Link Inference via Heterogeneous Multi-view Graph Neural Networks
database systems for advanced applications(2020)
摘要
Graph Neural Network (GNN) has been attaching great attention along with its successful industry applications, such as social network, recommendation system and so on. Most existing GNN algorithms for link inference tasks mainly concentrate on homogeneous network where single typed nodes and edges are considered. Besides, they are transductive, incapable of handling unseen data, and are difficult to generalize to big graph data. In this paper, we introduce a new idea, i.e. Heterogeneous Multi-view Graph Neural Network (HMGNN), to remedy these problems. A more complex and unstudied heterogeneous network structure where multiple node and edge types co-exist, and each of them also contains specific attributes, is learned in this framework. The proposed HMGNN is end-to-end and two stages are designed: i) The first stage extends the widely-used GraphSAGE model to the studied heterogeneous scenario to generate the vector embedded representations for each type of nodes. ii) The second stage develops a novel and inductive subspace-based strategy for link inference by aggregating multi-typed node and edge feature views. Comprehensive experiments on large-scale spam detection and link prediction applications clearly verify the effectiveness of our model.
更多查看译文
关键词
link inference,graph neural networks,neural networks,heterogeneous,multi-view
AI 理解论文
溯源树
样例
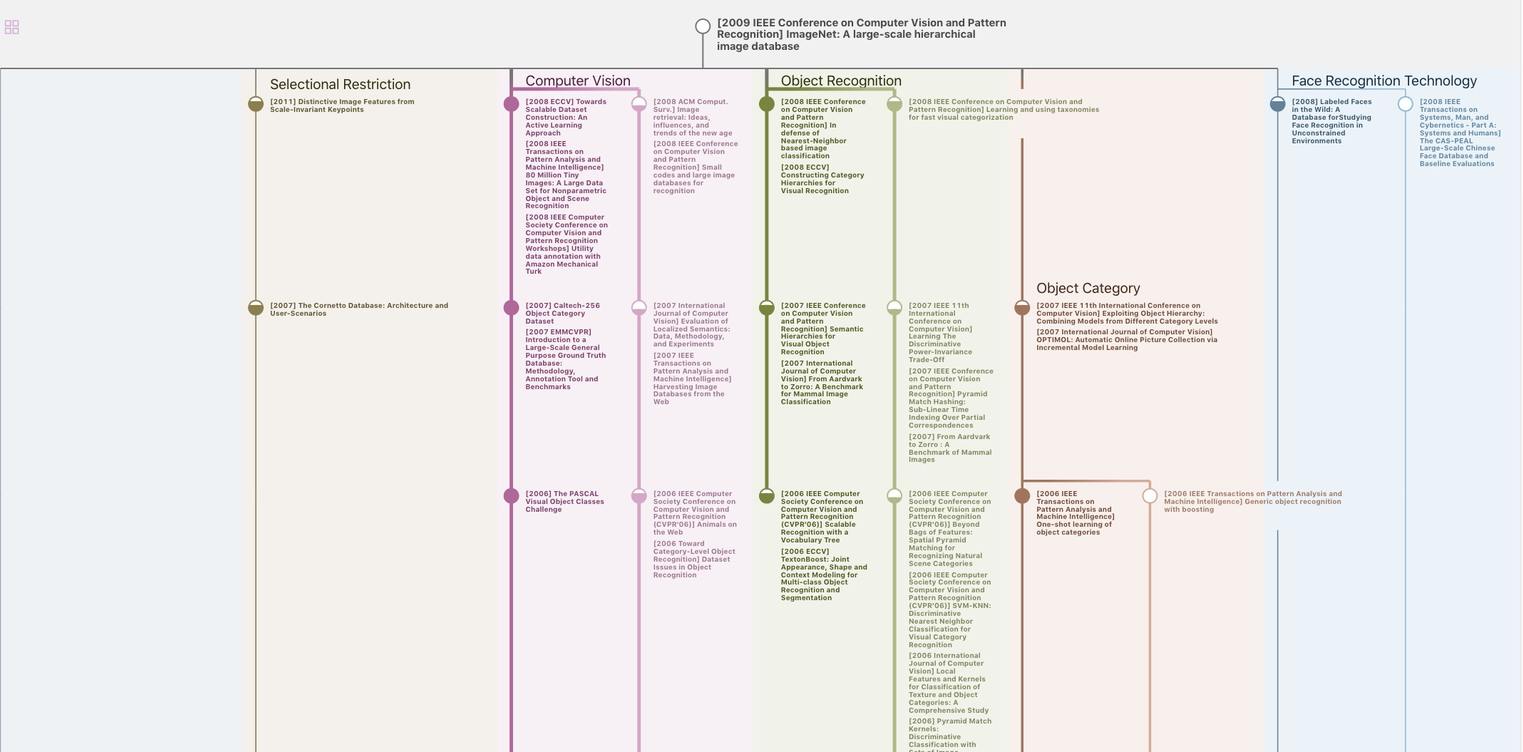
生成溯源树,研究论文发展脉络
Chat Paper
正在生成论文摘要