Glyph: Efficient ML-Based Detection of Heap Spraying Attacks
IEEE Transactions on Information Forensics and Security(2021)
摘要
Heap spraying is probably the most simple and effective memory corruption attack, which fills the memory with malicious payloads and then jumps at a random location in hopes of starting the attacker's routines. To counter this threat, GRAFFITI has been recently proposed as the first OS-agnostic framework for monitoring memory allocations of arbitrary applications at runtime; however, the main contributions of GRAFFITI are on the monitoring system, and its detection engine only considers simple heuristics which are tailored to certain attack vectors and are easily evaded. In this article, we aim to overcome this limitation and propose GLYPH as the first ML-based heap spraying detection system, which is designed to be effective, efficient, and resilient to evasive attackers. GLYPH relies on the information monitored by GRAFFITI, and we investigate the effectiveness of different feature spaces based on information entropy and memory n-grams, and discuss the several engineering challenges we have faced to make GLYPH efficient with an overhead compatible with that of GRAFFITI. To evaluate GLYPH, we build a representative dataset with several variants of heap spraying attacks, and assess GLYPH's resilience against evasive attackers through selective hold-out experiments. Results show that GLYPH achieves high accuracy in detecting spraying and is able to generalize well, outperforming the state-of-the-art approach for heap spraying detection, NOZZLE. Finally, we thoroughly discuss the trade-offs between detection performance and runtime overhead of GLYPH's different configurations.
更多查看译文
关键词
Heap spraying,memory exploitation,machine learning,memory monitoring,detection
AI 理解论文
溯源树
样例
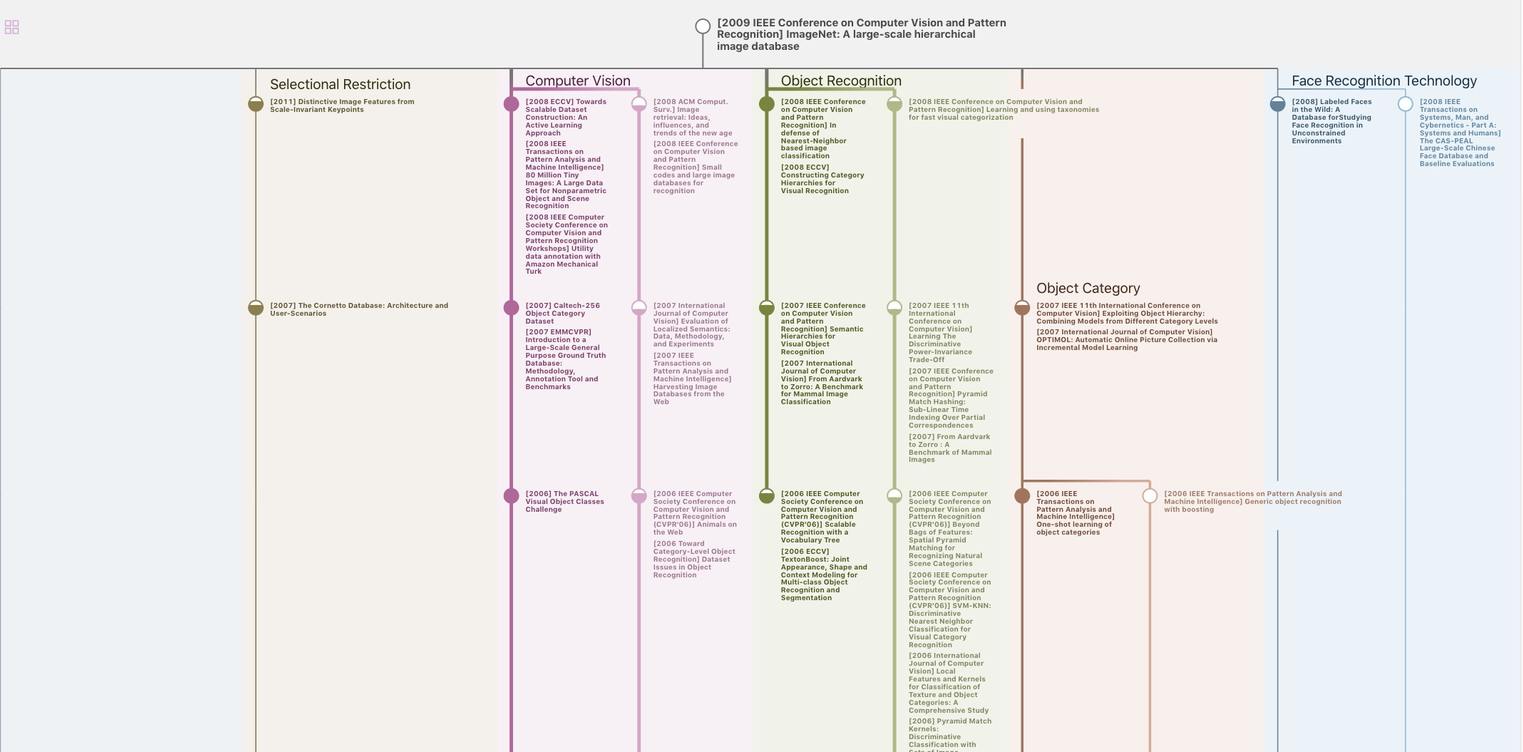
生成溯源树,研究论文发展脉络
Chat Paper
正在生成论文摘要