Two multivariate online change detection models
JOURNAL OF APPLIED STATISTICS(2022)
摘要
Online change point detection methods monitor changes in the distribution of a data stream. This article discusses two non-parametric online change detection methods based on the energy statistics and Mahalanobis depth. To apply the energy statistic, we use sliding-window algorithm with efficient training and updating procedures. For Mahalanobis depth, we propose an algorithm to train the threshold with desired protective ability against false alarms and discuss factors that have an influence on the threshold. Numerical studies evaluate and compare the performance of the proposed models with three existing methods to detect changes in the mean and variability of a data stream. The methods are applied to detecting changes in the flowing volume of the Mississippi River.
更多查看译文
关键词
Online change detection, nonparametric, sliding-window algorithm, energy statistics, depth model
AI 理解论文
溯源树
样例
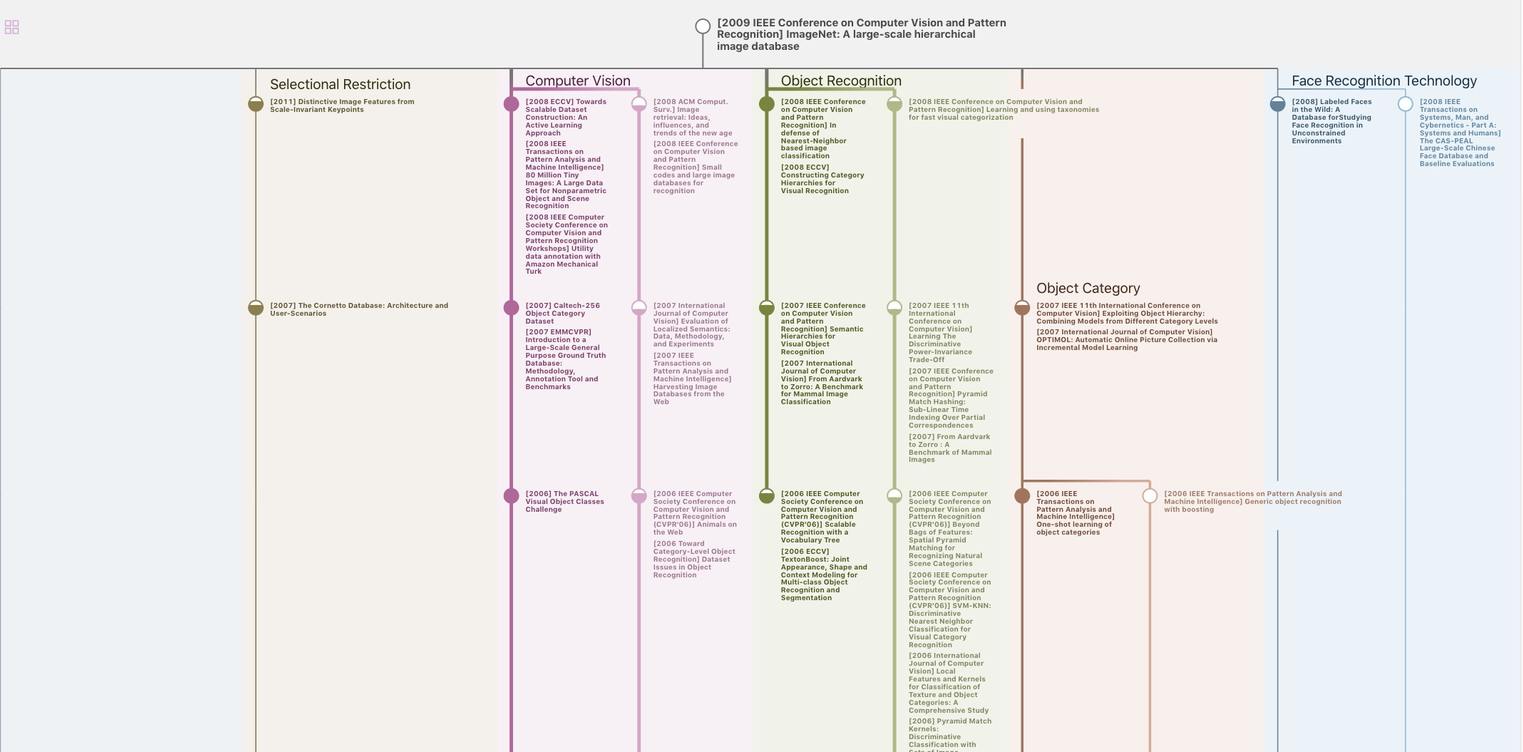
生成溯源树,研究论文发展脉络
Chat Paper
正在生成论文摘要