Attribute-based Granular Evaluation for Performance of Machine Learning Models
2020 IEEE International Conference On Artificial Intelligence Testing (AITest)(2020)
摘要
There is an increasing demand for quality assurance of machine learning (ML) models as more and more ML applications are investigated in various domains. This means that we need to explicitly take account of the requirements and environmental assumptions for quality evaluation and improvement of ML models. The traditional approach has been performance evaluation, typically in accuracy, for the target dataset. However, this approach only focuses on the global accuracy over the whole dataset and obfuscates performance for individual specific aspects in the requirements and environmental assumptions. We then lack insights necessary to deal with high-priority requirements and to detect risks or weaknesses in specific situations. In response to this problem, we investigate a testing method based on attributes that capture aspects in the requirements and environmental assumptions. We divide the input space into explicit and explainable sub-spaces, which allows the divide-and-conquer style in a granular manner as we have done for traditional software testing. We demonstrate our method with simple attributes for CIFAR-10 and BDD100K datasets.
更多查看译文
关键词
machine learning,testing,image classification,data design,requirements-based testing
AI 理解论文
溯源树
样例
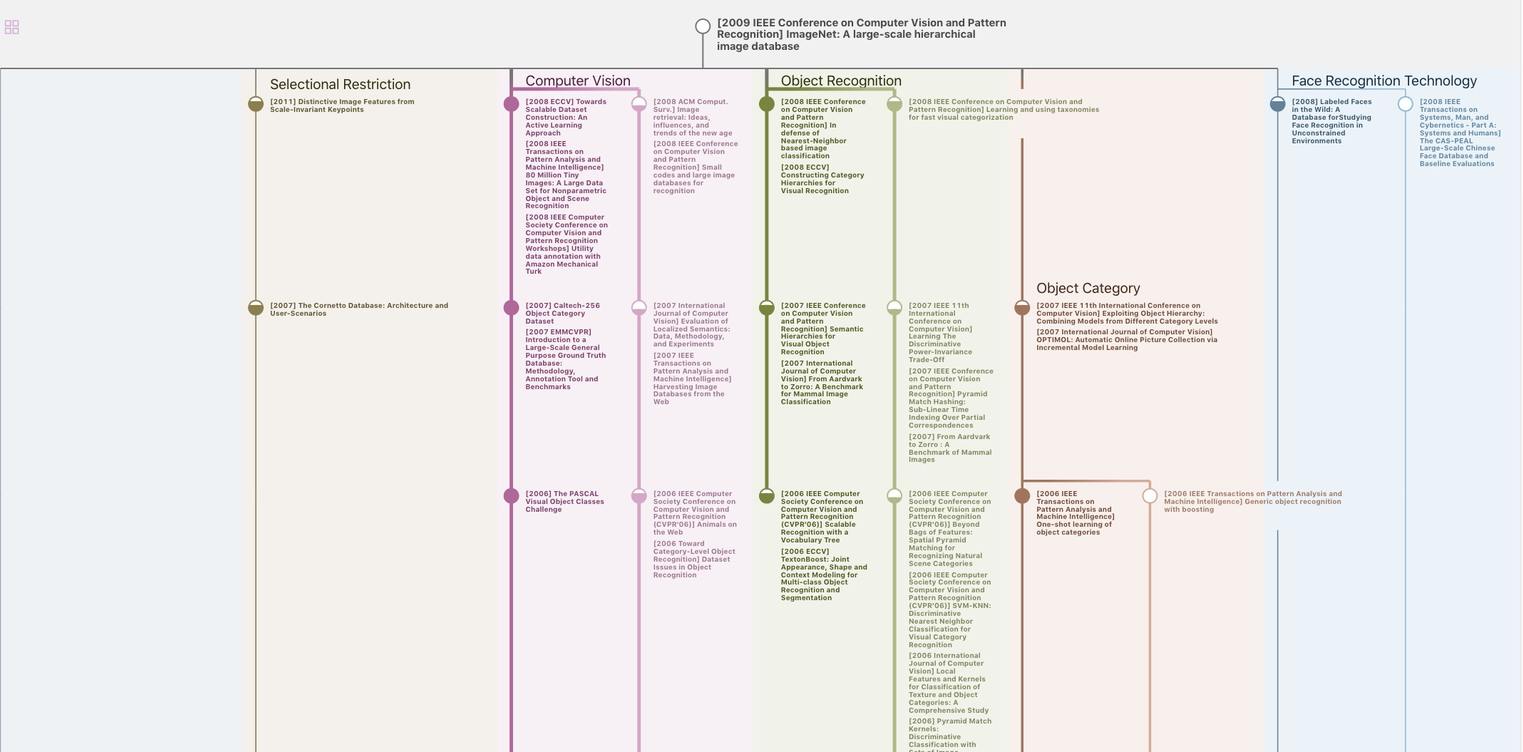
生成溯源树,研究论文发展脉络
Chat Paper
正在生成论文摘要