Analog Circuits to Accelerate the Relaxation Process in the Equilibrium Propagation Algorithm
ISCAS(2020)
摘要
Equilibrium Propagation (EP) is a novel biologically plausible algorithm for training deep neural networks. However, discrete time implementations of EP could not exploit the full potential of its proposed framework, because the relaxation step is slow on digital architectures. Here, we propose an analog circuit implementation for the relaxation process to accelerate its convergence. In this implementation, the optimization process is executed based on the continuous-time dynamics of EP. Our circuit is validated using a Continuous Hopfield Network prototype emulating the XOR function. This implementation improved the convergence time of EP by a factor of 250 compared to its Python counterpart. As this implementation is scalable to learn more complex functions, it has the potential to be applied on higher-dimensionality datasets.
更多查看译文
关键词
Free Phase, Equilibrium Propagation, Relaxation Process, Analog Implementation, Continuous Hopfield Model
AI 理解论文
溯源树
样例
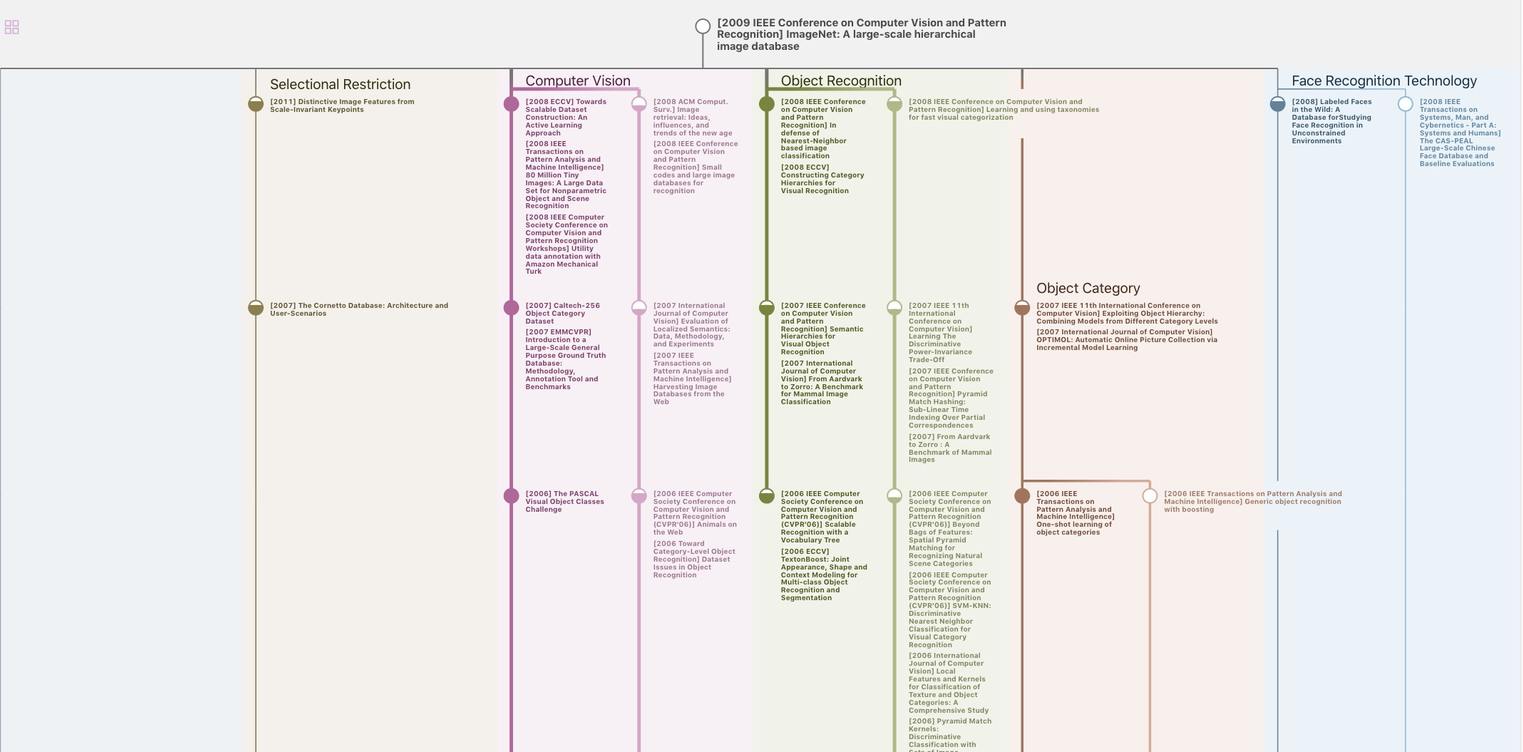
生成溯源树,研究论文发展脉络
Chat Paper
正在生成论文摘要