Time-Aware Attentive Neural Network for News Recommendation with Long- and Short-Term User Representation.
KSEM (2)(2020)
摘要
News recommendation is very critical to help users quickly find news satisfying their preferences. Modeling user interests with accurate user representations is a challenging task in news recommendation. Existing methods usually utilize recurrent neural networks to capture the short-term user interests, and have achieved promising performance. However, existing methods ignore the user interest drifts caused by time interval in the short session. Thus they always assume the short-term user interests are stable, which might lead to suboptimal performance. To address this issue, we propose the novel model named Time-aware Attentive Neural Network with Long-term and Short-term User Representation (TANN). Specifically, to reduce the influence of interest drifts, we propose the Time-aware Self-Attention (T-SA) which considers the time interval information about user browsing history. We learn the short-term user representations from their recently browsing news through the T-SA. In addition, we learn more informative news representations from the historical readers and the contents of news articles. Moreover, we adopt the latent factor model to build the long-term user representations from the entire browsing history. We combine the short-term and long-term user representations to capture more accurate user interests. Extensive experiments on two public datasets show that our model outperforms several state-of-the-art methods.
更多查看译文
关键词
news recommendation,neural network,time-aware,short-term
AI 理解论文
溯源树
样例
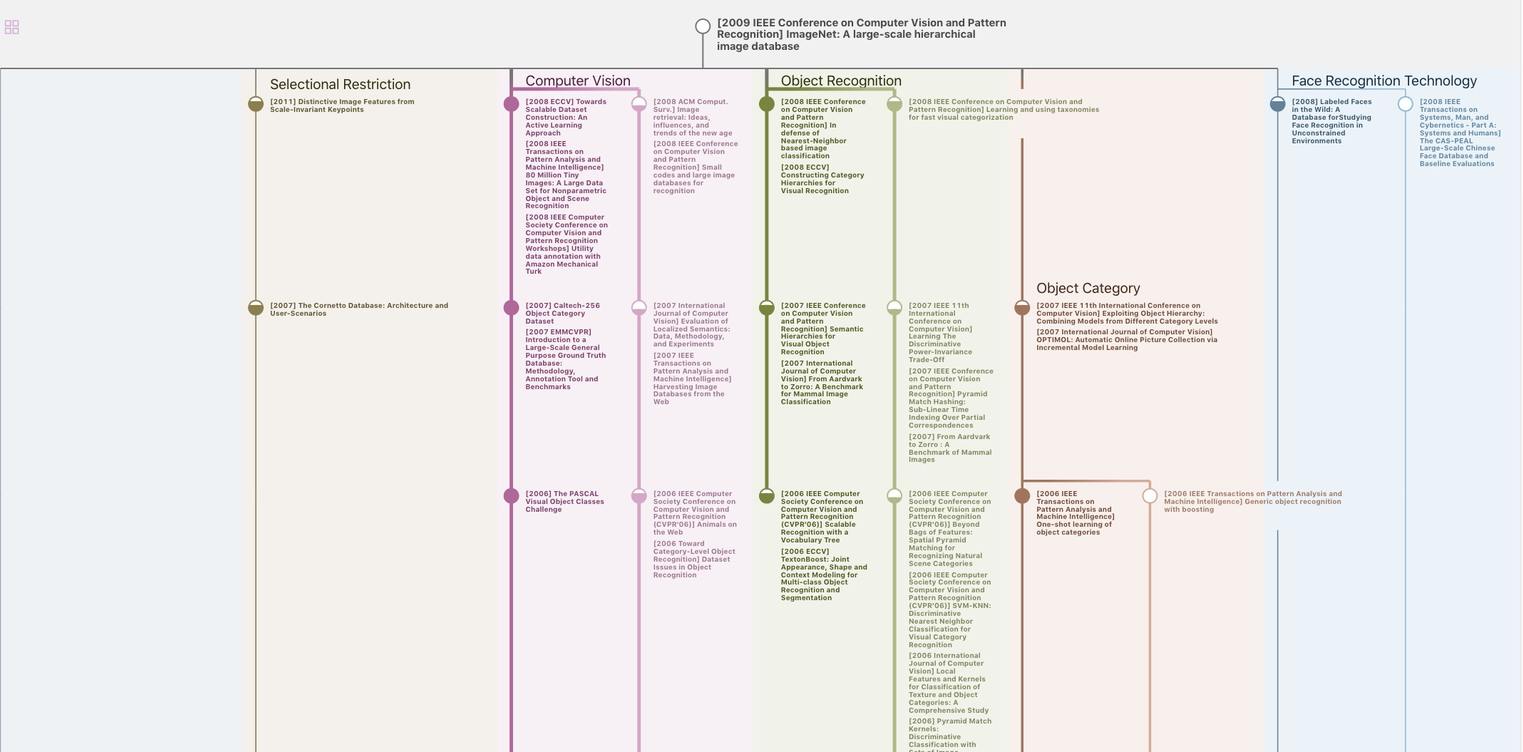
生成溯源树,研究论文发展脉络
Chat Paper
正在生成论文摘要