Hyperspectral Unmixing Using Spectral Library Sparse Scaling and Guided Filter
IEEE GEOSCIENCE AND REMOTE SENSING LETTERS(2022)
摘要
Sparse regression based on spectral libraries has become a promising alternative for addressing the hyperspectral unmixing problem. However, the actual endmembers of a scene are usually inconsistent with the corresponding spectral signatures in the spectral library, which largely limits the performance of sparse regression approaches. In this letter, a new sparse regression algorithm considering spectral library mismatch is proposed, which allows the spectral signatures in the spectral library to independently scale in each band and regularizes the differential of the scaling factors to be sparse. Moreover, a guided filter (GF)-based regularizer is introduced to explore the spatial-contextual information. The spectral library sparse scaling and GF constraints are combined to mitigate the impact of the spectral library mismatch. Experimental results on both synthetic and real data show that the proposed algorithm outperforms other methods that address the spectral library mismatch problem.
更多查看译文
关键词
Libraries, Hyperspectral imaging, TV, Image edge detection, Collaboration, Optimization, Dictionary mismatch, guided filter (GF), hyperspectral unmixing, spectral library sparse scaling, spectral variability
AI 理解论文
溯源树
样例
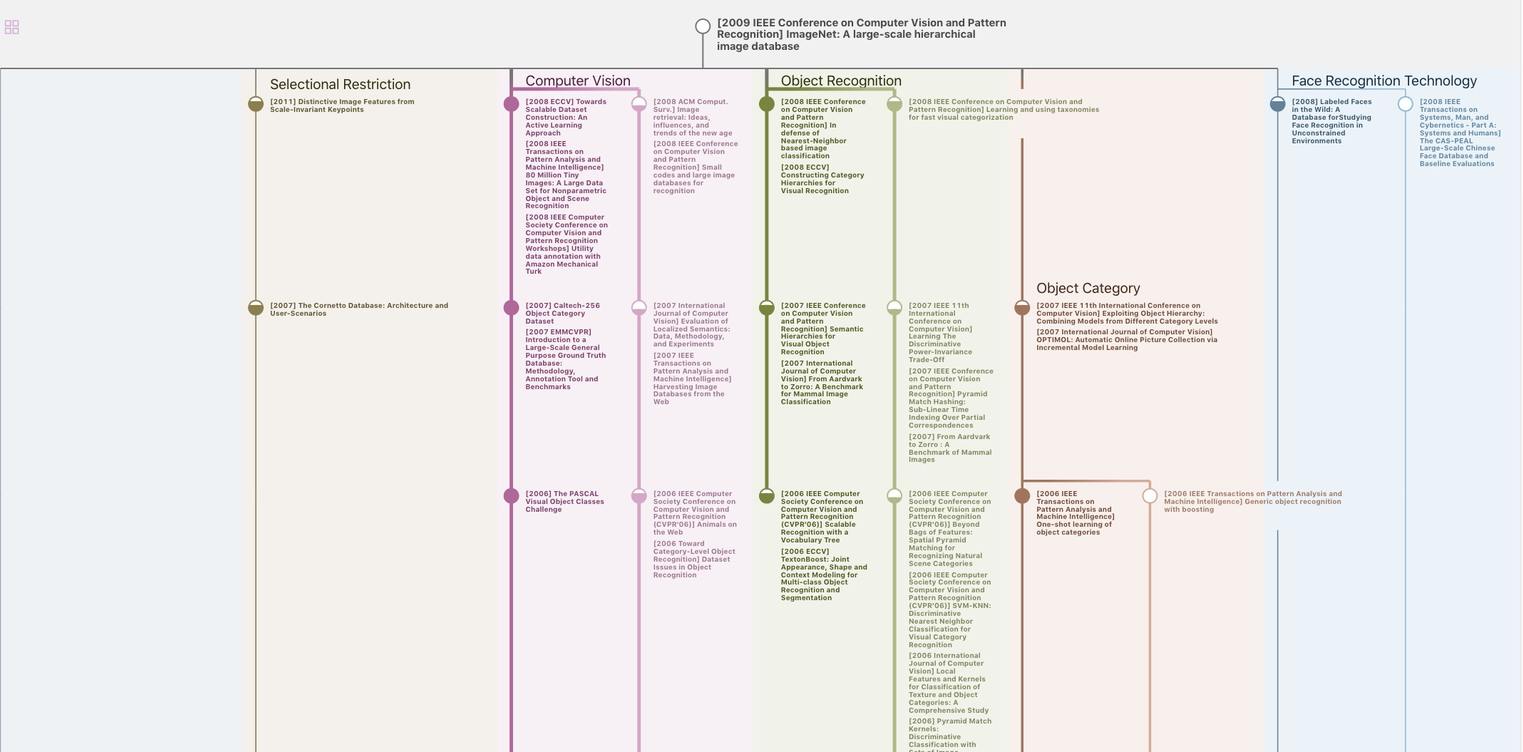
生成溯源树,研究论文发展脉络
Chat Paper
正在生成论文摘要