Fresh Tea Shoot Maturity Estimation Via Multispectral Imaging And Deep Label Distribution Learning
IEICE TRANSACTIONS ON INFORMATION AND SYSTEMS(2020)
摘要
Fresh Tea Shoot Maturity Estimation (FTSME) is the basement of automatic tea picking technique, determines whether the shoot can be picked. Unfortunately, the ambiguous information among single labels and uncontrollable imaging condition lead to a low FTSME accuracy. A novel Fresh Tea Shoot Maturity Estimating method via multispectral imaging and Deep Label Distribution Learning (FTSME-DLDL) is proposed to overcome these issues. The input is 25-band images, and the output is the corresponding tea shoot maturity label distribution. We utilize the multiple VGG-16 and auto-encoding network to obtain the multispectral features, and learn the label distribution by minimizing the Kullback-Leibler divergence using deep convolutional neural networks. The experimental results show that the proposed method has a better performance on FTSME than the state-of-the-art methods.
更多查看译文
关键词
fresh tea shoot maturity estimation, deep label distribution learning, Kullback-Leibler loss function, label ambiguity
AI 理解论文
溯源树
样例
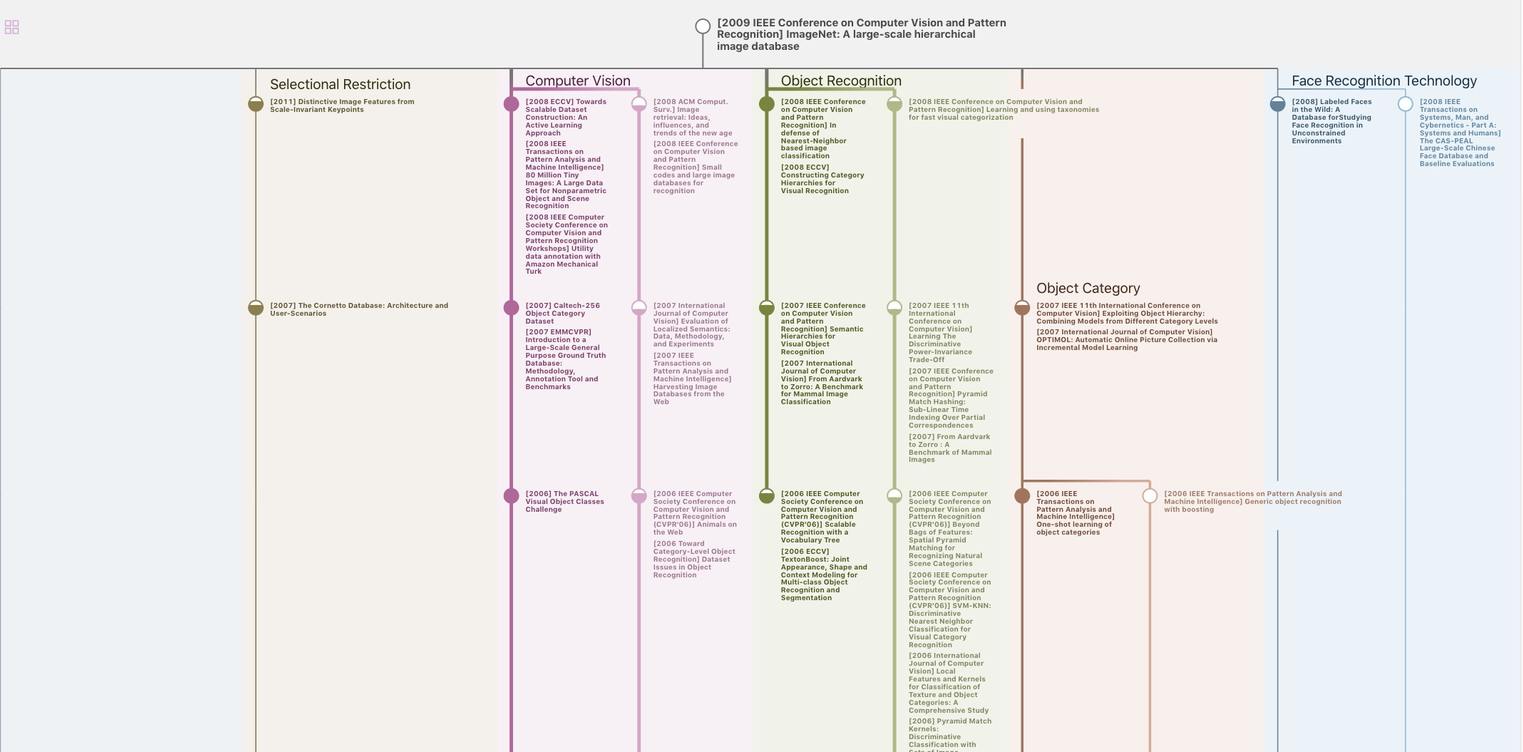
生成溯源树,研究论文发展脉络
Chat Paper
正在生成论文摘要