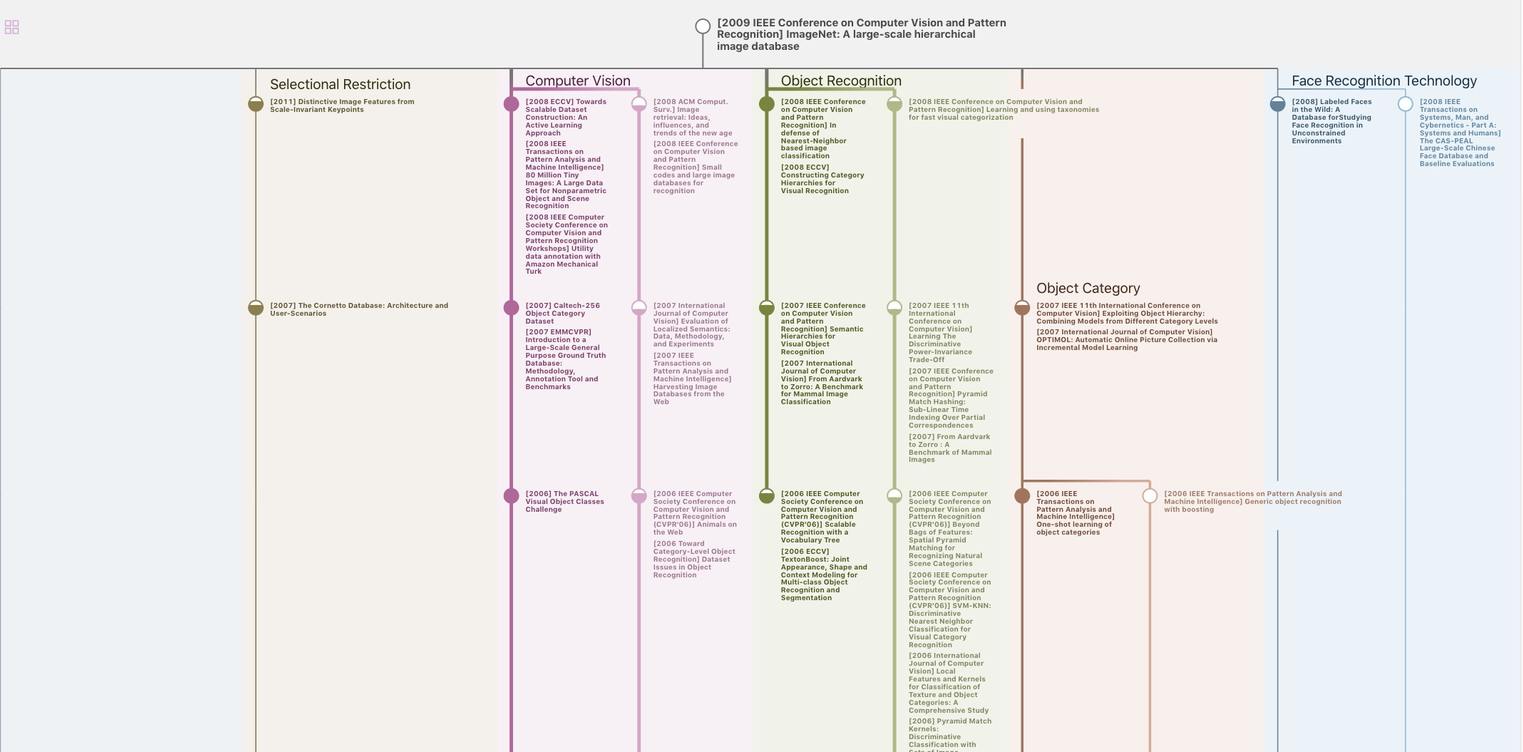
生成溯源树,研究论文发展脉络
Chat Paper
正在生成论文摘要