Optimizing microtask assignment on crowdsourcing platforms using Markov chain Monte Carlo
decision support systems(2020)
摘要
Abstract Microtasking is a type of crowdsourcing, denoting the act of breaking a job into several tasks and allocating them to multiple workers to complete. The assignment of tasks to workers is a complex decision-making process, particularly when considering budget and quality constraints. While there is a growing body of knowledge on the development of task assignment algorithms, the current algorithms suffer from shortcomings including: after-worker quality estimation, meaning that workers need to complete all tasks after which point their quality can be estimated; and one-off quality estimation method which estimates workers\u0027 quality only at the start of microtasking using a set of pre-defined quality-control tasks. To address these shortcomings, we propose a Markov Chain Monte Carlo–based task assignment approach known as MCMC-TA which provides iterative estimations of workers\u0027 quality and dynamic task assignment. Specifically, we apply Gaussian mixture model (GMM) to estimate workers\u0027 quality and Markov Chain Monte Carlo to shortlist workers for task assignment. We use Google Fact Evaluation dataset to measure the performance of MCMC-TA and compare it against the state-of-the-art algorithms in terms of AUC and F-Score. The results show that the proposed MCMC-TA algorithm not only outperforms the rival algorithms, but also offers a spammer-resistant result that maximizes the learning of workers\u0027 quality with minimal budget.
更多查看译文
关键词
Crowdsourcing, Task assignment, Markov chain, Crowd labeling, Quality estimation
AI 理解论文
溯源树
样例
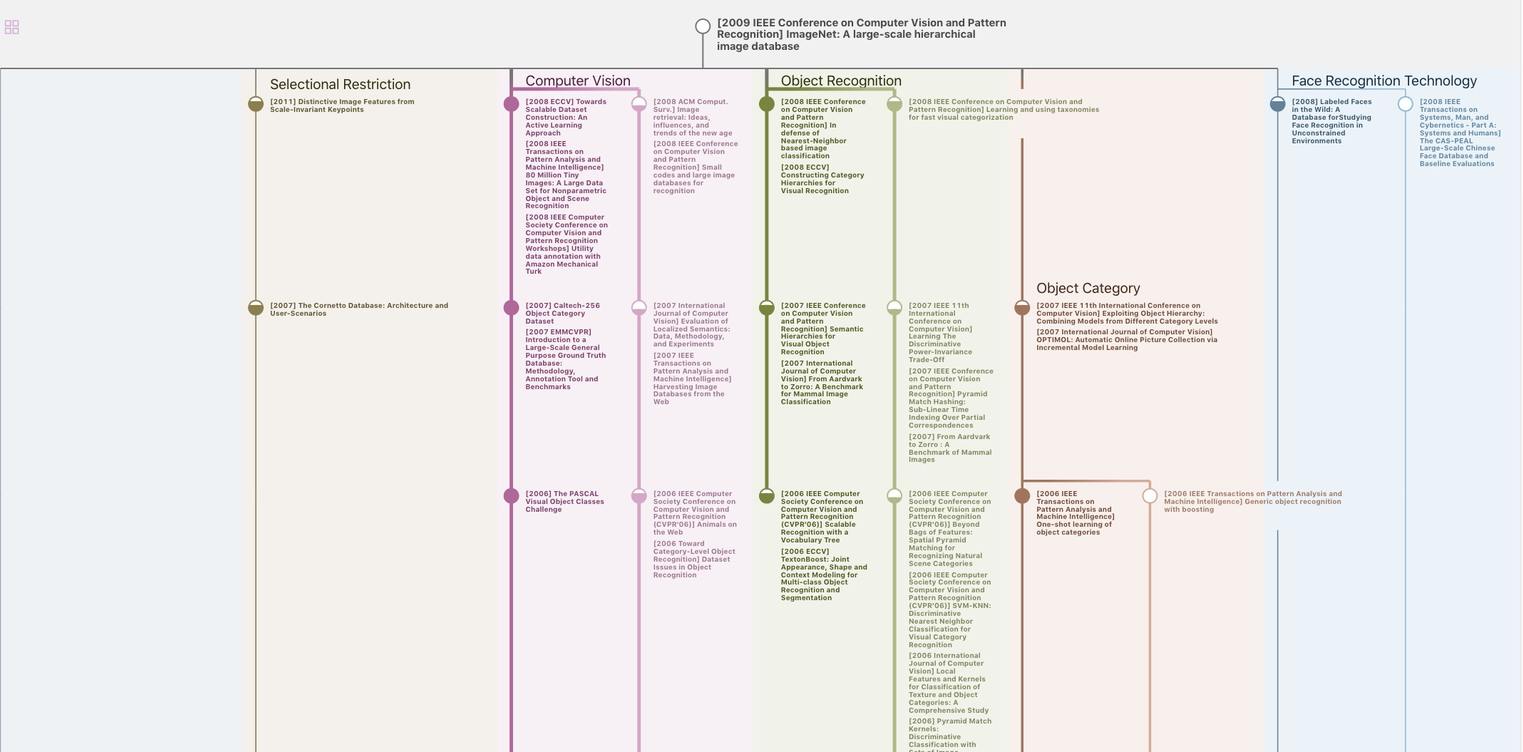
生成溯源树,研究论文发展脉络
Chat Paper
正在生成论文摘要