Edge Intelligence Empowered Urban Traffic Monitoring: A Network Tomography Perspective
IEEE Transactions on Intelligent Transportation Systems(2021)
摘要
Efficient urban traffic monitoring is a key enabler for intelligent planning and management of modern cities. Network tomography can monitor the urban traffic with a comparably small number of traffic detectors like cameras, and has become an appealing technique for urban traffic management. However, previous work on network tomography based traffic monitoring focuses primarily on developing estimators using the given end-to-end travel time measurements, while the design of data collection for efficiently distributed collecting and processing the raw monitoring videos to such measurements is often neglected. We fill this gap by exploring the vision of edge intelligence for optimal urban traffic monitoring, and tackle the following two problems in regard of limited telecommunications resources: 1) when the total number of monitoring videos that are successfully processed into the end-to-end travel time measurements is pre-bounded, we employ a Fisher Information Matrix (FIM) to help determine the best quota scheme for the monitoring videos that each traffic detector need to generate and 2) when the centralised processing of monitoring videos alone is insufficient, we make use of the computation capabilities from these edge devices, i.e., traffic detectors, and employ a multi-agent reinforcement learning approach to help them conduct intelligent computation offloading individually. Extensive simulations demonstrate that our proposed scheme effectively reduces the estimation error of network tomography compared to common approaches with either uniform or random strategy.
更多查看译文
关键词
Traffic monitoring,network tomography,fisher information matrix,reinforcement learning,edge intelligence
AI 理解论文
溯源树
样例
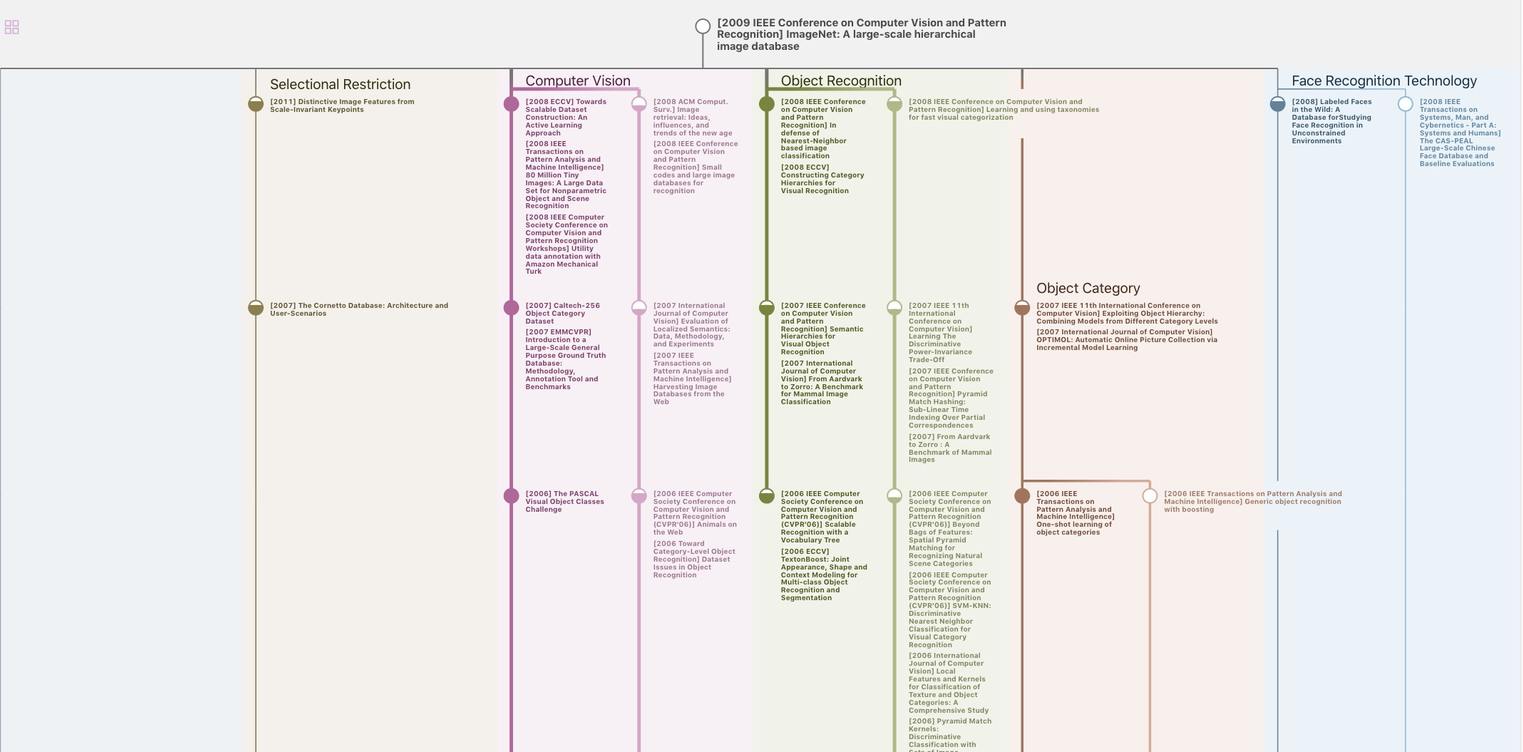
生成溯源树,研究论文发展脉络
Chat Paper
正在生成论文摘要