Gan-Based Classifier Protection Against Adversarial Attacks
JOURNAL OF INTELLIGENT & FUZZY SYSTEMS(2020)
摘要
In recent years, deep neural networks have made significant progress in image classification, object detection and face recognition. However, they still have the problem of misclassification when facing adversarial examples. In order to address security issue and improve the robustness of the neural network, we propose a novel defense network based on generative adversarial network (GAN). The distribution of clean - and adversarial examples are matched to solve the mentioned problem. This guides the network to remove invisible noise accurately, and restore the adversarial example to a clean example to achieve the effect of defense. In addition, in order to maintain the classification accuracy of clean examples and improve the fidelity of neural network, we input clean examples into proposed network for denoising. Our method can effectively remove the noise of the adversarial examples, so that the denoised adversarial examples can be correctly classified. In this paper, extensive experiments are conducted on five benchmark datasets, namely MNIST, Fashion-MNIST, CIFAR10, CIFAR100 and ImageNet. Moreover, six mainstream attack methods are adopted to test the robustness of our defense method including FGSM, PGD, MIM, JSMA, CW and Deep-Fool. Results show that our method has strong defensive capabilities against the tested attack methods, which confirms the effectiveness of the proposed method.
更多查看译文
关键词
Deep neural network, generative adversarial network, adversarial example, defense
AI 理解论文
溯源树
样例
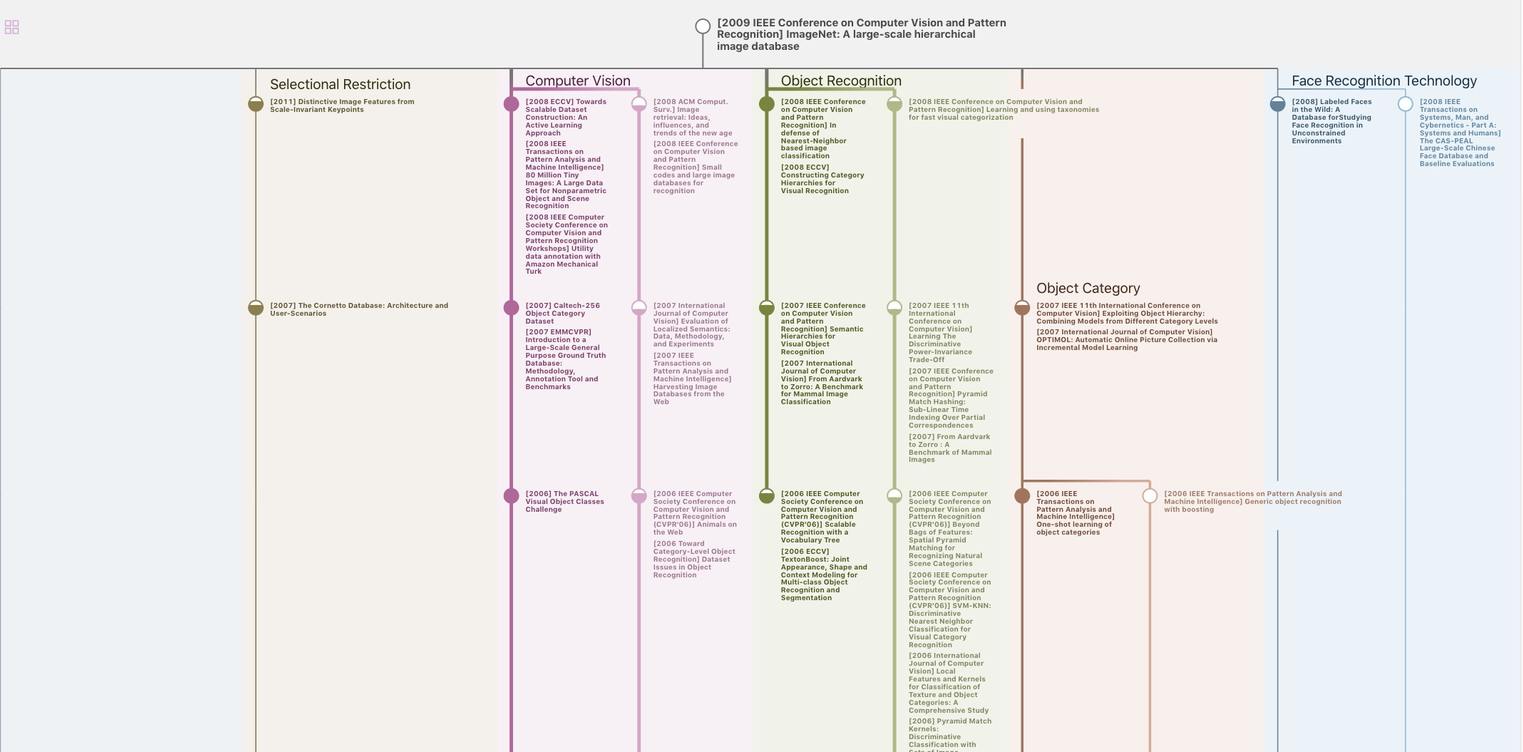
生成溯源树,研究论文发展脉络
Chat Paper
正在生成论文摘要