Harden Deep Convolutional Classifiers via K-Means Reconstruction
IEEE ACCESS(2020)
摘要
Adversarial examples are carefully perturbed input examples that aim to mislead the deep neural network models into producing unexpected outputs. In this paper, we employ a K-means clustering algorithm as a pre-processing method to defend against adversarial examples. Specifically, we reconstruct adversarial examples according to their cluster assignments in pixel level to reduce the impact of the injected perturbation. Our approach does not rely on any neural network architectures and can also work with existing pre-processing defenses to provide better protection for modern classifiers. Comprehensive comparison and evaluation have been conducted to investigate our proposal, where the models protected by the proposed defense show substantial robustness to strong adversarial attacks. As a by-product of our exploration of ensemble defense, we identify that the order of defense methods has a crucial impact on the final performance. Additionally, the limitation of K-means reconstruction and the impact of the number of clusters have also been studied to provide an in-deep understanding of pre-processing defenses.
更多查看译文
关键词
Perturbation methods,Clustering algorithms,Image reconstruction,Robustness,Training,Neural networks,Machine learning,Adversarial robustness,pre-processing defense,K-means clustering,deep learning
AI 理解论文
溯源树
样例
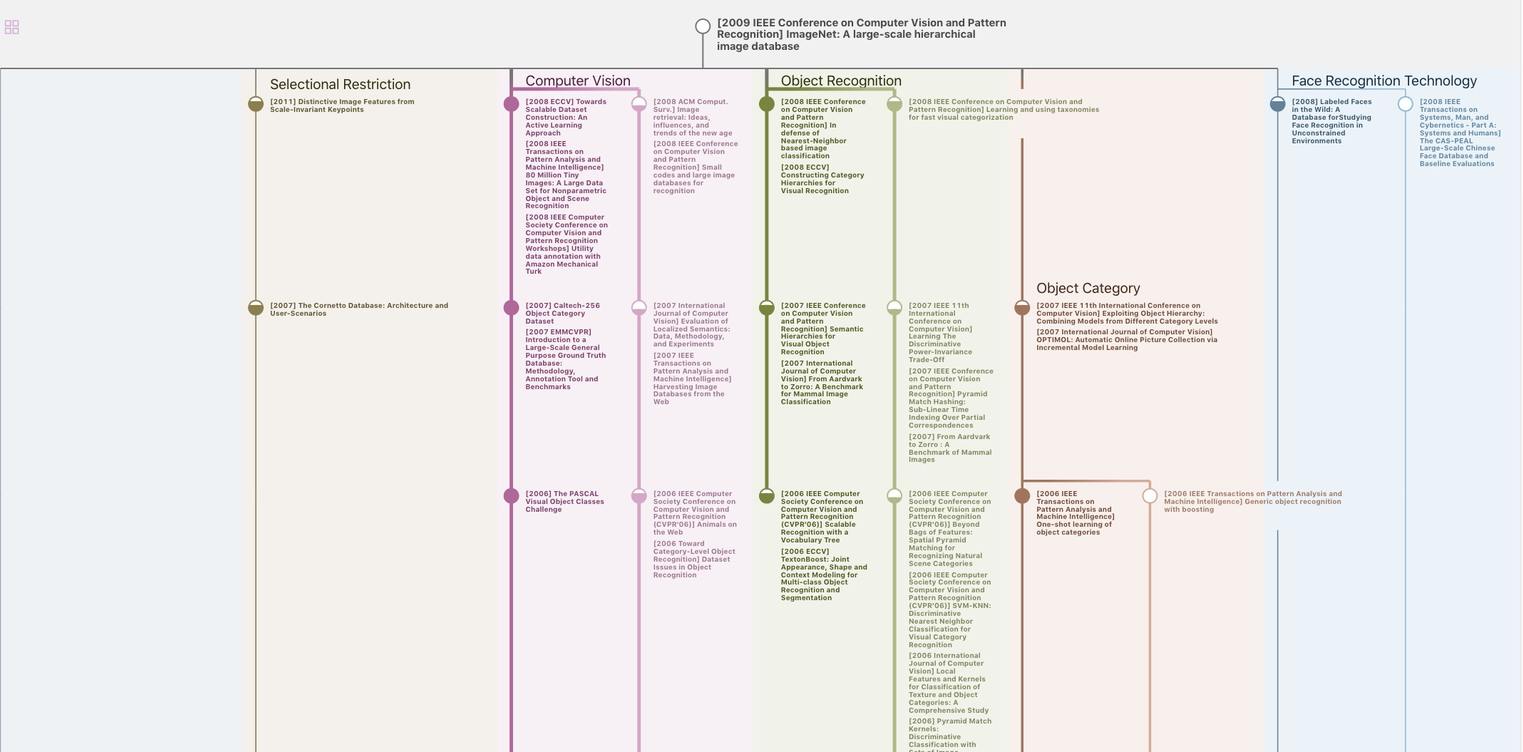
生成溯源树,研究论文发展脉络
Chat Paper
正在生成论文摘要