Skipping Non-essential Instructions Makes Data-Dependence Profiling Faster
Euro-Par(2020)
摘要
Data-dependence profiling is a dynamic program-analysis technique to discover potential parallelism in sequential programs. Unlike purely static analysis, which may overestimate the number of dependences because it does not know many pointers values and array indices at compile time, profiling has the advantage of recording data dependences that actually occur at runtime. But it has the disadvantage of significantly slowing down program execution, often by a factor of 100. In our earlier work, we lowered the overhead of data-dependence profiling by excluding polyhedral loops, which can be handled statically using certain compilers. However, neither does every program contain polyhedral loops, nor are statically identifiable dependences restricted to such loops. In this paper, we introduce an orthogonal approach, focusing on data dependences between accesses to scalar variables - across the entire program, inside and outside loops. We first analyze the program statically and identify memory-access instructions that create data dependences that would appear in any execution of these instructions. Then, we exclude these instructions from instrumentation, allowing the profiler to skip them at runtime and avoid the associated overhead. We evaluate our approach with 49 benchmarks from three benchmark suites. We improved the profiling time of all programs by at least 38%, with a median reduction of 61% across all the benchmarks.
更多查看译文
关键词
Data dependences, Parallelism discovery, Profiling
AI 理解论文
溯源树
样例
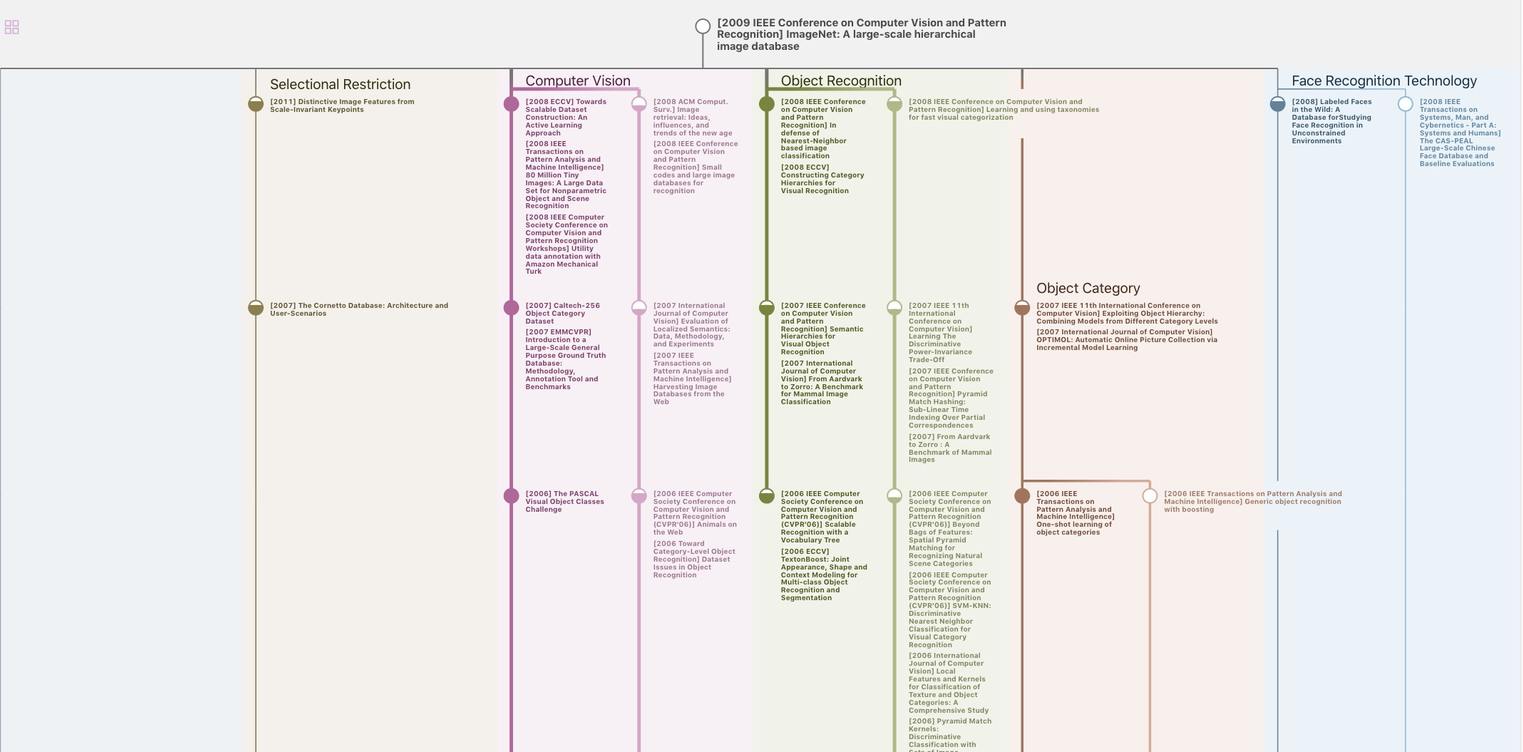
生成溯源树,研究论文发展脉络
Chat Paper
正在生成论文摘要